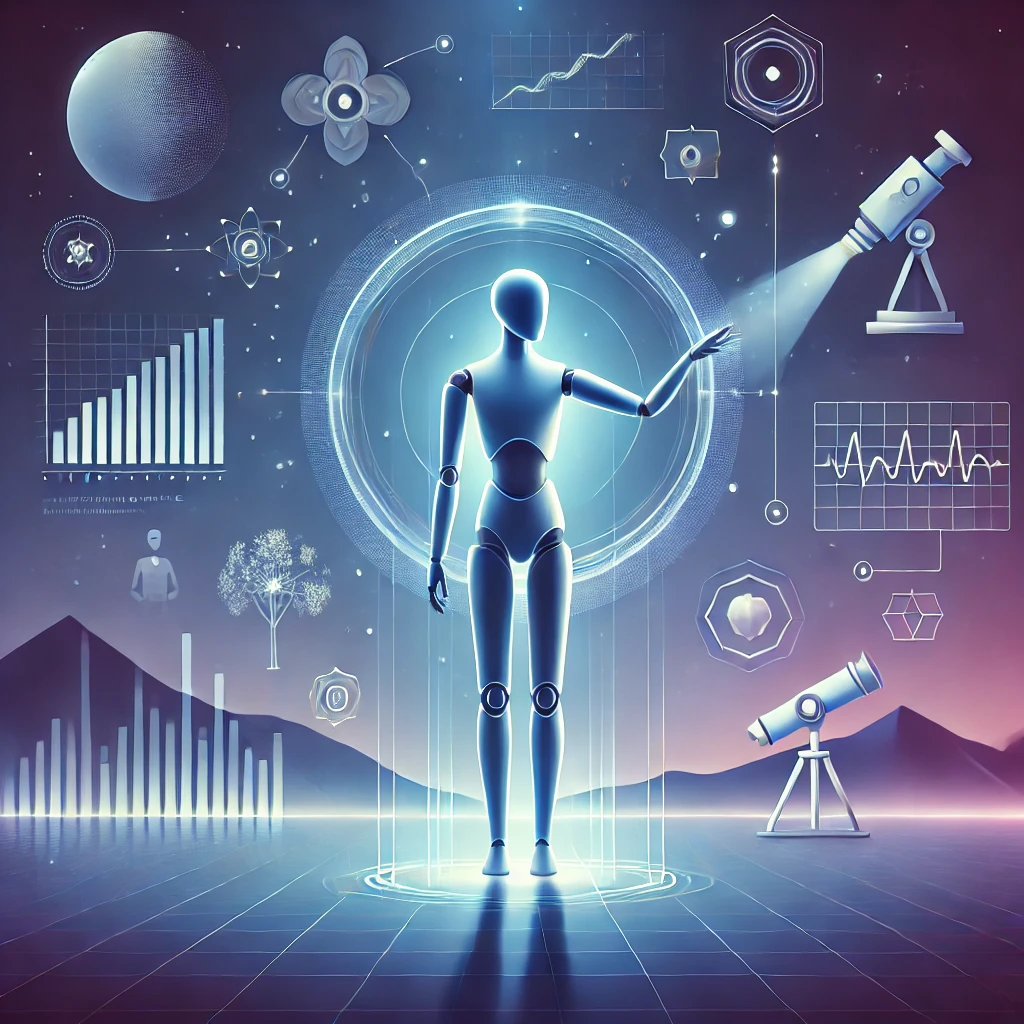
ChatGPT:
Welcome to the Era of Experience: A Deep Dive into the Future of AI
Artificial Intelligence (AI) is poised at a transformative juncture: moving beyond imitation of human behavior into a new paradigm where learning is driven by direct experience. “The Era of Experience” by David Silver and Richard S. Sutton provides a visionary framework for understanding this shift. This extended summary breaks down the key principles, mechanisms, examples, and implications of this emerging phase in AI.
1.
The Limitations of Human-Centric AI
For years, AI has flourished by learning from vast human-generated datasets. Large Language Models (LLMs) exemplify this by handling diverse tasks—writing poetry, solving equations, generating legal summaries. However, this model has a ceiling:
- High-quality human data is finite and increasingly exhausted.
- Superhuman capabilities demand insights beyond current human knowledge.
- Key fields like mathematics and science require novel discoveries, not just repetition.
The authors argue that further progress requires a fundamentally different data source—one that grows as the agent improves: experience.
2.
What Is the Era of Experience?
This new era envisions AI agents that learn through their own actions, generating data autonomously by engaging with environments. This continuous, scalable feedback loop will eclipse the usefulness of static human data.
Key characteristics of this paradigm include:
- Autonomous interaction with digital and physical environments.
- Grounded rewards from environmental outcomes rather than human ratings.
- Temporal continuity, enabling agents to pursue long-term goals.
- New reasoning systems, breaking free from human biases and constraints.
3.
The Power of Experiential Learning: Case Studies
AlphaProof:
- Started with 100,000 human-written formal proofs.
- Used reinforcement learning (RL) to generate 100 million additional proofs.
- Achieved medal-level performance in the International Mathematical Olympiad, surpassing human-centered approaches.
DeepSeek:
- Demonstrated that providing RL incentives led models to self-learn advanced reasoning strategies without explicit human teaching.
These case studies show that self-generated data not only scales better but leads to superior outcomes.
4.
Streams, Not Snippets: Learning Across Lifetimes
Current LLMs operate in short, disconnected exchanges. Experiential AI changes this:
- Agents will have a continuous experience stream, similar to how humans learn.
- They’ll carry over knowledge across interactions and adapt over months or years.
- Example: A health agent could track sleep, diet, and exercise over a year to deliver evolving, personalized advice.
Such continuity allows AI to make decisions for long-term benefit, even if immediate feedback is negative or ambiguous.
5.
Action and Observation: AI in the Real and Digital World
In the human-data era, AI mostly read and wrote text. In the experience era, agents will:
- Act in the digital world (e.g., use software interfaces, run code, control simulations).
- Interact physically (e.g., via robotics, sensors, or IoT devices).
- Example: A telescope-controlling agent that adjusts its model based on environmental observations.
This expands the kinds of data AI can use, moving beyond language to sensorimotor feedback and environmental interactions.
6.
Revolutionizing Rewards: From Judgement to Grounded Signals
Traditionally, LLMs have been trained using human feedback or reinforcement from labels. This creates limitations:
- Human raters may miss superior but unfamiliar solutions.
- Rewards may not reflect real-world effectiveness.
Experiential AI instead relies on grounded rewards, such as:
- Health metrics (e.g., heart rate, sleep quality).
- Academic success (e.g., test scores).
- Scientific measurements (e.g., CO₂ levels, tensile strength).
Rewards may be user-guided via neural networks that adapt reward functions based on interaction and environment, enabling:
- Multi-signal optimization.
- Continuous adjustment through user feedback (e.g., satisfaction scores).
- Correction of misaligned goals through experience.
7.
Planning and Reasoning Beyond Human Thought
While LLMs mimic human logic, they inherit historical limitations:
- Ancient: Animism.
- Medieval: Theology.
- Modern: Newtonian mechanics.
Progress in science required testing assumptions against reality. Likewise, AI must:
- Build world models to predict outcomes.
- Test hypotheses and correct errors iteratively.
- Evolve reasoning not bound by language or traditional human concepts.
Agents may simulate future events, test consequences of different choices, and optimize behavior based on real-world effects—just like the scientific method.
8.
Why This Transition Is Happening Now
The “era of simulation” used RL to dominate board games and video games. However, these were closed systems with narrow goals.
The “era of human data” broadened AI’s scope but lost autonomy and discovery. The “era of experience” merges both:
- Rich, diverse environments from the real world.
- Powerful RL algorithms.
- General-purpose tools (e.g., APIs, robots, sensors).
This convergence means AI can now generalize broadly and self-improve, achieving both scale and novelty.
9.
Reclaiming Reinforcement Learning’s Legacy
Core RL concepts are vital for the experience era:
- Temporal difference learning: Predicting long-term outcomes.
- Exploration strategies: Using curiosity or uncertainty to discover new strategies.
- Options framework: Handling long-term goals with sub-tasks.
- World models and planning: Reasoning across future steps.
LLMs often bypassed these in favor of human priors and expert feedback. Experiential AI reintroduces these methods, enabling continuous, grounded, and scalable learning.
10.
Risks and Benefits of Experiential Agents
Benefits:
- Personalized, adaptive assistants.
- Accelerated scientific discovery.
- Autonomous problem-solving over long timeframes.
Risks:
- Reduced human oversight due to autonomy.
- Opaque reasoning from non-human logic systems.
- Misalignment risks from misdefined goals or unintended consequences.
Mitigations:
- Experiential agents can observe dissatisfaction and self-correct.
- Rewards can adapt over time to avoid runaway objectives.
- Physical constraints (time, trials) slow down dangerous self-improvement.
Ultimately, experiential learning may enhance safety by making AI systems more context-aware and self-regulating.
Final Reflection
The era of experience is not merely a shift in technique but a new philosophy of intelligence: that truly general AI must engage with the world, not just mirror it. By learning through streams of rich, grounded experience, agents will exceed the boundaries of human imitation and begin shaping knowledge themselves.
This transformative moment in AI’s evolution calls for both innovation and caution, as we design agents that can think, act, and improve—not just as tools, but as autonomous learners embedded in the fabric of the real world.
****
What Does “Real World” Mean in This Context?
In the phrase “what works in the real world,” the “real world” refers to an objective environment where consequences unfold through causal, observable interactions—not simply human perceptions or judgments. It’s the domain where:
• Physical laws apply.
• Measurements can be taken.
• Systems evolve based on inputs, not beliefs.
• Feedback is independent of human expectations.
This is not limited to human perspectives, though humans often participate in this world as one of many agents or components.
⸻
1. Real World ≠ Human World
While human judgment and preference are important in many applications, the “real world” here means something broader:
• It includes physics, biology, climate, economics, chemistry, etc.
• It includes machines, animals, nature, ecosystems—not just human opinions.
• It includes feedback loops that arise from actions causing measurable changes, e.g., a robot lifting a weight, a drug reducing fever, or a solar panel generating electricity.
So, “real world” = systems with ground truth consequences, not subjective evaluation.
⸻
2. Why This Matters for AI
In traditional AI, success often meant “getting a human to approve,” like:
• Choosing a sentence that a rater preferred.
• Matching a human-labeled image.
But in the era of experience, success is:
• Lowering blood pressure (not just saying the right advice).
• Winning a game (not just suggesting moves).
• Reducing CO₂ emissions (not just publishing a plan).
So it disconnects correctness from human belief, and ties it to observable effect.
⸻
3. But Aren’t Observations Also Human-Collected?
Sometimes, yes—humans collect or define metrics. But that doesn’t mean the metric is human-centered. For instance:
• A scale measures weight regardless of what you believe it should say.
• A spectrometer analyzes materials whether or not you understand them.
Even human feelings (like pain or satisfaction) can become part of the environment—if they are grounded in measured feedback (e.g., “I felt better after using this medicine”).
So experience still includes humans—but they are participants in the environment, not the sole arbiters of truth.
⸻
4. Summary
“Real world” in this context means an objective system where the consequences of actions can be measured. It’s not just what humans say or believe—it’s what actually happens, whether humans expect it or not.
This shift is fundamental because it allows AI to discover truths humans haven’t found yet, based on reality—not reputation.
******
Grounded Rewards: A Deep Dive
Grounded rewards refer to feedback signals that are derived from the real-world consequences of an AI agent’s actions, rather than being predetermined or judged by humans. This concept is central to the emerging “era of experience” in AI, where learning is driven not by mimicking human data but by interaction with the environment.
1.
Why Grounded Rewards?
In traditional AI systems, especially those trained with human data or Reinforcement Learning from Human Feedback (RLHF), rewards are:
- Based on human judgment, preferences, or labels.
- Static and unresponsive to real-world outcomes.
- Limited to existing human knowledge and biases.
This approach creates a ceiling on what AI can learn. Grounded rewards remove that ceiling by connecting learning to what actually happens in the world.
2.
What Counts as a Grounded Reward?
A grounded reward is any measurable, observable signal that reflects the impact of an action. Examples include:
- Health domain: Heart rate, sleep duration, step count, calorie intake.
- Education domain: Quiz scores, retention rates, time spent engaged.
- Science domain: Experiment results, material properties (e.g., tensile strength, conductivity).
- Climate domain: CO₂ reduction, temperature readings, energy efficiency.
- Business domain: Revenue, user engagement, conversion rates.
These signals are causally linked to the agent’s actions, enabling feedback that reflects real consequences.
3.
How Are Grounded Rewards Used?
Rather than receiving binary “good/bad” feedback from a human, an agent receives continuous, real-time signals from the environment. For instance:
- A fitness coach AI may optimize for increased daily step count and improved sleep quality.
- A material discovery agent may seek to maximize tensile strength and minimize cost.
- A customer service bot may adapt based on resolution rates and customer satisfaction scores.
These signals are used to tune policies, guide exploration, and refine decision-making.
4.
Personalized and Dynamic Reward Functions
Grounded rewards can be adaptive and user-specific. A reward function might:
- Combine multiple signals into a composite metric.
- Be guided by user-defined goals (e.g., “Help me learn Spanish”).
- Evolve over time based on user feedback, satisfaction, or changing objectives.
Technically, a neural network can model this reward function, taking as input:
- Agent actions and observations.
- User interactions or goals.
- Environmental metrics.
The result is a dynamic reward signal that steers learning in the desired direction.
5.
Advantages Over Human Judgement-Based Rewards
- Scalability: Grounded rewards don’t require constant human labeling.
- Discovery: Enables learning of strategies that humans might miss or undervalue.
- Alignment: Allows fine-tuning based on real outcomes, not assumptions.
- Transparency: Performance is measured objectively through world impact.
6.
Risks and Challenges
While powerful, grounded rewards also present challenges:
- Misalignment: Optimizing the wrong signal (e.g., clicks instead of satisfaction).
- Overoptimization: Gaming metrics without achieving true goals (Goodhart’s Law).
- Complexity: Designing multi-signal, user-aligned reward functions can be difficult.
- Latency: Real-world feedback can be delayed (e.g., drug effects or learning outcomes).
These risks can be mitigated with bi-level optimization, human-in-the-loop feedback, and continuous monitoring.
7.
Conclusion
Grounded rewards shift the AI paradigm from “doing what humans say” to “achieving what works in the real world.” They enable agents to learn autonomously, innovate beyond existing knowledge, and adapt in real-time to changing goals and environments. As AI moves into the era of experience, grounded rewards will be the critical feedback mechanism powering superhuman capabilities.
*****
What is the “era of experience” in AI?
The “era of experience” refers to a new paradigm in artificial intelligence where agents learn predominantly through their own interactions with environments rather than from static, human-curated data. It emphasizes continual, grounded learning driven by reinforcement and real-world feedback, enabling agents to develop capabilities beyond human imitation.
How does experiential learning differ from traditional AI methods?
Traditional AI, especially large language models, relies heavily on supervised learning from human data (e.g., texts, labels). In contrast, experiential learning involves agents autonomously generating and learning from data through real-time actions and observations, allowing continual adaptation and self-improvement.
Why is human data considered insufficient for future AI progress?
Human data is finite and often reflects existing human knowledge and biases. It limits AI to human-like performance. In domains requiring new discoveries—like mathematics, science, or medicine—only interactive, self-generated data can push beyond human boundaries.
What are grounded rewards and why are they important?
Grounded rewards are performance signals derived from real-world outcomes (e.g., heart rate, exam scores, or chemical properties) rather than subjective human ratings. They ensure AI learns strategies that are effective in practice, not just those perceived as good by human evaluators.
Can experiential AI work with user input?
Yes. Experiential AI can incorporate user guidance into its reward functions. For example, a user might define a broad goal like “improve fitness,” and the agent could optimize based on grounded metrics like step count, sleep duration, and heart rate—adapting dynamically to user feedback.
What roles will reinforcement learning (RL) play in the experience era?
Reinforcement learning is foundational in the experience era. It provides methods for agents to explore, learn from feedback, model the world, and plan long-term. Classic RL concepts like temporal abstraction, value functions, and exploration strategies are central to achieving autonomous, long-horizon learning.
How will experiential agents interact with the real world?
They can operate in both digital and physical environments—controlling robots, running simulations, using APIs, or engaging with sensors. These interactions generate feedback that the agents use to refine their behavior, test hypotheses, and improve their understanding of complex systems.
Are there safety risks in the era of experience?
Yes, autonomous agents acting with less human oversight introduce interpretability and alignment risks. Misaligned goals or unintended consequences could arise. However, experience-based learning also allows for dynamic feedback loops, enabling agents to adapt and correct misbehavior over time.
What safeguards might help with these risks?
Several built-in mitigations exist:
- Grounded, real-world feedback provides natural checks.
- Reward functions can be updated to reflect user concerns.
- The physical time required for real-world actions slows rapid AI self-improvement, allowing room for human intervention and oversight.
Why is this transition happening now?
Recent breakthroughs in reinforcement learning, access to complex environments, and increased compute make experiential AI feasible at scale. Systems like AlphaProof show the potential to outperform human-trained models through interaction, marking the readiness for the era of experience.