ChatGPT:
The “butterfly effect” is a concept from chaos theory that describes how small changes in initial conditions can lead to vastly different outcomes. The term was popularized by meteorologist Edward Lorenz in the 1960s.
Origin
Lorenz discovered the butterfly effect while working on weather prediction models. In a 1963 paper, he described how minute changes in the starting point of his weather simulations (such as rounding off a number) could result in completely different weather patterns. He famously illustrated this with the metaphor that the flap of a butterfly’s wings in Brazil could set off a tornado in Texas.
Explanation
The butterfly effect underscores the sensitivity of complex systems to initial conditions. In mathematical terms, it shows how non-linear dynamics can amplify small perturbations over time. This means that in systems like the weather, predicting long-term behavior becomes extremely difficult, as tiny variations can grow into significant differences.
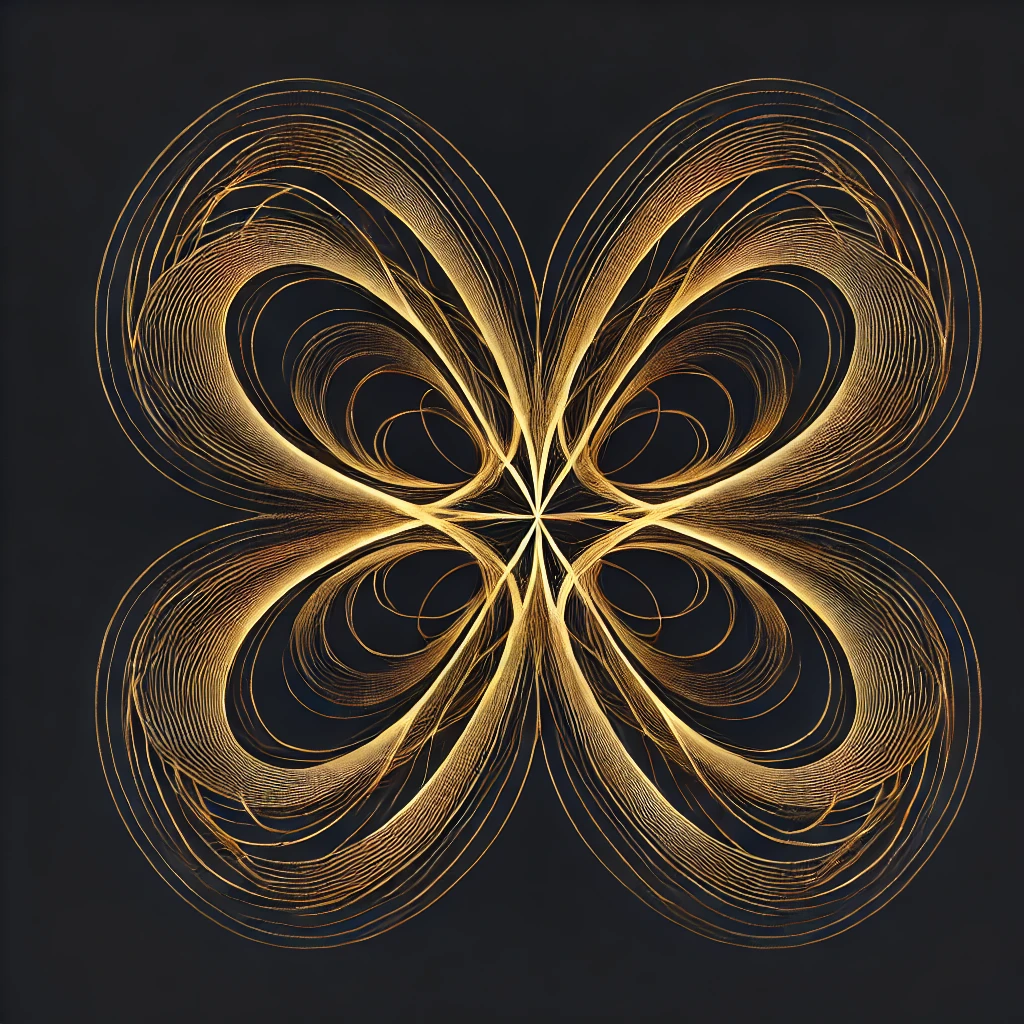
Chaos theory is a branch of mathematics and physics that deals with systems that appear to be disordered or random, but are actually governed by underlying patterns and deterministic laws. These systems exhibit sensitive dependence on initial conditions, meaning that small variations in the starting point can lead to vastly different outcomes. Here’s a detailed explanation of chaos theory:
Key Concepts
- Deterministic Systems: Despite their apparent randomness, chaotic systems are deterministic. This means that their behavior is entirely determined by their initial conditions and the laws governing their evolution. There is no randomness in the equations themselves.
- Sensitivity to Initial Conditions: Often illustrated by the butterfly effect, this concept highlights how small differences in the initial state of a system can lead to significantly different outcomes. This makes long-term prediction impossible in practice for chaotic systems.
- Nonlinearity: Chaotic systems are typically nonlinear, meaning that changes in output are not proportional to changes in input. This nonlinearity can create feedback loops where small effects can become amplified over time.
- Strange Attractors: In the phase space of a chaotic system, where each point represents a state of the system, strange attractors are fractal structures toward which the system evolves over time. These attractors have a complex, non-repeating structure, yet they govern the long-term behavior of the system.
- Fractals: Many chaotic systems exhibit fractal geometry, where structures are self-similar at different scales. Fractals are a hallmark of the complex, detailed patterns that emerge from simple deterministic rules.
- Bifurcation: This is a process where a small change in a system’s parameter value causes a sudden qualitative or topological change in its behavior. Bifurcations are often seen in chaotic systems as they transition from orderly to chaotic behavior.
Examples of Chaotic Systems
- Weather Systems: The atmosphere is a classic example of a chaotic system, where small variations in initial conditions can lead to vastly different weather patterns, making long-term weather forecasting difficult.
- Double Pendulum: A simple mechanical system that exhibits chaotic behavior. The motion of a double pendulum (a pendulum with another pendulum attached to its end) is highly sensitive to initial conditions.
- Population Dynamics: In ecology, the population sizes of certain species can exhibit chaotic fluctuations based on nonlinear interactions with their environment and other species.
- Electrical Circuits: Certain electronic circuits can exhibit chaotic behavior due to the nonlinear components and feedback loops.
Applications of Chaos Theory
- Meteorology: Understanding the limits of predictability in weather forecasting.
- Engineering: Designing systems that can tolerate or mitigate the effects of chaos, such as in control systems or communication networks.
- Economics: Analyzing market dynamics and financial systems that exhibit chaotic behavior.
- Medicine: Studying the irregular rhythms in cardiac and neural activity to understand and predict medical conditions.
- Environmental Science: Modeling the complex interactions within ecosystems and predicting their responses to various changes.
Implications
- Unpredictability: Chaos theory demonstrates that even deterministic systems can be fundamentally unpredictable over the long term, challenging the classical notion of predictability in science.
- Complexity from Simplicity: It shows how complex, seemingly random behavior can arise from simple deterministic rules, offering insights into the nature of complexity in the universe.
- Interdisciplinary Impact: Chaos theory has profound implications across various scientific and engineering disciplines, influencing how researchers approach problems involving complex systems.
Chaos theory fundamentally changes our understanding of order and randomness, illustrating that what appears chaotic may actually be governed by intricate and deterministic principles.
Feedback loops are mechanisms in which the output of a system influences its own input, often leading to self-regulation or amplification of certain behaviors within the system. There are two main types of feedback loops: positive feedback loops and negative feedback loops. Each type has distinct characteristics and effects on the system’s behavior.
Positive Feedback Loops
Definition: In a positive feedback loop, the output of a system enhances or amplifies the initial input, leading to an increase in the output. This process can create exponential growth or amplification within the system.
Characteristics:
- Amplification: Small changes can lead to significant increases in the system’s state.
- Runaway Effect: If unchecked, positive feedback can lead to runaway effects where the system grows uncontrollably.
- Destabilization: Positive feedback can make a system more unstable if not balanced by other factors.
Examples:
- Microphone Feedback: When a microphone picks up sound from a speaker, it amplifies it, which the speaker then outputs again, creating a loop that can lead to loud screeching.
- Ice-Albedo Effect: In climate science, melting ice reduces the Earth’s albedo (reflectivity), leading to more heat absorption and further ice melt.
- Economic Bubbles: Rising asset prices attract more investors, driving prices even higher, potentially leading to market bubbles.
Negative Feedback Loops
Definition: In a negative feedback loop, the output of a system counteracts or dampens the initial input, leading to stabilization and equilibrium within the system.
Characteristics:
- Stabilization: Helps maintain a system’s stability and balance.
- Self-Regulation: Negative feedback loops enable systems to self-regulate and correct deviations from a set point.
- Homeostasis: Common in biological systems to maintain internal stability.
Examples:
- Thermostat: A thermostat regulates temperature by switching heating or cooling devices on and off to maintain a desired temperature.
- Body Temperature Regulation: The human body maintains a stable temperature through sweating to cool down or shivering to warm up.
- Market Equilibrium: In economics, if prices rise too high, demand decreases, leading to a drop in prices, and vice versa.
Applications and Implications
Engineering:
- Control Systems: Feedback loops are fundamental in designing control systems, such as those used in robotics, automation, and aircraft stability.
- Electronic Circuits: Used in amplifiers and oscillators to control signal strength and frequency.
Biology:
- Homeostasis: Negative feedback loops are crucial for maintaining stable internal conditions, such as glucose levels, blood pressure, and pH balance.
- Ecological Systems: Feedback loops regulate population dynamics, resource availability, and ecosystem stability.
Economics:
- Supply and Demand: Negative feedback helps achieve market equilibrium, while positive feedback can lead to economic cycles and bubbles.
- Policy Interventions: Understanding feedback can help design policies to stabilize or stimulate economic activity.
Climate Science:
- Climate Models: Feedback loops, such as the ice-albedo effect or carbon cycle feedbacks, are key components in predicting climate change and its impacts.
Sociology:
- Social Dynamics: Feedback loops can explain phenomena such as social reinforcement, where behaviors and norms are amplified or stabilized within communities.
Summary
Feedback loops are essential mechanisms in both natural and engineered systems, driving self-regulation, stability, and change. Positive feedback loops amplify changes and can lead to exponential growth or instability, while negative feedback loops promote stability and equilibrium by counteracting deviations. Understanding these loops is crucial for analyzing, designing, and managing complex systems across various fields.
Following are examples of the butterfly effect’s applications in the fields mentioned above :
Meteorology
- Weather Forecasting: A slight change in temperature or pressure in one part of the world can significantly affect the weather patterns elsewhere. For example, a minor atmospheric disturbance in the Pacific Ocean can influence the development of hurricanes in the Atlantic.
Economics
- Stock Market: A seemingly insignificant event, like a small company’s unexpected success, can lead to large-scale investor behavior changes, affecting the stock market’s overall performance. An example is how minor technological advancements in a startup can lead to a tech boom or bust.
Ecology
- Ecosystem Changes: The introduction or removal of a single species can have cascading effects on the entire ecosystem. For example, the reintroduction of wolves to Yellowstone National Park drastically changed the park’s ecosystem, affecting populations of other animals and even the vegetation.
Sociology
- Social Movements: An individual’s small action, such as a social media post, can go viral and spark widespread social movements. The Arab Spring was partly catalyzed by social media posts that mobilized large groups of people.
Technology
- Cybersecurity: A minor vulnerability in a piece of software can be exploited, leading to significant breaches and widespread damage. For instance, the Heartbleed bug in OpenSSL, a seemingly small flaw, led to massive security vulnerabilities worldwide.
In the context of AI-driven large language models, the “butterfly effect” can be understood as the phenomenon where small changes or variations in the input data, training process, or initial conditions can lead to significantly different outcomes in the model’s behavior and responses. Here’s a detailed explanation of how this manifests in large language models:
Sensitivity to Initial Conditions
Training Data:
• Minor Data Variations: Small changes in the training dataset, such as the inclusion or exclusion of specific examples, can influence the model’s understanding and generation of language. For instance, adding a few sentences with specific vocabulary or topics might cause the model to produce more responses related to those areas.
• Bias Introduction: Tiny biases in the training data can lead to significant biases in the model’s outputs. Even subtle skewing of data can amplify stereotypes or particular viewpoints.
Model Parameters
Hyperparameters:
• Parameter Tuning: Small adjustments to hyperparameters (like learning rate, batch size, etc.) during the training process can lead to drastically different models. These parameters control how the model learns and adapts to data.
• Initial Weights: The initial weights of the neural network, often set randomly, can affect the trajectory of the training process, potentially leading to different learned patterns and behaviors.
Input Sensitivity
Prompt Variations:
• Subtle Changes in Input Prompts: Slight variations in the input prompt given to the model can produce vastly different outputs. For instance, changing a single word or punctuation in a query can alter the generated response significantly.
• Context Sensitivity: The model’s responses are highly context-sensitive. Small contextual changes can lead to different interpretations and outputs, demonstrating the model’s sensitivity to initial conditions.
Generative Behavior
Response Diversity:
• Stochastic Elements: Language models often use stochastic processes (random sampling) to generate text. Small changes in the sampling method or the seed value for randomness can lead to different text outputs.
• Creative Variations: Because the model can generate a wide range of responses to a given prompt, slight differences in input can steer the output in diverse directions, illustrating the model’s expansive generative capacity.
Implications
Bias and Fairness:
• Amplification of Bias: The butterfly effect in AI-driven language models can amplify minor biases present in the training data, leading to significant ethical and fairness concerns. Small biases can snowball into larger issues in the generated content.
• Unpredictable Outputs: The sensitivity to initial conditions can result in unpredictable or inconsistent outputs, challenging the reliability and consistency of the model’s responses.
Applications and Control:
• Fine-tuning and Adaptation: Understanding the butterfly effect in language models is crucial for fine-tuning and adapting models to specific tasks. Careful control of training conditions and data is necessary to achieve desired outcomes.
• Robustness Testing: Testing the robustness of models against small changes in input can help identify potential weaknesses and areas where the model may produce undesirable or biased outputs.
Ethical Considerations:
• Transparency and Accountability: Given the sensitivity of AI models to small changes, transparency in the training data, model parameters, and development processes becomes essential to ensure accountability and trustworthiness.
In summary, the butterfly effect in AI-driven large language models highlights the importance of careful management of data, training processes, and inputs. Understanding and mitigating the impacts of small changes can help develop more reliable, fair, and consistent AI systems.
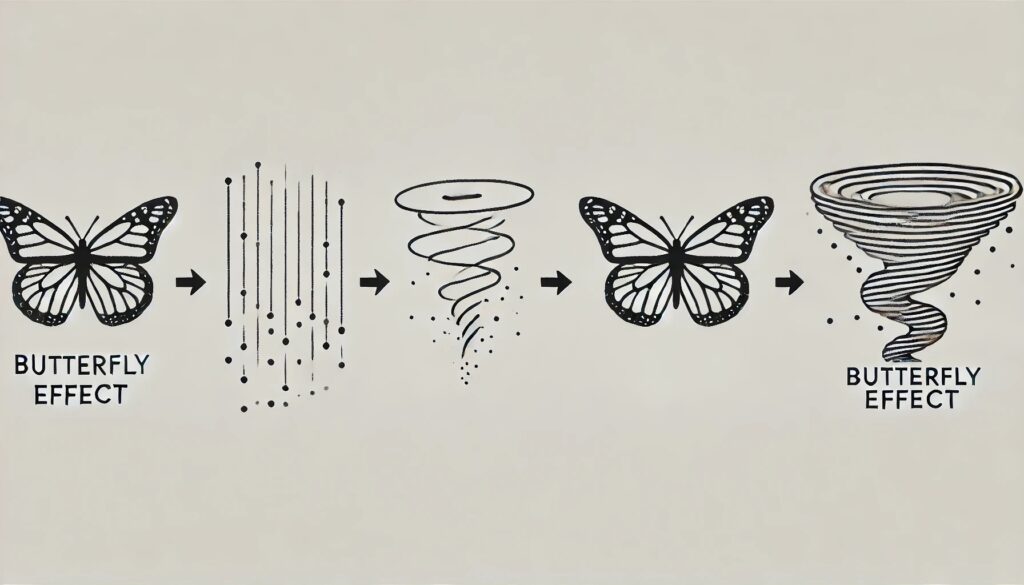
The underlying reasonings for these observations of the butterfly effect stem from the inherent complexity and interconnectedness of systems in each field. Here’s a detailed discussion:
Meteorology
- Complex Interactions: The atmosphere is a highly dynamic system where numerous factors such as temperature, pressure, humidity, and wind interact in complex ways. Small variations in these factors can propagate and amplify through these interactions, making precise long-term weather forecasting extremely challenging.
- Nonlinearity: Meteorological systems are nonlinear, meaning the relationship between variables is not directly proportional. A small change in one variable can lead to disproportionately large effects.
Economics
- Interconnected Markets: Financial markets are interconnected and influenced by numerous factors including investor behavior, economic policies, and global events. Small events can lead to changes in market sentiment, triggering broader market movements.
- Feedback Loops: Economic systems often have feedback loops where initial changes can reinforce or dampen subsequent behavior. For instance, an initial increase in stock prices can lead to more buying, further driving up prices.
Ecology
- Trophic Cascades: Ecosystems are structured in layers of interdependent relationships (food webs). Changes at one trophic level (e.g., predator introduction or removal) can cascade through the system, affecting multiple species and their interactions.
- Adaptive Behaviors: Species adapt to changes in their environment, which can lead to new behaviors that further alter the ecosystem. For example, changes in predator populations can influence prey behaviors, which in turn affect vegetation and other species.
Sociology
- Network Effects: Social behaviors are often influenced by networks of relationships and communications. Small actions can spread through social networks, influencing larger groups of people. Social media amplifies this effect, allowing information to spread rapidly and widely.
- Cultural Resonance: Individual actions can resonate with broader cultural or social sentiments, leading to collective actions. A small protest or viral post can tap into existing frustrations, mobilizing large-scale social movements.
Technology
- System Interdependencies: Modern technological systems are highly interconnected. A minor issue in one part of a network can propagate, causing widespread disruptions. For example, a software vulnerability can be exploited across multiple systems, leading to significant security breaches.
- Rapid Information Spread: In the digital age, information spreads rapidly. Small discoveries or innovations can quickly influence a wide array of applications and industries, leading to significant technological shifts.
General Reasoning
- Sensitivity to Initial Conditions: Across all these fields, a common thread is the sensitivity to initial conditions. Small differences or perturbations at the start can lead to vastly different outcomes due to the complex, interconnected nature of these systems.
- Nonlinear Dynamics: Many of these systems exhibit nonlinear dynamics, where small changes can have disproportionately large effects. This makes predicting outcomes challenging but also highlights the potential for significant impact from seemingly minor actions.
These observations underscore the importance of understanding and considering the intricate and often unpredictable nature of complex systems in various domains.
These examples illustrate how minor initial events or changes can have profound and far-reaching impacts in various domains, reflecting the butterfly effect’s core principle.
The acceptance of explanations for the butterfly effect varies across different fields, but generally, they are well-regarded and have been integrated into both theoretical frameworks and practical applications. Here’s a closer look at the acceptance and implications in each field:
Meteorology
- Wide Acceptance: The butterfly effect is widely accepted in meteorology. The recognition that small changes can lead to significant differences in weather outcomes is a fundamental principle in atmospheric sciences.
- Practical Implications: This understanding has led to the development of ensemble forecasting, where multiple simulations with slightly varied initial conditions are used to predict a range of possible outcomes, improving the accuracy and reliability of weather forecasts.
Economics
- Accepted with Nuances: Economists accept that small events can have large impacts, but the application of the butterfly effect is more nuanced. Economic models often incorporate factors like market sentiment and feedback loops, acknowledging the potential for small triggers to cause significant market shifts.
- Practical Implications: Policymakers and financial analysts use scenarios and stress testing to prepare for unexpected events, recognizing the potential for minor disturbances to lead to major economic consequences.
Ecology
- Broad Acceptance: The concept is well-accepted in ecology, particularly through the study of trophic cascades and ecosystem dynamics. Ecologists recognize that small changes can propagate through ecosystems, leading to significant ecological shifts.
- Practical Implications: Conservation efforts often consider the butterfly effect by focusing on keystone species and critical habitats, understanding that protecting or altering these can have wide-ranging impacts on the ecosystem.
Sociology
- Increasing Acceptance: Sociologists increasingly acknowledge the butterfly effect, particularly in the context of social networks and the spread of information. The idea that small actions can lead to large-scale social changes is supported by studies on viral phenomena and social movements.
- Practical Implications: Activists and policymakers use this understanding to strategize social campaigns and interventions, aiming to create significant change through targeted, small-scale actions.
Technology
- High Acceptance: The butterfly effect is highly accepted in technology, especially in cybersecurity and network theory. Small vulnerabilities are recognized as potential sources of major disruptions.
- Practical Implications: This has led to the implementation of robust security measures, continuous monitoring, and rapid response strategies to mitigate the effects of small issues before they escalate.
General Scientific Community
- Theoretical Acceptance: The butterfly effect is a cornerstone of chaos theory and is well-supported by mathematical models and empirical evidence across various scientific disciplines.
- Interdisciplinary Influence: Its acceptance transcends individual fields, influencing a broad range of scientific research and practical applications, from engineering to climate science.
Criticisms and Limitations
- Predictive Challenges: One criticism is the inherent unpredictability it implies, which can make long-term forecasting and planning difficult.
- Complexity in Application: Applying the butterfly effect in practical scenarios often requires sophisticated models and simulations, which can be resource-intensive.
Overall, while there are nuances in acceptance and application, the butterfly effect is a widely recognized and influential concept across multiple disciplines, shaping both theoretical understanding and practical approaches to complex systems.
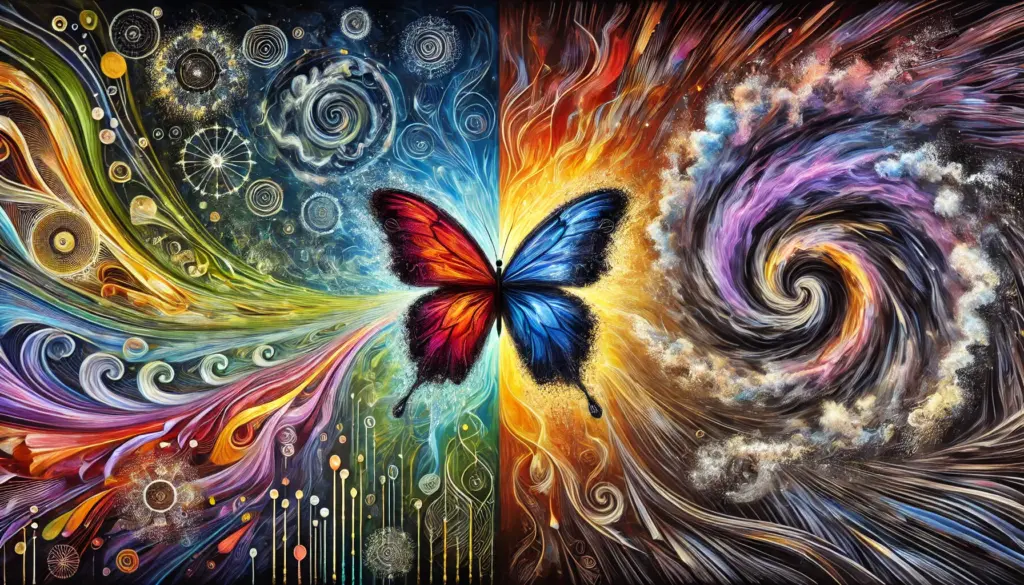