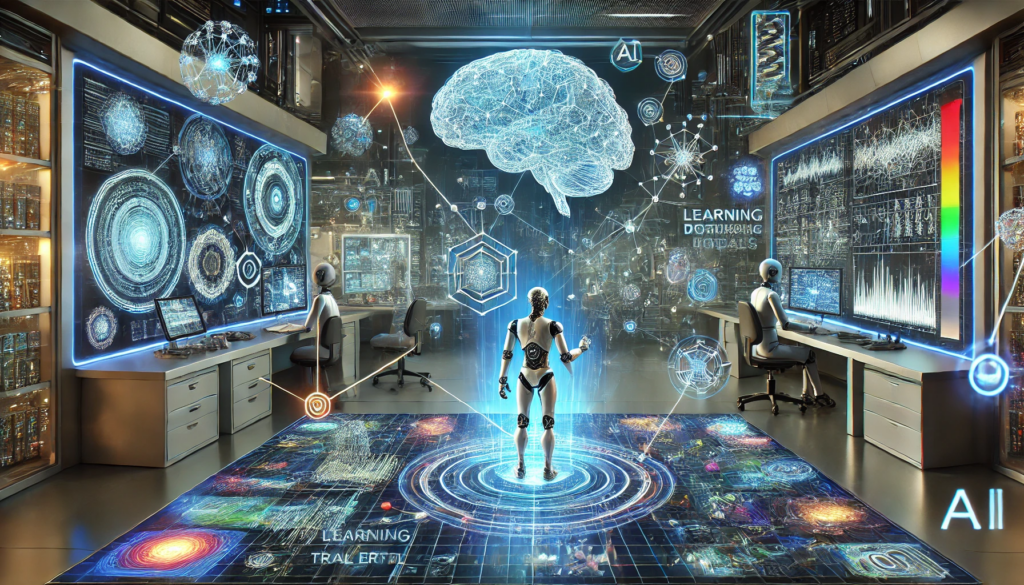
ChatGPT:
Deep Learning, Reinforcement Learning, and World Models
Introduction
This summary explores advancements in deep learning (DL) and reinforcement learning (RL), focusing on their integration to achieve human-like intelligence. It highlights key frameworks like world models, self-supervised learning, and hierarchical reinforcement learning, emphasizing methods to improve data efficiency, planning, and adaptation. The paper also discusses probabilistic models, graphical learning frameworks, and meta-learning approaches that enhance AI performance and adaptability.
🧠 Deep Learning and World Models
1. World Models for Perception and Control
World models simulate environments, enabling AI to predict actions and learn behaviors based on interactions.
• Multimodal AI integrates data from different sensory inputs for robust decision-making.
• Joint Multimodal Variational Autoencoders (JMVAE) handle missing data by reconstructing inputs through learned representations.
• Behavior Regularized Offline RL (BREMEN) reduces data requirements for policy optimization, achieving efficiency with fewer interactions.
Example Applications:
• Predicting object behavior in physical environments using simulated interactions.
• Efficient decision-making models for robotics and autonomous systems.
🧩 Self-Supervised Learning
2. Learning Without Labels
Self-supervised learning (SSL) trains AI by predicting missing data, requiring no explicit supervision.
Key Features:
• Mimics how humans learn by observing patterns and filling gaps.
• Builds hierarchical representations for language (e.g., BERT) and vision (e.g., SimCLR).
• Utilizes Energy-Based Models (EBMs) for uncertainty prediction in dynamic systems.
Example Applications:
• Autonomous driving simulations predicting car movements.
• Video and image processing for future event predictions.
🌐 Probabilistic Graphical Models
3. Learning Structures for Inference
Graphical models capture statistical dependencies to improve AI reasoning.
• Directed models link causal relationships.
• Undirected models infer dependencies between variables.
Dynamic Neural Systems:
• Distributive Distributional Coding (DDC) models encode probability distributions in neural circuits.
• Recurrent structures in neural networks mimic brain connectivity for learning and inference.
Key Concepts:
• Representations of uncertainty for dynamic processes.
• Applications in predicting causal interactions in changing environments.
🎮 Reinforcement Learning Frameworks
4. Policy Optimization and Generalized Learning
Reinforcement learning (RL) focuses on maximizing rewards through interaction.
Approaches:
• Generalized Policy Updates (GPU) reuse learned policies to speed up adaptation to new tasks.
• Successor Features enable knowledge transfer across related tasks, reducing data requirements.
Deep Reinforcement Learning (DRL):
• Combines neural networks with RL to learn strategies, as seen in AlphaGo and MuZero.
• Model-based RL frameworks like Dreamer V2 improve efficiency through simulated training environments.
⚙️ Hierarchical and Composite RL
5. Breaking Down Complex Tasks
Hierarchical RL decomposes tasks into subtasks, mimicking human-like strategies.
• Efficient for motor control and navigation tasks.
• Used for robotic arms and humanoid robots.
Compositionality Theory:
• Combines pre-trained policies to handle novel tasks.
• Reduces computation by leveraging prior knowledge.
🛠 Advanced Techniques for Data Efficiency
6. Entropy Regularization
Enhances exploration and decision-making by encouraging randomness in policy selection.
• Soft Actor–Critic (SAC) improves sample efficiency and learning stability.
• KL-Divergence prevents drastic policy updates, stabilizing training.
🚀 Simulation-Based Learning
7. Sim-to-Real Transfer
AI policies are trained in simulated environments before deployment, minimizing real-world risks.
• MuZero learns dynamics from visual data and actions, achieving efficient decision-making in games.
• Dreamer V2 trains latent models for complex tasks using fewer resources.
🌟 Future Directions
8. Meta-Learning and Lifelong AI
AI systems are evolving to learn multiple tasks with minimal supervision, adapting meta-parameters like learning rates.
• Meta-RL enhances adaptability to new goals with fewer iterations.
• Promotes self-learning rules for task-independent optimization.
9. Human-Inclusive AI
AI collaborates with humans in creative fields like art and design.
• Example: AI-assisted dress designs combined computational creativity with human intuition.
• Focus on enriching society through human-AI synergy.
Conclusion
The paper emphasizes that deep learning and reinforcement learning are critical to achieving brain-like intelligence.
• World Models simulate environments, providing efficient learning strategies.
• Self-supervised learning reduces reliance on labeled data, enhancing scalability.
• Hierarchical and compositional RL mirrors human problem-solving, improving adaptability.
• Entropy regularization and meta-learning strategies push AI toward autonomy and lifelong learning.
Future AI systems will rely on these methods to combine learning efficiency with creative capabilities, supporting broader real-world applications.
Key Takeaways
🧠 World Models: Simulate and predict environmental dynamics for efficient decision-making.
📊 Multimodal Learning: Integrates diverse sensory inputs, addressing missing data challenges.
🔍 Self-Supervised Learning: Enables unsupervised data-driven learning through prediction tasks.
🌐 Graphical Models: Represent probabilistic relationships to support inference and adaptation.
🎮 Reinforcement Learning: Optimizes rewards to develop adaptive AI strategies.
⚙️ Generalized Policy Updates: Transfers prior knowledge to speed up learning in new tasks.
💡 Hierarchical RL: Uses modular frameworks for complex problem-solving.
🦾 Simulation-Based Training: Prepares AI through virtual interactions before real-world deployment.
🚀 Meta-Learning: Enables lifelong learning and quick adaptation to multiple tasks.
🤖 Human-AI Collaboration: Enhances creativity and design through AI-human partnerships.
Applications and Impact
• Robotics: Advanced control systems for humanoids and drones.
• Games: High-performance algorithms like AlphaGo and MuZero.
• Autonomous Vehicles: Predictive systems for navigation and safety.
• Creativity: AI tools for art and design.
• Healthcare: AI-driven diagnostics and decision-making systems.
FAQs
1. What are world models in AI and how are they used?
World models simulate environments to predict and control actions, enabling AI to learn behaviors through interaction.
2. How does self-supervised learning improve AI training?
It predicts missing data without labeled datasets, allowing unsupervised learning and better generalization.
3. What is entropy regularization in reinforcement learning?
It encourages exploration by maximizing the entropy of policies, balancing randomness and learning efficiency.
4. How does hierarchical reinforcement learning (HRL) mimic human learning?
HRL breaks tasks into smaller subtasks, enabling modular and layered strategies similar to human decision-making.
5. What role does meta-learning play in AI?
Meta-learning helps AI quickly adapt to new tasks by optimizing learning rules and hyperparameters automatically.
6. How do graphical models enhance AI inference?
They capture probabilistic relationships, improving predictions and adaptability to dynamic environments.
7. What is the significance of MuZero in AI development?
MuZero learns latent models from image data, enabling decision-making without predefined rules.
8. How does reinforcement learning differ from supervised learning?
Reinforcement learning focuses on maximizing rewards through exploration, while supervised learning uses labeled data.
9. What is the advantage of compositionality in AI models?
It combines pre-trained policies to handle new tasks efficiently, reducing computational costs.
10. What is the primary benefit of human-inclusive AI?
Human-inclusive AI enhances creativity and collaboration, especially in fields like arts and design.
Recent advancements in deep learning (DL) and reinforcement learning (RL) have significantly impacted various industries, leading to more efficient and intelligent systems. Here are some notable developments:
1. Integration of World Models in AI
World models, or world simulators, have gained prominence as a means to enhance AI’s understanding and interaction with complex environments. These models enable AI systems to predict outcomes and make informed decisions by simulating real-world scenarios. For instance, Fei-Fei Li’s World Labs has raised substantial funding to develop large world models, aiming to create systems that generate entire worlds with their own physics and logic.
2. Practical Applications of AI Tools
In 2024, the focus of artificial intelligence shifted from creating large models to developing practical applications. AI tools like generative chatbots found widespread use in tech services, while significant investments poured into their development. However, AI’s high costs and limited productivity led to tempered expectations about its transformative potential. Concerns about job replacement by AI and ethical issues persist across various professions, including entertainment and law. AI remains limited by its lack of common sense and is expected to evolve into more useful ‘agents’ capable of complex reasoning.
3. Advances in Deep Reinforcement Learning (DRL)
DRL, which combines deep learning and reinforcement learning, has seen significant progress, particularly in robotic manipulation tasks. Recent studies have focused on improving perception, assembly, multitasking, transfer learning, and human-robot interaction. These advancements aim to make AI systems more trustworthy, interpretable, and explainable.
4. Quantum Computing Breakthroughs
Google announced a quantum computing breakthrough with its Willow chip, which solved a complex math problem in five minutes, illustrating the immense potential of quantum technology. This advancement showcases notable progress in the field, despite not being as headline-grabbing as past innovations.
5. AI in Scientific Research
AI-powered tools are transforming content creation, research, and workflow automation, allowing faster, cost-effective solutions and transforming business operations and communication. For instance, AI-powered research tools will transform how data is analyzed and insights are generated by 2025. These tools will empower researchers, students, and professionals to streamline information gathering.
6. AI in Creative Industries
AI is increasingly being used in creative fields, enhancing human creativity and collaboration. For example, AI-assisted dress designs combine computational creativity with human intuition, showcasing the potential for combining AI with human creativity in arts and design.
7. Ethical and Societal Considerations
The rapid advancement of AI technologies has raised ethical concerns, particularly regarding job displacement and the need for responsible AI development. Experts emphasize the importance of addressing these challenges to ensure AI benefits society as a whole.
These developments highlight the dynamic nature of AI research and its expanding influence across various sectors. As AI continues to evolve, ongoing research and collaboration will be crucial in addressing challenges and harnessing its full potential.
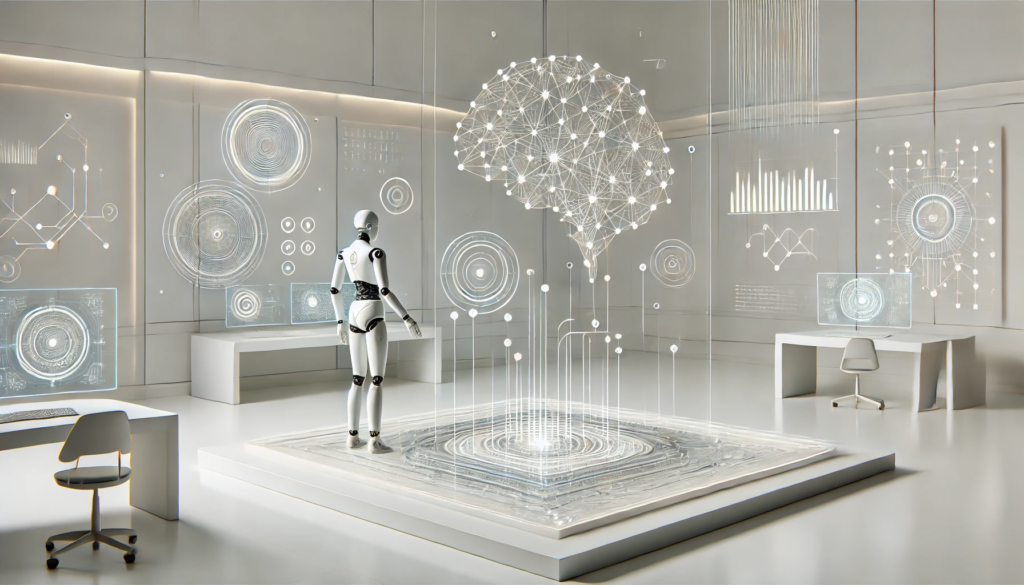