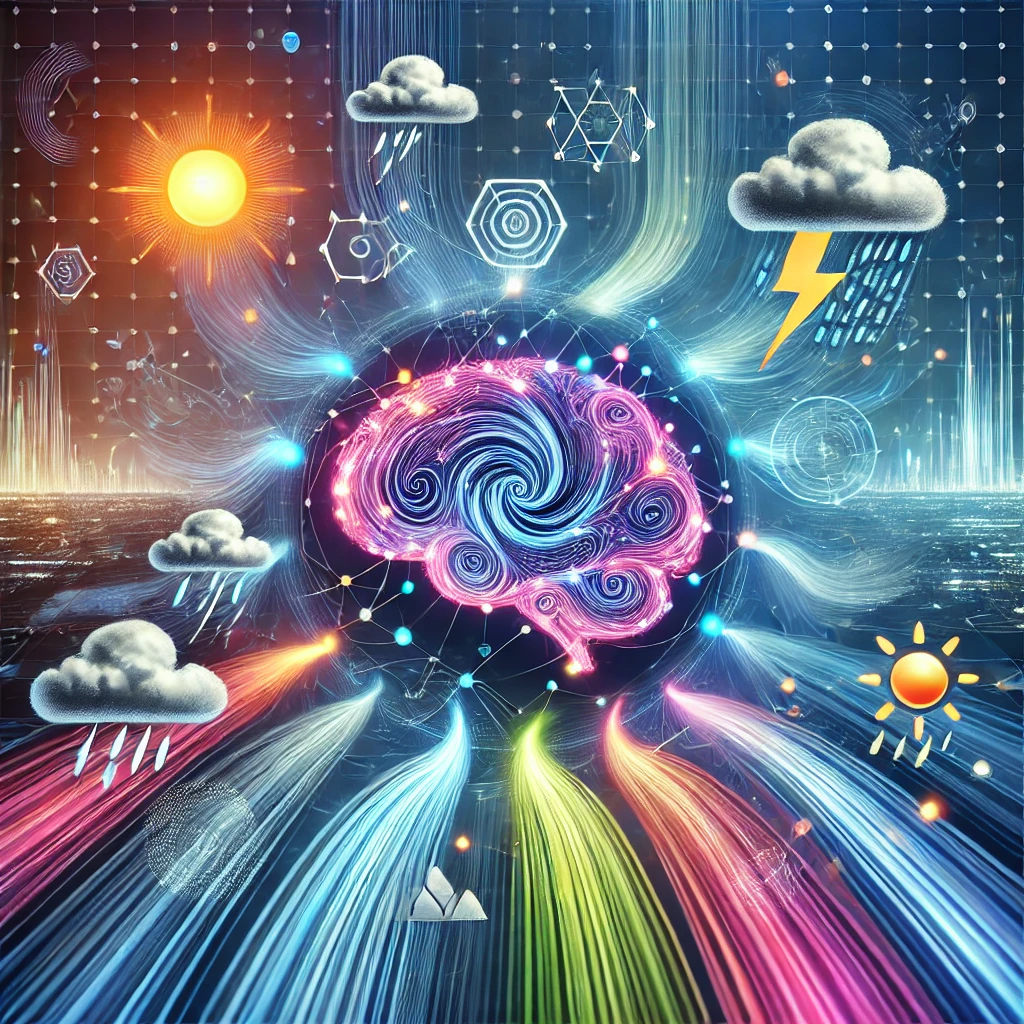
ChatGPT:🚀 The Rise of AI in Weather Forecasting
🌩️ Introduction
Artificial Intelligence (AI) is transforming the field of meteorology by offering more rapid and accurate weather predictions than traditional methods. Historically, weather forecasting relied heavily on supercomputers processing vast amounts of real-time data to simulate atmospheric conditions. However, the advent of AI, particularly machine learning techniques, has introduced a new paradigm in forecasting, enabling predictions to be made with unprecedented speed and precision.
🌀 Case Study: Hurricane Beryl
In early July, as Hurricane Beryl approached the Caribbean, traditional and AI-based forecasting methods offered contrasting predictions. The European Center for Medium-Range Weather Forecasts (ECMWF), utilizing supercomputers and comprehensive global data, predicted Beryl’s landfall in Mexico. In contrast, an AI system called GraphCast, developed by DeepMind, anticipated the hurricane making landfall in Texas. Ultimately, the hurricane struck Texas, confirming the accuracy of the AI model. This event highlighted the potential of AI to enhance predictive accuracy, particularly in critical scenarios where human lives and property are at risk.
📊 The Role of GraphCast
GraphCast exemplifies the power of AI in meteorology. It leverages a machine learning approach, where the model is trained on decades of historical weather data rather than relying solely on real-time inputs. This method allows the system to learn patterns and relationships within the data, enabling it to make predictions rapidly—sometimes in seconds. Unlike traditional models that require extensive computational resources, GraphCast can run on relatively modest hardware, such as desktop computers, making advanced forecasting accessible to a broader audience.
🎖️ Recognition and Achievements
The innovative nature of GraphCast has not gone unnoticed. The team behind it recently received Britain’s top engineering prize from the Royal Academy of Engineering. The recognition underscores the model’s significant contributions to the field, as it consistently outperforms traditional models in predictive accuracy. In particular, GraphCast has shown remarkable proficiency in forecasting the paths of hurricanes and other extreme weather events, which are critical for public safety and preparedness.
🔬 Scientific and Practical Implications
The integration of AI into weather forecasting extends beyond mere prediction. AI models like GraphCast offer meteorologists a powerful tool for scientific discovery. By analyzing vast datasets, AI can identify subtle patterns and anomalies that may indicate new meteorological phenomena or refine our understanding of existing ones. This capability is crucial for advancing the field, particularly in understanding and predicting extreme weather events such as tornadoes, severe thunderstorms, and heatwaves.
🌍 A Broader Adoption and Future Integration
Major meteorological institutions, including ECMWF, have begun incorporating AI models into their forecasting systems. This integration signifies a broader acceptance and validation of AI’s capabilities within the scientific community. AI is not expected to replace traditional methods entirely but rather to complement them. Each approach—traditional supercomputers and AI—has unique strengths. Supercomputers excel at detailed, physics-based simulations, while AI provides rapid, pattern-based predictions.
🤔 The Human Element and Future Directions
Despite the advancements in AI, the role of human meteorologists remains vital. Experts like Jamie Rhome from the National Hurricane Center emphasize the importance of human judgment and situational awareness in interpreting forecasts. While AI provides data and predictions, the nuances of weather forecasting—such as the assessment of storm intensity, potential impacts, and public communication—require expert human input.
The future of weather forecasting likely involves a hybrid approach, leveraging the strengths of both traditional models and AI. This combined method will provide the most comprehensive and reliable forecasts, crucial for decision-making in various sectors, from agriculture to disaster management.
🌟 Conclusion
AI represents a transformative force in weather forecasting, offering unprecedented speed and accuracy. Systems like GraphCast demonstrate the potential to revolutionize how we predict and prepare for weather events. However, the most effective approach will integrate AI with traditional methods and expert human oversight, ensuring comprehensive and reliable forecasting. As the technology continues to evolve, its role in meteorology will undoubtedly expand, offering new tools and insights for understanding our planet’s complex and dynamic atmosphere.
Key Areas of Expansion
The Role of Big Tech in Meteorology: The influence of major tech companies like Google (via DeepMind) in shaping the future of weather forecasting and climate science.
Detailed Analysis of AI Models in Weather Forecasting: Exploration of different AI models, their methodologies, and comparative performance metrics.
Case Studies on AI Successes and Limitations: In-depth examination of specific weather events where AI forecasting was notably accurate or faced challenges.
The Science Behind Machine Learning in Meteorology: An explanation of how machine learning models are trained on historical weather data and the kinds of patterns they can detect.
The Role of Supercomputers vs. AI Models: A discussion on the unique advantages and limitations of traditional supercomputer-based models and AI-based models.
Human Expertise in AI-Driven Forecasting: The continued importance of meteorologists in interpreting AI data and making critical decisions based on forecasts.
Future Directions in AI and Meteorology: Speculations on upcoming advancements in AI technologies and their potential impact on weather prediction and climate science.
Global Impact of AI in Weather Forecasting: How different countries and regions are adopting AI in their meteorological practices and the global implications.
Ethical and Practical Considerations: Addressing concerns around data privacy, the reliance on automated systems, and the implications of AI-driven decision-making in meteorology.
Educational and Training Implications: The need for training new meteorologists in AI and data science to leverage these tools effectively.
Q&A on AI in Weather Forecasting
Q: What is GraphCast and how does it differ from traditional weather forecasting methods?
A: GraphCast is an AI-based weather forecasting system developed by DeepMind. Unlike traditional methods that rely on real-time data and extensive computation using supercomputers, GraphCast uses machine learning to analyze historical weather data and identify patterns. This allows it to generate forecasts quickly and accurately, even on less powerful hardware like desktops.
Q: How accurate is AI compared to traditional forecasting methods?
A: AI models, like GraphCast, have shown remarkable accuracy in predicting weather events. In tests, GraphCast outperformed the European Center for Medium-Range Weather Forecasts’ (ECMWF) traditional models over 90% of the time, especially in predicting hurricane paths.
Q: Why is AI in weather forecasting considered a “revolutionary” advancement?
A: AI is revolutionary in weather forecasting because it significantly speeds up the prediction process, making it possible to generate forecasts in minutes instead of hours. It also democratizes access to advanced forecasting, as these AI models can run on relatively simple hardware compared to the room-sized supercomputers traditionally used.
Q: How did AI outperform traditional models in the case of Hurricane Beryl?
A: During Hurricane Beryl, traditional models predicted a different landfall location compared to the AI forecast provided by GraphCast, which accurately predicted the storm would hit Texas. This demonstrated AI’s capability to identify critical patterns and predict outcomes more precisely.
Q: What are the potential limitations of using AI for weather forecasting?
A: While AI can excel at predicting certain aspects like storm paths, it may struggle with other factors such as precipitation, wind speeds, and storm surge predictions. AI models still require significant training data and might not fully account for all complex atmospheric dynamics.
Q: How do meteorologists view the integration of AI in forecasting?
A: Meteorologists see AI as a valuable tool that can complement traditional methods. While AI can offer quick and accurate forecasts, human expertise is still essential for interpreting the data, especially for complex weather phenomena and public communication.
Q: What are some of the broader implications of using AI in weather forecasting?
A: The use of AI in weather forecasting could lead to more accurate and timely predictions, potentially saving lives by improving emergency preparedness. It also opens up new avenues for research in meteorology, helping scientists discover and understand new weather phenomena.
Q: How accessible is AI weather forecasting technology compared to traditional methods?
A: AI technology for weather forecasting is more accessible because it can operate on standard desktop or laptop computers, unlike traditional methods that require expensive and large-scale supercomputers. This makes advanced forecasting technology available to a wider range of institutions and individuals.
Q: Will AI replace traditional weather forecasting methods?
A: AI is not expected to completely replace traditional methods but rather complement them. The combination of traditional physics-based models and AI’s pattern recognition capabilities provides a more robust approach to forecasting.
Q: What is the future outlook for AI in meteorology?
A: The future of AI in meteorology looks promising, with continued advancements expected to improve the accuracy and scope of weather predictions. This includes better forecasting of extreme weather events and deeper insights into climate patterns, potentially transforming the field of meteorology.
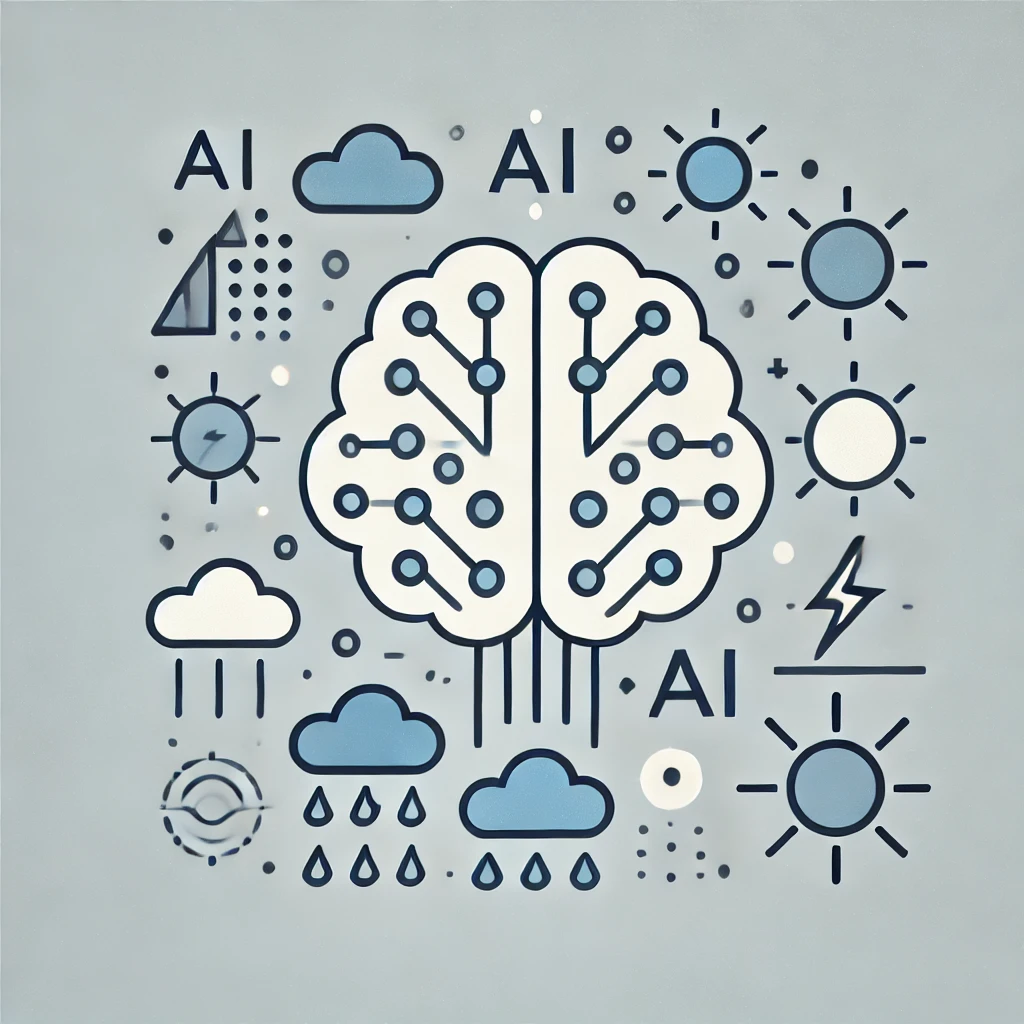