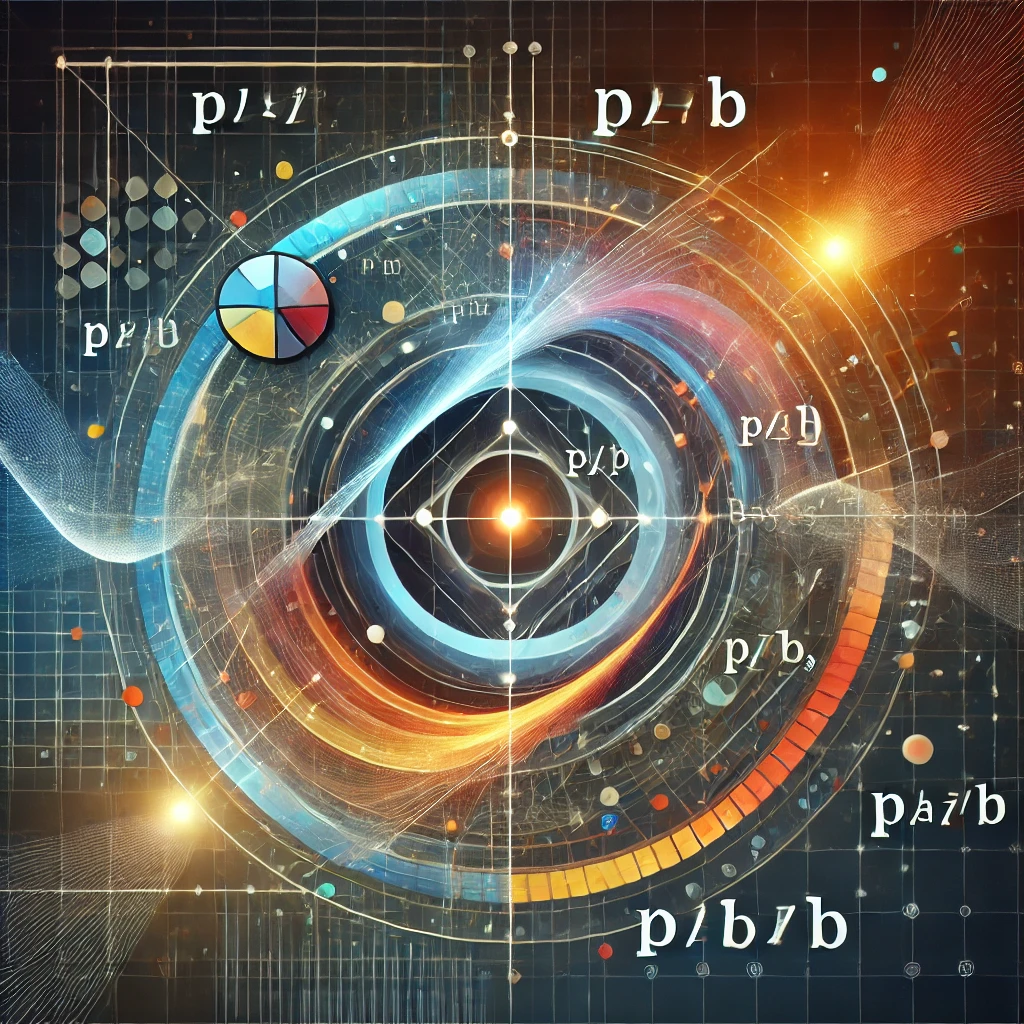
ChatGPT:
Bayes’ Theorem: Definition and Explanation
Bayes’ Theorem is a fundamental concept in probability theory and statistics that provides a mathematical framework for updating probabilities based on new evidence. It relates conditional probabilities and is expressed as:
Where:
• : Probability of event given that event has occurred.
• : Probability of event given that event has occurred.
• : Probability of event occurring.
• : Probability of event occurring.
The theorem is often used in various fields, such as medical diagnosis, machine learning, and spam detection, to update prior knowledge with new data.
Origin of Bayes’ Theorem
1. Thomas Bayes (1701–1761):
• Bayes’ Theorem is named after Thomas Bayes, an English Presbyterian minister and mathematician.
• Bayes developed the foundational ideas of his theorem in his posthumously published paper, “An Essay Towards Solving a Problem in the Doctrine of Chances” (1763), edited by Richard Price.
• In the essay, Bayes tackled the problem of inverse probability—how to update probabilities with new evidence.
2. Richard Price’s Role:
• Richard Price, Bayes’ friend, polished and presented Bayes’ work to the Royal Society.
• Price emphasized the practical implications of the theorem in decision-making under uncertainty.
Development and Popularization
1. Pierre-Simon Laplace:
• Independently rediscovered Bayes’ ideas and generalized them.
• Laplace formulated the Bayesian interpretation of probability as a measure of belief and incorporated the theorem into his Theory of Probability (1812).
2. Neglect and Resurgence:
• For much of the 19th and early 20th centuries, the frequentist approach to probability dominated, sidelining Bayesian methods.
• Bayesian statistics saw a resurgence in the mid-20th century, driven by advances in computation and practical applications.
3. Modern Bayesian Applications:
• In the 20th century, statisticians like Harold Jeffreys and Leonard Savage developed Bayesian methods for scientific reasoning and decision theory.
• The advent of powerful computing enabled complex Bayesian models, making the theorem a cornerstone of modern machine learning, artificial intelligence, and data science.
Philosophical Significance
Bayes’ Theorem has fueled debates in the philosophy of science about the nature of probability:
• Bayesian View: Probability represents degrees of belief, updated with evidence.
• Frequentist View: Probability is the long-term frequency of an event.
Despite philosophical differences, Bayes’ Theorem is now widely acknowledged as a versatile tool for reasoning under uncertainty.
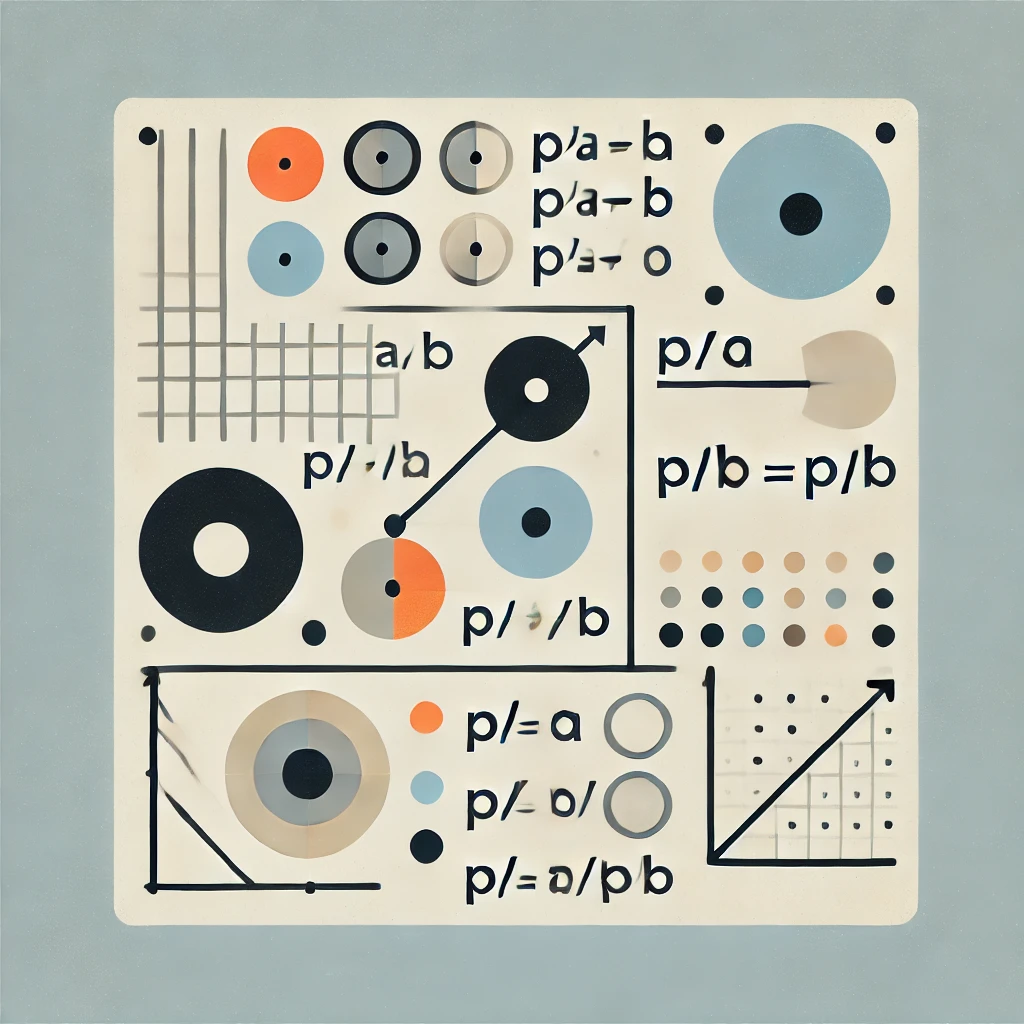
Bayesian Inference: Definition and Explanation
Bayesian inference is a statistical method that uses Bayes’ Theorem to update the probability of a hypothesis as new data or evidence becomes available. It combines prior beliefs (or knowledge) with observed evidence to make decisions or predictions under uncertainty.
The core idea of Bayesian inference is that probabilities are a measure of belief or certainty, and these beliefs are updated when new data is introduced.
Bayesian Inference Formula
The key formula in Bayesian inference is derived from Bayes’ Theorem:
Where:
• : The posterior probability, the probability of the hypothesis given the data .
• : The likelihood, the probability of observing the data given that the hypothesis is true.
• : The prior probability, the initial belief about the hypothesis before observing the data.
• : The evidence, the total probability of the observed data under all possible hypotheses.
Steps in Bayesian Inference
1. Define Prior Probability ():
• Represent your initial belief about the hypothesis based on past knowledge or assumptions.
2. Collect Data ():
• Gather new evidence or observations.
3. Calculate the Likelihood ():
• Determine the probability of observing the data assuming the hypothesis is true.
4. Update Belief with Posterior Probability ():
• Use Bayes’ Theorem to compute the updated probability of the hypothesis given the new evidence.
5. Iterate as New Data Comes In:
• The posterior probability becomes the new prior when additional data is observed.
Applications of Bayesian Inference
1. Medical Diagnosis:
• Estimate the probability of a disease based on symptoms, test results, and prior prevalence rates.
2. Machine Learning:
• Use Bayesian models in algorithms like Naive Bayes or Bayesian Networks for classification and decision-making.
3. Decision-Making:
• Make better-informed choices in fields like finance, marketing, and engineering by updating beliefs with real-time data.
4. Scientific Research:
• Test hypotheses and update conclusions based on experimental results.
5. Risk Assessment:
• Predict and mitigate risks in industries like insurance and security.
Advantages of Bayesian Inference
• Flexibility: It can incorporate prior knowledge into the analysis.
• Dynamic Updating: Beliefs can be continuously refined as new data arrives.
• Interpretability: Provides direct probabilities for hypotheses, making results easier to understand.
Challenges in Bayesian Inference
• Choice of Prior: Selecting an appropriate prior can be subjective and influence results.
• Computational Intensity: For complex models, calculations can be computationally expensive, requiring techniques like Markov Chain Monte Carlo (MCMC).
Key Insight
Bayesian inference is powerful because it combines evidence with prior beliefs in a mathematically rigorous way, making it invaluable for reasoning and decision-making under uncertainty.
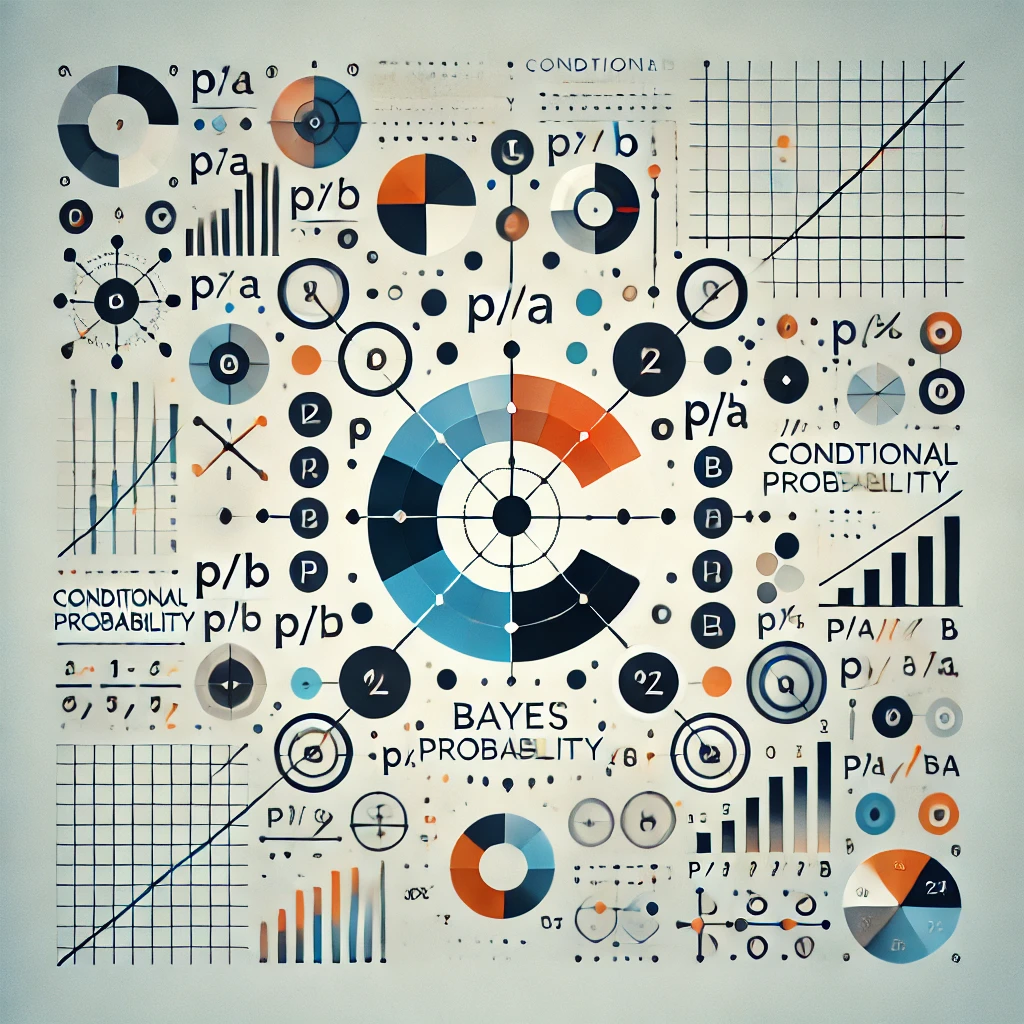
Applying Bayes’ Theorem to optimize the life of aging seniors involves using probabilistic reasoning to make informed decisions in areas like healthcare, lifestyle adjustments, and risk management. Here’s how Bayes’ Theorem can be applied step by step:
1. Personalized Health Risk Assessment
Bayes’ Theorem can help estimate the likelihood of diseases or conditions based on new information, such as test results or lifestyle changes.
Example: Early Detection of Chronic Diseases
• Prior Probability (): The probability of a senior having a disease based on age, family history, or general population statistics.
• Likelihood (): Probability of observing certain symptoms or test results if the disease is present.
• Posterior Probability (): Updated probability of having the disease after test results.
This allows for early interventions tailored to the individual’s needs.
2. Medication Management
Bayes’ Theorem can assess the likelihood of side effects or adverse reactions to medications based on the senior’s medical history and demographics.
Example:
• Prior Probability (): Likelihood of experiencing side effects based on general statistics.
• Likelihood (): Probability of specific side effects given the senior’s current medications.
• Posterior Probability (): Updated risk of side effects for the individual, aiding in safer medication choices.
3. Cognitive Decline Prevention
For conditions like dementia, Bayesian methods can help optimize preventive strategies by integrating genetic, lifestyle, and medical data.
Example: Lifestyle Interventions
• Prior Probability (): Risk of cognitive decline based on age and family history.
• Likelihood (): Impact of factors like exercise, diet, or mental stimulation if decline is occurring.
• Posterior Probability (): Updated likelihood of decline after implementing lifestyle changes.
This approach helps focus resources on high-impact areas, like targeted brain training or social engagement.
4. Fall Risk Prediction and Prevention
Bayes’ Theorem can combine multiple factors (e.g., muscle strength, home safety, vision) to predict and prevent falls.
Example:
• Prior Probability (): Probability of a senior experiencing a fall based on their age and health history.
• Likelihood (): Probability of environmental hazards or physical limitations leading to a fall.
• Posterior Probability (): Adjusted fall risk based on assessments, enabling targeted interventions like physical therapy or home modifications.
5. Decision-Making for Senior Care
Bayesian frameworks can support decision-making in caregiving, balancing the probabilities of different outcomes.
Example: Choosing Between Assisted Living and Home Care
• Prior Probability (): Baseline satisfaction level with assisted living.
• Likelihood (): Observed quality of life improvements under assisted living conditions.
• Posterior Probability (): Updated probability that assisted living will improve life quality after considering personal preferences and outcomes.
6. Adaptive Learning and Cognitive Training
Bayesian models optimize cognitive training programs for seniors by tailoring exercises to individual progress.
Example:
• Prior Probability (): Baseline effectiveness of a training method.
• Likelihood (): Improvement in memory or reasoning observed in sessions.
• Posterior Probability (): Updated effectiveness, leading to adjustments in training difficulty or focus.
Conclusion
By applying Bayes’ Theorem, caregivers and seniors can:
1. Make informed, personalized decisions.
2. Continuously adapt strategies based on outcomes.
3. Improve overall quality of life through targeted interventions.
Bayesian approaches integrate multiple data points—medical history, lifestyle, and preferences—into a unified framework for decision-making, ensuring tailored and effective care.
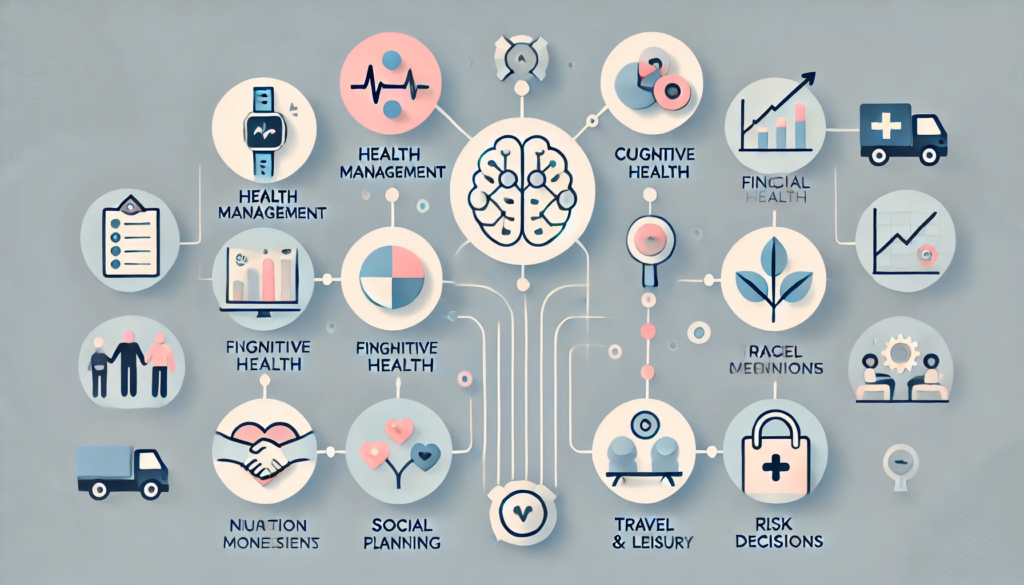
Bayesian inference can guide aging seniors in optimizing decisions by systematically updating their understanding of uncertain situations based on new evidence. Below are specific examples across various aspects of life:
1. Health Management
Bayesian inference can help seniors make data-driven choices about health risks, treatments, and preventive care.
• Example: Choosing a Treatment Plan
• Prior: Initial belief about treatment effectiveness based on medical advice or personal experience.
• Evidence: Success rates, side effects, and test results.
• Decision: Choose the treatment plan with the highest updated (posterior) probability of success while minimizing risks.
• Example: Assessing Fall Risk
• Prior: Baseline risk of falling based on age and mobility.
• Evidence: New observations like balance tests or home safety evaluations.
• Decision: Update safety measures such as installing grab bars or starting physical therapy.
2. Cognitive Health
Bayesian inference can optimize cognitive training programs and preventive strategies for dementia or memory decline.
• Example: Adapting Brain Training
• Prior: Initial effectiveness of puzzles or memory exercises based on research.
• Evidence: Personal improvement in memory scores.
• Decision: Focus on activities showing the most benefit, like spatial reasoning or vocabulary training.
• Example: Lifestyle Interventions
• Prior: Belief that regular exercise reduces cognitive decline risk.
• Evidence: Feedback from health markers like energy levels or memory performance.
• Decision: Adjust intensity or type of exercise to maximize cognitive benefit.
3. Financial Planning
Bayesian inference can assist seniors in managing investments, retirement funds, and budgeting.
• Example: Investment Strategy
• Prior: Risk level of an investment portfolio based on historical data.
• Evidence: Changes in market conditions or financial advice.
• Decision: Update asset allocation (e.g., stocks vs. bonds) to maintain desired risk levels.
• Example: Budgeting for Healthcare
• Prior: Expected healthcare costs based on average statistics.
• Evidence: New medical needs or insurance changes.
• Decision: Adjust the budget to prioritize health-related expenses.
4. Social Connections
Bayesian inference can guide decisions on maintaining or improving social interactions.
• Example: Choosing Social Activities
• Prior: Belief that attending a senior center increases social satisfaction.
• Evidence: Feedback from attending sessions (e.g., mood improvements or new friendships).
• Decision: Decide whether to attend more often, try new activities, or join a different group.
5. Nutrition and Fitness
Seniors can use Bayesian reasoning to optimize diet and exercise routines for health and energy.
• Example: Dietary Adjustments
• Prior: Belief that a Mediterranean diet improves cardiovascular health.
• Evidence: Improved blood pressure or cholesterol levels after following the diet.
• Decision: Continue, refine, or switch to another diet based on the updated belief.
• Example: Exercise Regimens
• Prior: Expectation that walking 30 minutes daily improves mobility.
• Evidence: Observed changes in stamina or joint pain.
• Decision: Adjust the frequency, intensity, or type of exercise.
6. Travel and Leisure
Bayesian inference can help seniors decide how to allocate time and energy for hobbies or travel.
• Example: Planning a Vacation
• Prior: Belief that visiting a new city will be enjoyable based on past experiences.
• Evidence: Reviews from peers, current health status, or travel costs.
• Decision: Choose destinations with the highest updated probability of satisfaction.
• Example: Pursuing New Hobbies
• Prior: Belief that gardening is relaxing.
• Evidence: Feedback from time spent gardening (e.g., stress reduction or physical strain).
• Decision: Invest more time in gardening or try a less physically demanding activity.
7. Risk Management
Bayesian inference can assist seniors in managing risks in daily life, like driving or home safety.
• Example: Deciding When to Stop Driving
• Prior: Confidence in driving skills based on recent experiences.
• Evidence: Frequency of minor mistakes or advice from family.
• Decision: Continue driving with modifications (e.g., driving only during daylight) or stop altogether.
• Example: Home Safety Enhancements
• Prior: Belief that the home is relatively safe.
• Evidence: Observations of tripping hazards or lighting issues.
• Decision: Make targeted improvements like better lighting or removing clutter.
8. Caregiving Decisions
Bayesian inference can help seniors and caregivers decide on care arrangements or living environments.
• Example: Choosing Assisted Living
• Prior: Initial belief about satisfaction in assisted living based on peer reviews.
• Evidence: Tours, trial stays, and discussions with residents.
• Decision: Opt for assisted living or enhance home care based on updated beliefs.
Conclusion
Bayesian inference empowers aging seniors to make personalized, data-driven decisions by continuously updating their beliefs with new evidence. Whether for health, finances, or daily living, it provides a structured framework to navigate uncertainty and optimize outcomes.
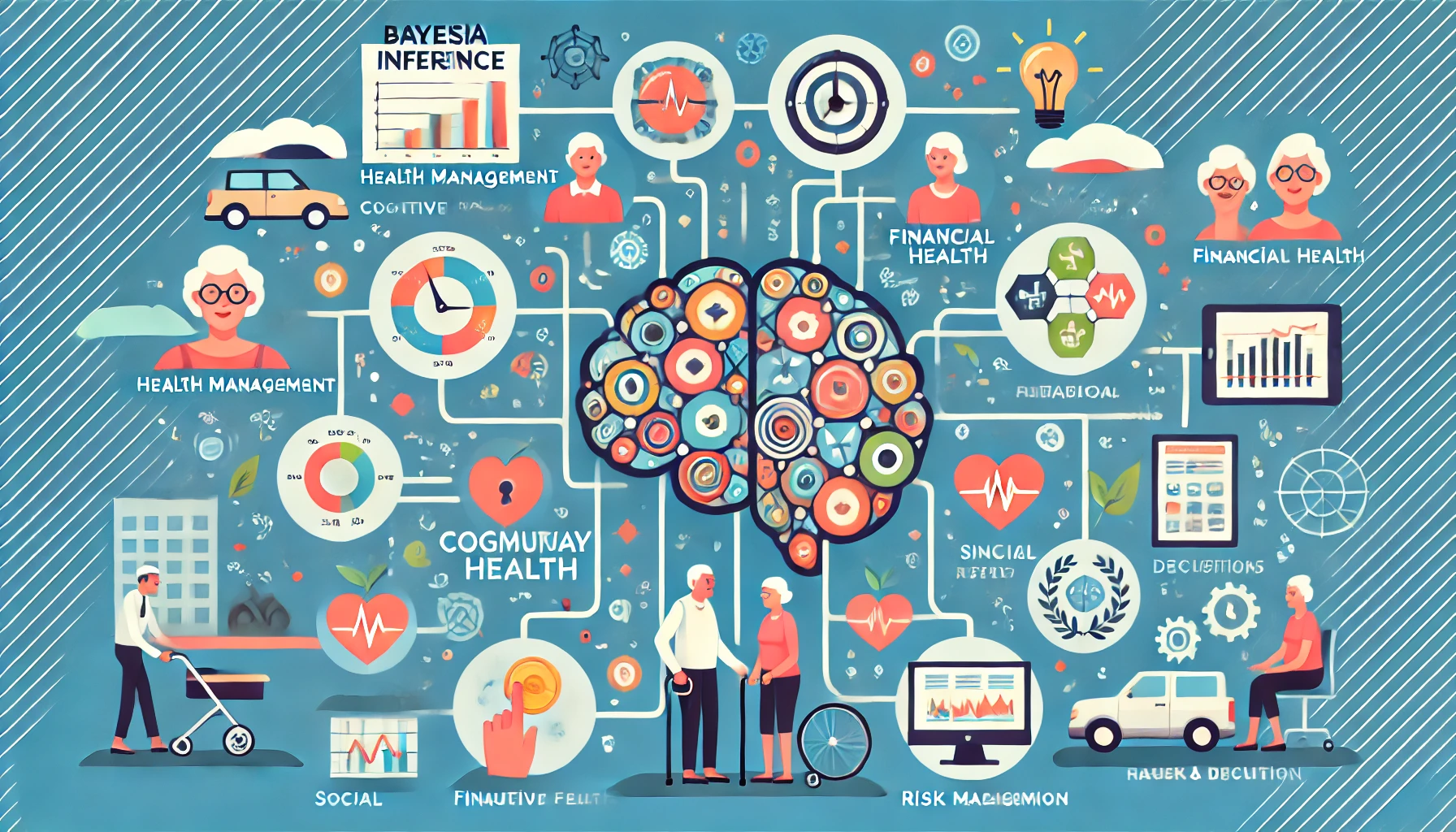