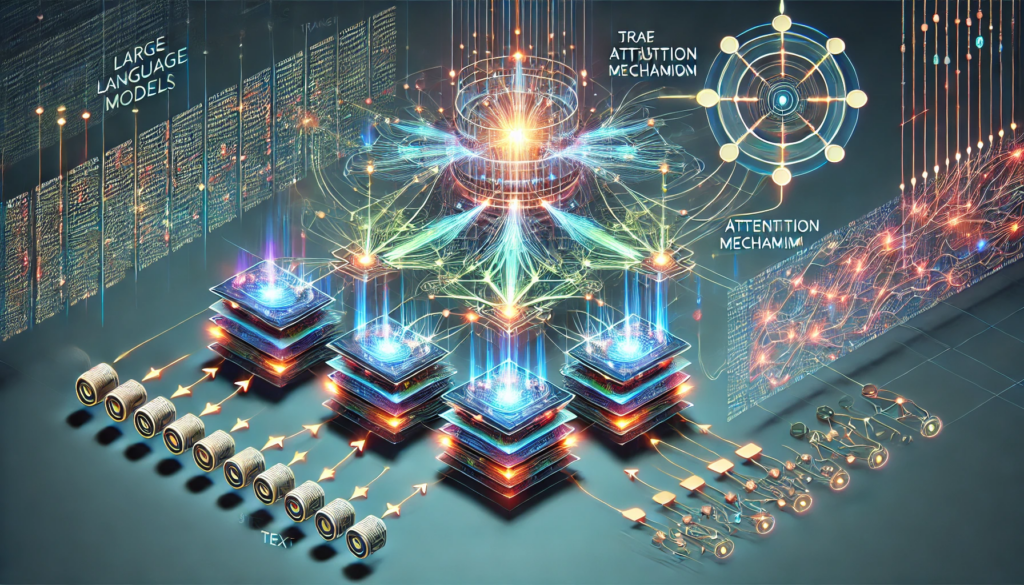
ChatGPT:
🚀 Transformers Revolutionized AI: What Will Replace Them?
Introduction
The 2017 research paper “Attention Is All You Need” by Google introduced transformers, a deep learning architecture that revolutionized artificial intelligence. Over the past six years, transformers have become the backbone of advanced AI systems like ChatGPT, GPT-4, Stable Diffusion, and GitHub Copilot. Their ability to process data in parallel through an attention mechanism has made them dominant across domains, from natural language processing to biology and robotics. However, transformers face limitations, particularly in computational cost and scalability, prompting researchers to explore new architectures that might eventually replace them.
The Rise of Transformers
The Breakthrough Paper
The transformer architecture, introduced by eight researchers at Google, was designed to replace recurrent neural networks (RNNs) by focusing entirely on attention mechanisms. This shift eliminated the sequential processing bottlenecks of RNNs and enabled parallel processing of data.
Key contributors to the paper, such as Ashish Vaswani and Jakob Uszkoreit, have since founded AI startups, further advancing AI technologies.
Attention Mechanism
The defining feature of transformers is their ability to use attention to determine relationships between words, regardless of sequence order. Unlike RNNs, which process data sequentially, transformers analyze all words simultaneously, improving both efficiency and accuracy.
Parallelization and Scalability
Transformers leverage GPUs, which are optimized for parallel computation, enabling the training of massive models like GPT-4. This scalability has driven breakthroughs in generative AI, from chatbots to image generation tools like Midjourney.
The Challenges of Transformers
Computational Costs
Transformers’ strength—scaling to massive datasets—becomes a weakness due to quadratic scaling. As sequence length increases, computational requirements grow exponentially, making them costly and inefficient for long inputs.
• Example: Doubling a sequence length from 32 to 64 tokens increases computation by four times instead of two.
• GPT-4’s context window of 32,000 tokens still struggles with processing longer sequences like entire books or genomes.
Hardware Dependence
Transformers require enormous computing power, leading to global shortages of GPUs and skyrocketing costs. For example, OpenAI raised $10 billion and Inflection secured $1 billion to sustain their AI operations.
Handling Long Sequences
Despite modifications like Longformer and Big Bird, transformers still face limitations when handling extremely long sequences, such as DNA strands or entire books.
Emerging Alternatives to Transformers
1. Hyena – Subquadratic Scaling
Developed at Stanford, Hyena replaces transformers’ attention mechanism with long convolutions and element-wise multiplication.
Key Features:
• Handles long sequences efficiently by scaling sub-quadratically.
• Demonstrates superior performance for datasets requiring long contexts.
• Example: At 64,000-token inputs, Hyena is 100 times faster than transformers.
Applications:
• HyenaDNA processes genomic data, leveraging its 1-million-token context window to handle complex biological sequences.
• Potential use cases include personalized medicine and genome analysis.
2. Liquid Neural Networks – Adaptability
Developed at MIT, liquid neural networks are inspired by biological brains and adjust their weights dynamically based on input.
Advantages:
• Smaller and more explainable than transformers.
• Require fewer parameters—MIT’s autonomous vehicle demo used just 253 parameters.
• Suited for time-series data and robotics.
Limitations:
• Cannot process static data like images or text effectively.
• Better suited for specialized tasks such as autonomous driving or drone navigation.
3. Swarm Intelligence Models
Sakana AI, founded by Llion Jones, explores architectures inspired by nature, focusing on collaborative, decentralized systems.
Key Principles:
• Collective intelligence modeled after swarms in nature.
• Small models work together instead of relying on a single, massive architecture.
• Prioritizes adaptability and learning-based approaches.
Challenges:
• Remains in early stages, requiring proof of scalability and performance.
Domain-Specific Models
4. Genomics and Biology
HyenaDNA’s ability to handle extremely long sequences highlights its potential for genomics, where entire DNA strands need analysis.
Example:
• HyenaDNA’s 1-million-token context window processes human genomes with 3.2 billion nucleotides.
• Future applications may include personalized health predictions and drug response modeling.
5. Robotics and Time-Series Data
Liquid neural networks offer promise for robotics due to their adaptability and explainability.
Key Advantage:
• Real-time learning makes them ideal for environments requiring fast decision-making, such as autonomous vehicles and drones.
Key Technical Challenges
1. Scalability
While transformers dominate AI today, alternatives like Hyena and Monarch Mixer focus on subquadratic scaling to reduce computational costs.
2. Explainability
Transformers operate as “black boxes,” making their decision-making opaque. New architectures aim to improve transparency, particularly in safety-critical fields like healthcare.
3. Continuous Learning
Transformers lack continuous learning—they cannot adapt based on new inputs once trained. Liquid neural networks address this with dynamic weight updates.
What’s Next for AI?
Domain-Specific Models
Future AI may shift toward specialized architectures optimized for distinct applications rather than general-purpose systems like transformers.
Hybrid Approaches
Combining elements from multiple architectures, such as Hyena’s convolution-based operations and liquid networks’ adaptability, could produce more versatile AI models.
Cost-Efficiency Innovations
Reducing computational demands remains a priority, enabling broader accessibility and sustainability for AI development.
Conclusion
Transformers have set the standard for AI development, but their computational costs and scalability issues create openings for new architectures. Models like Hyena, liquid neural networks, and swarm intelligence systems propose solutions to overcome these limitations. While transformers remain dominant, future advancements may produce hybrid or domain-specific architectures that surpass them in performance and efficiency. The AI landscape is rapidly evolving, and these emerging technologies could redefine the future of artificial intelligence.
Key Questions Moving Forward:
1. Will Hyena scale effectively to match GPT-4-sized models?
2. Can liquid neural networks handle broader applications outside robotics?
3. Will hybrid architectures combining transformer features emerge as dominant models?
As AI continues to develop at a breakneck pace, the next transformative breakthrough may be closer than we think.
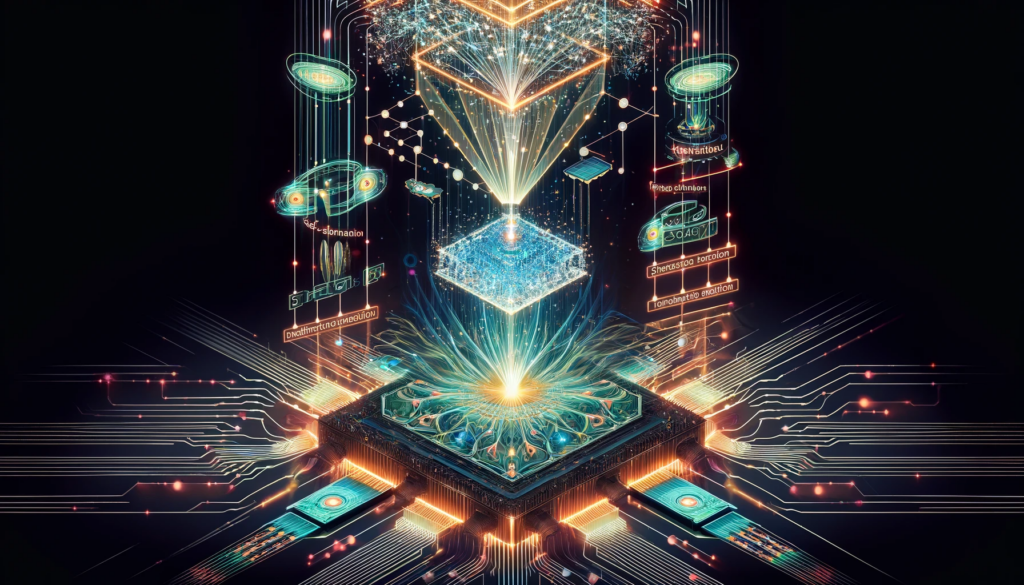
1. Will Hyena scale effectively to match GPT-4-sized models?
Hyena, developed at Stanford, is an emerging AI architecture designed to address transformers’ scalability and computational limitations. It achieves sub-quadratic scaling by replacing attention mechanisms with long convolutions and element-wise multiplication, enabling faster processing of long sequences.
Scaling Challenges:
• Current Hyena models are relatively small, with a maximum size of 1.3 billion parameters, whereas GPT-4 is rumored to have 1.8 trillion parameters.
• Scaling Hyena to GPT-4’s size requires extensive testing to ensure its computational efficiency and performance hold up at larger scales.
Advantages for Scaling:
• Hyena’s design reduces computational costs as sequence length increases, making it inherently more scalable for tasks involving long contexts (e.g., DNA analysis, full-length books).
• Its architecture processes inputs more efficiently, offering 100x speed improvements for sequences longer than 64,000 tokens.
Potential Limitations:
• Hyena’s scalability beyond billions of parameters remains unproven and requires further research.
• Compatibility with massive datasets, as used in GPT-4, may need optimizations in hardware utilization and data preprocessing pipelines.
Outlook:
If Hyena maintains efficiency and performance as it scales, it could challenge transformers for domains requiring long-context processing. However, widespread adoption will depend on proving its viability at GPT-4’s scale through real-world applications and benchmarks.
2. Can liquid neural networks handle broader applications outside robotics?
Liquid neural networks, inspired by biological neurons, dynamically adjust their weights based on incoming data, allowing them to adapt in real time. Their flexibility and efficiency make them particularly suited for robotics and time-series data processing.
Advantages in Broader Applications:
• Adaptability: Unlike transformers, liquid networks can continuously learn and adapt without retraining, enabling them to handle evolving datasets.
• Efficiency: With far fewer parameters (e.g., 253 parameters used in a self-driving system), they are computationally lightweight, making them ideal for edge devices and mobile systems.
• Explainability: Their smaller size improves interpretability, addressing one of the main criticisms of transformers as “black boxes.”
Challenges Beyond Robotics:
• Static Data Limitations: Liquid networks excel with sequential data but struggle with static data like images or text, which transformers handle more effectively.
• Scalability: While efficient for small-scale tasks, liquid networks have yet to demonstrate competitiveness for large-scale, general-purpose AI systems.
• Niche Focus: Their current focus on real-time, adaptive tasks may limit their adoption in broader fields like natural language processing or generative AI.
Outlook:
Liquid neural networks show strong potential for niche applications, particularly in areas requiring continuous learning, such as autonomous systems, healthcare monitoring, and financial forecasting. However, their scalability and performance in tasks dominated by transformers remain an open question.
3. Will hybrid architectures combining transformer features emerge as dominant models?
Given transformers’ dominance and the emergence of alternative architectures, hybrid models that combine transformers’ strengths with new innovations may define the next AI evolution.
Current Hybrid Approaches:
• Hyena Transformer Hybrids: Models combining Hyena’s convolutional approach with transformers’ attention mechanisms could offer the best of both worlds—efficiency and scalability.
• Vision Transformers (ViTs): Already combine transformer features with convolutional neural networks for image processing.
• Fusion Models: Mixing transformer components with graph neural networks or liquid neural networks to address specific weaknesses, such as explainability or adaptability.
Advantages of Hybrid Models:
• Efficiency: By incorporating sub-quadratic mechanisms like Hyena’s, hybrids could reduce computational costs while retaining transformers’ versatility.
• Domain-Specific Optimization: Hybrid designs can target specific use cases, such as biology, genomics, and robotics, without sacrificing general-purpose capabilities.
• Flexibility: Combining features from multiple architectures allows models to handle long sequences, adapt to real-time data, and scale more effectively.
Challenges:
• Complexity: Hybrid architectures may introduce added complexity, requiring more sophisticated training processes and infrastructure.
• Compatibility: Integrating multiple architectures must preserve performance and scalability without introducing bottlenecks.
• Evaluation: Benchmarks for hybrid models need to assess their performance across diverse domains, making validation more resource-intensive.
Outlook:
Hybrid architectures are likely to gain traction, especially for domain-specific AI tasks. However, their ability to replace transformers entirely depends on maintaining or exceeding the performance and scalability standards set by transformer-based models.
Final Thoughts
Hyena, liquid neural networks, and hybrid models represent promising alternatives or complements to transformers, each addressing specific weaknesses such as computational inefficiency, adaptability, and explainability. While transformers continue to dominate AI, the field’s rapid progress suggests that these innovations could reshape AI architectures, either through competition or integration.
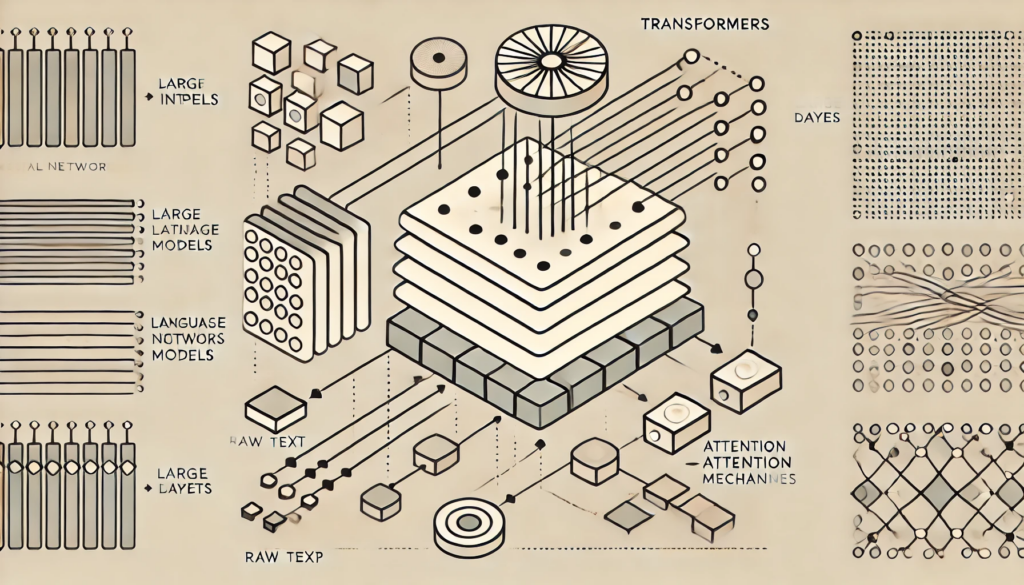
Q&A
Q1: What is a transformer in AI?
A: A transformer is a deep learning architecture introduced in 2017 that uses attention mechanisms for parallel processing of data, enabling scalability and efficiency.
Q2: Why are transformers so influential in AI?
A: Transformers enable breakthroughs in natural language processing, image generation, and robotics by processing data in parallel, making them scalable for large datasets.
Q3: What limitations do transformers face?
A: Transformers require high computational resources due to quadratic scaling with sequence length, limiting their efficiency with very long inputs.
Q4: What are Hyena models?
A: Hyena models are new AI architectures using convolutions instead of attention, offering sub-quadratic scaling to handle long sequences more efficiently.
Q5: How does Hyena outperform transformers?
A: Hyena scales better with long sequences, becoming 100 times faster than transformers at 64,000-token lengths while maintaining similar performance.
Q6: What are liquid neural networks?
A: Inspired by biology, liquid neural networks dynamically update their weights based on inputs, making them adaptive and transparent.
Q7: Where are liquid neural networks useful?
A: They are ideal for robotics and time-series data due to their adaptability, requiring fewer parameters and providing explainable outputs.
Q8: What is swarm intelligence in AI?
A: Swarm intelligence involves decentralized AI systems working collaboratively, inspired by nature, to enhance adaptability and efficiency.
Q9: Who is behind Sakana AI, and what is its focus?
A: Sakana AI, founded by Llion Jones, focuses on building AI architectures inspired by evolution and collective intelligence principles.
Q10: Will transformers remain dominant in AI?
A: While transformers are currently dominant, emerging architectures like Hyena, liquid networks, and swarm models may replace them in specific domains or use cases.
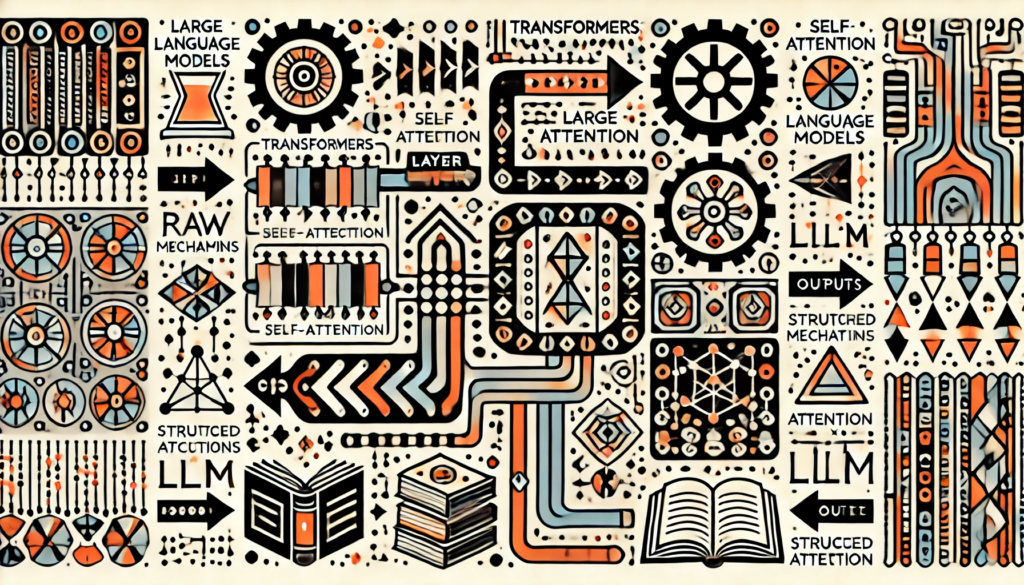