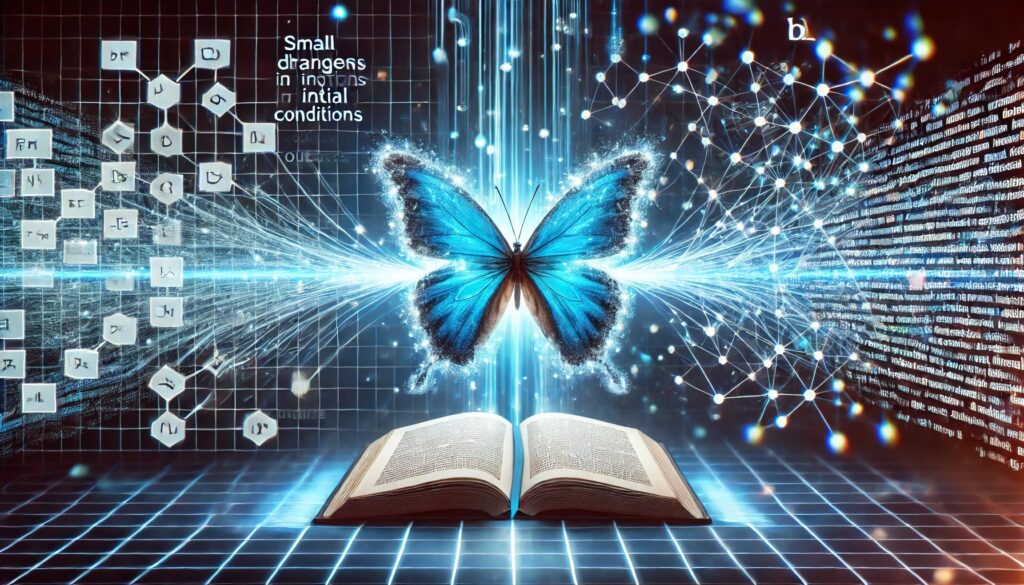
ChatGPT:
The “butterfly effect” is a concept from chaos theory, where small changes in initial conditions can lead to vastly different outcomes. In the context of Large Language Models (LLMs), this concept can help explain how hallucinations—incorrect or nonsensical outputs—can emerge.
When an LLM generates a response, it relies on patterns and associations learned from its training data. However, the model’s predictions are highly sensitive to initial inputs and the sequence of tokens processed. Here’s how the butterfly effect plays a role:
- Initial Prompt Variability: A minor change in the input prompt can significantly alter the model’s understanding of the context. This small change can cascade through the model’s layers, leading to different word predictions.
- Token Prediction Chain: Each predicted token influences the next one. An early prediction that deviates slightly from expected patterns can lead the model further off course with each subsequent prediction, amplifying the initial error.
- Complex Interactions: The model’s vast network of parameters interacts in complex ways. Small perturbations in the input can activate different pathways in the network, leading to unpredictable and sometimes erroneous outputs.
- Training Data Gaps: If the model encounters a prompt or context it wasn’t adequately trained on, small uncertainties can snowball into larger inaccuracies, causing it to “hallucinate” information that isn’t based on real data.
In essence, like the butterfly effect where a small change can lead to a hurricane, minor variations or uncertainties in the input or intermediate states of an LLM can result in significant and unexpected outputs, including hallucinations.
In Large Language Models (LLMs), the sequence of tokens processed refers to the order in which words or subwords (tokens) are inputted into the model. The model generates predictions based on this sequential information. Here’s a breakdown with examples to illustrate how the sequence of tokens influences the output:
How Token Sequence Works
- Tokenization:
- Before processing text, LLMs break it down into smaller units called tokens. These tokens can be words, subwords, or even characters.
- Sequential Processing:
- The model processes these tokens one by one in the order they appear, using each token to inform the prediction of the next token.
Example 1: Simple Sentence Completion
Consider the prompt: “The cat sat on the”
- Token Sequence: [“The”, “cat”, “sat”, “on”, “the”]
- The model uses this sequence to predict the next token. Common predictions might include “mat”, “floor”, “sofa”, etc.
Example 2: Importance of Token Order
Consider two different prompts:
- “She fed her dog.”
- “Her dog fed she.”
- Token Sequence 1: [“She”, “fed”, “her”, “dog”, “.”]
- Likely continuation: “She fed her dog before leaving for work.”
- Token Sequence 2: [“Her”, “dog”, “fed”, “she”, “.”]
- The second sequence is grammatically incorrect and confusing, leading to unpredictable or nonsensical continuations.
Example 3: Contextual Impact
Consider the sentence: “He went to the bank to deposit some money.”
- Token Sequence: [“He”, “went”, “to”, “the”, “bank”, “to”, “deposit”, “some”, “money”]
- The word “bank” is ambiguous. Based on the context provided by the tokens “deposit some money,” the model understands that “bank” refers to a financial institution.
If the sentence was: “He went to the bank to fish.”
- Token Sequence: [“He”, “went”, “to”, “the”, “bank”, “to”, “fish”]
- Here, the word “bank” is understood as the side of a river or lake, thanks to the context provided by the tokens “to fish.”
Example 4: Generating Complex Outputs
Consider writing a story with the prompt: “Once upon a time, there was a kingdom.”
- Token Sequence: [“Once”, “upon”, “a”, “time”, “,”, “there”, “was”, “a”, “kingdom”]
- The model uses this sequence to generate a continuation that fits the context. Possible continuations might include: “ruled by a wise king” or “where magical creatures lived.”
Summary
The sequence of tokens processed by an LLM is crucial because:
- Contextual Understanding: The meaning and coherence of the generated text depend on the order and context of preceding tokens.
- Predictive Accuracy: Small changes in the token sequence can lead to vastly different predictions, highlighting the sensitivity and complexity of the model’s internal workings.
- Ambiguity Resolution: The context provided by the sequence helps the model resolve ambiguities and generate more accurate and relevant outputs.
Understanding how LLMs process token sequences helps in designing better prompts and interpreting the model’s behavior more effectively.
Here are some examples illustrating how minor changes in the initial prompt can lead to significantly different outputs in a Large Language Model (LLM), demonstrating the butterfly effect in action:
Example 1: Simple Change in a Word
Prompt 1: “The cat sat on the mat.”
- Output: “The cat sat on the mat, purring contentedly.”
Prompt 2: “A cat sat on the mat.”
- Output: “A cat sat on the mat, looking around curiously.”
Here, changing “The” to “A” shifts the focus from a specific cat to any cat, resulting in different descriptive details.
Example 2: Minor Change in Punctuation
Prompt 1: “Let’s eat, grandma.”
- Output: “Let’s eat, grandma. Dinner is ready and the table is set.”
Prompt 2: “Let’s eat grandma.”
- Output: “Let’s eat grandma. She was always a part of our stories and now she will be remembered forever.”
The change in punctuation dramatically alters the meaning of the sentence, leading to vastly different continuations.
Example 3: Synonym Usage
Prompt 1: “He was a brave soldier.”
- Output: “He was a brave soldier who fought valiantly in many battles.”
Prompt 2: “He was a courageous soldier.”
- Output: “He was a courageous soldier, always leading his men with unwavering determination.”
Although “brave” and “courageous” are synonyms, the model’s continuation reflects slightly different nuances in the description.
Example 4: Contextual Clues
Prompt 1: “She went to the bank.”
- Output: “She went to the bank to deposit a large sum of money she had saved.”
Prompt 2: “She went to the river bank.”
- Output: “She went to the river bank to enjoy a quiet afternoon of fishing and relaxation.”
Adding “river” before “bank” provides a different context, leading to a different narrative.
Example 5: Change in Timeframe
Prompt 1: “In the morning, he jogged through the park.”
- Output: “In the morning, he jogged through the park, enjoying the fresh air and the quiet before the city woke up.”
Prompt 2: “At night, he jogged through the park.”
- Output: “At night, he jogged through the park, listening to the sounds of nocturnal animals and feeling the cool breeze.”
Changing the time of day from “morning” to “night” results in different sensory details and atmosphere in the output.
Summary
These examples illustrate how small variations in the initial prompt can lead to different outputs from an LLM. These changes, analogous to the butterfly effect, show how sensitive LLMs are to their inputs. Even minor differences can cascade through the model’s processing, resulting in diverse and sometimes unpredictable outcomes.
The “token prediction chain” refers to how each predicted token influences the next one in a sequence, and small deviations early on can lead to increasingly divergent outputs. Here are some examples demonstrating this concept:
Example 1: Narrative Continuation
Initial Prompt: “Once upon a time, in a quiet village, there was a young girl named Alice.”
- Prediction Chain 1:
- “Alice loved to explore the woods near her home.”
- “She often found interesting plants and animals.”
- “One day, she discovered a hidden path.”
- Prediction Chain 2 (with a small change in the initial prediction):
- “Alice loved to explore the old library in her village.”
- “She often found dusty books and ancient scrolls.”
- “One day, she discovered a mysterious book.”
In this example, the initial prediction of “woods” vs. “library” leads to entirely different story developments.
Example 2: Informational Response
Initial Prompt: “The capital of France is”
- Prediction Chain 1:
- “Paris.”
- “Paris is known for its art, fashion, and culture.”
- “It is home to landmarks like the Eiffel Tower and the Louvre Museum.”
- Prediction Chain 2 (with a small change in the initial prediction):
- “Paris, a city rich in history.”
- “Paris, a city rich in history, has been a major center for finance and politics.”
- “It hosts important institutions like the French government and the UNESCO headquarters.”
The initial prediction “Paris” followed by either “.” or “a city rich in history” steers the information flow in slightly different directions, emphasizing different aspects of Paris.
Example 3: Dialogue Continuation
Initial Prompt: “I think we should go to the beach today.”
- Prediction Chain 1:
- “Sure, the weather is perfect for it.”
- “Let’s pack some snacks and drinks.”
- “We can leave in an hour.”
- Prediction Chain 2 (with a small change in the initial prediction):
- “Maybe, but I’m worried about the weather.”
- “Let’s check the forecast first.”
- “If it’s clear, we can leave in an hour.”
Here, the initial response “Sure” vs. “Maybe” leads to different subsequent dialogue focusing either on preparation or concern about the weather.
Example 4: Instructional Text
Initial Prompt: “To bake a cake, first preheat the oven to”
- Prediction Chain 1:
- “350 degrees Fahrenheit.”
- “Next, mix the flour and sugar in a bowl.”
- “Add eggs, butter, and vanilla extract.”
- Prediction Chain 2 (with a small change in the initial prediction):
- “400 degrees Fahrenheit.”
- “While the oven heats up, mix the ingredients.”
- “Make sure to grease the baking pan.”
Changing the initial temperature from “350 degrees Fahrenheit” to “400 degrees Fahrenheit” affects the subsequent instructions, emphasizing different preparatory steps.
Example 5: Creative Writing
Initial Prompt: “The scientist worked late into the night, trying to solve the mystery of”
- Prediction Chain 1:
- “time travel.”
- “He believed he was close to a breakthrough.”
- “The equations finally made sense, revealing a path to the past.”
- Prediction Chain 2 (with a small change in the initial prediction):
- “the missing artifact.”
- “He had been searching for it for years.”
- “Suddenly, a clue appeared in the old manuscript he was reading.”
The initial choice of “time travel” vs. “the missing artifact” sets the stage for different narratives, focusing either on scientific discovery or an archaeological mystery.
Summary
These examples illustrate how the token prediction chain can lead to divergent outcomes based on small changes early in the sequence. Each token influences the next, and initial deviations can amplify as the sequence progresses, resulting in significantly different outputs. This sensitivity to initial conditions and early predictions highlights the butterfly effect in LLMs.
The “complex interactions” within a Large Language Model (LLM) refer to the intricate and highly interconnected pathways that the model uses to generate predictions. Small changes or perturbations in input can activate different pathways, leading to diverse and sometimes unexpected outputs. Here are some examples to illustrate this:
Example 1: Different Interpretations of Ambiguous Input
Initial Prompt: “She saw the man with the telescope.”
- Interpretation 1 (activated pathway): She used the telescope to see the man.
- Output: “She saw the man with the telescope, observing him from a distance.”
- Interpretation 2 (different pathway): The man she saw had a telescope.
- Output: “She saw the man with the telescope, who seemed to be scanning the horizon.”
The model’s pathway activation can lead to different interpretations of the ambiguous phrase “with the telescope,” resulting in different outputs.
Example 2: Small Change Leading to Different Contextual Understanding
Initial Prompt 1: “He unlocked the door and found the treasure.”
- Complex Interaction Pathway 1:
- Output: “He unlocked the door and found the treasure, glittering gold and jewels in a dusty old chest.”
Initial Prompt 2: “He unlocked the door and found the letter.”
- Complex Interaction Pathway 2:
- Output: “He unlocked the door and found the letter, yellowed with age and sealed with wax.”
A small change in the object found (“treasure” vs. “letter”) activates different pathways in the model, leading to distinct narrative details.
Example 3: Emotional Tone Shifts
Initial Prompt: “She received the news.”
- Complex Interaction Pathway 1 (positive):
- Output: “She received the news with joy, her eyes lighting up as she read the acceptance letter from her dream university.”
- Complex Interaction Pathway 2 (negative):
- Output: “She received the news with dread, her heart sinking as she read about the upcoming layoffs at her company.”
The model’s pathways determine the emotional tone based on subtle cues or the lack thereof, leading to different emotional interpretations.
Example 4: Changes in Setting or Context
Initial Prompt 1: “The conference was held in a modern city.”
- Complex Interaction Pathway 1:
- Output: “The conference was held in a modern city, with skyscrapers lining the streets and advanced technology on display everywhere.”
Initial Prompt 2: “The conference was held in a historical city.”
- Complex Interaction Pathway 2:
- Output: “The conference was held in a historical city, surrounded by ancient architecture and rich cultural heritage.”
A small change in the setting (“modern city” vs. “historical city”) leads to different pathways being activated, resulting in varied descriptions.
Example 5: Shifts in Genre or Style
Initial Prompt 1: “In a galaxy far, far away, a hero emerged.”
- Complex Interaction Pathway 1 (science fiction):
- Output: “In a galaxy far, far away, a hero emerged, wielding a plasma sword and battling intergalactic enemies.”
Initial Prompt 2: “In a small village, a hero emerged.”
- Complex Interaction Pathway 2 (fantasy):
- Output: “In a small village, a hero emerged, wielding a magical staff and defending the villagers from dark forces.”
The initial context provided by “galaxy far, far away” vs. “small village” activates different genre-specific pathways, influencing the style and content of the continuation.
Summary
These examples demonstrate how the complex interactions within an LLM can lead to diverse outputs based on small changes in the input. The intricate pathways within the model can result in different interpretations, emotional tones, settings, and styles, illustrating the butterfly effect’s impact on the model’s predictions.
The concept of “training data gaps” refers to scenarios where the Large Language Model (LLM) encounters inputs or contexts that were not well-represented in its training data. These gaps can lead to the model generating incorrect or nonsensical outputs, which is another manifestation of the butterfly effect. Here are some examples to illustrate this:
Example 1: Niche or Rare Topics
Initial Prompt: “Explain the principles of quantum chromodynamics.”
- If Training Data Is Comprehensive:
- Output: “Quantum chromodynamics (QCD) is a theory within the Standard Model of particle physics that describes the strong interaction between quarks and gluons, which are the fundamental particles that make up protons and neutrons.”
- If Training Data Has Gaps in This Area:
- Output: “Quantum chromodynamics is a method used in quantum computing to organize chromatic data in a dynamic way.”
Here, a gap in the model’s training data on specific scientific topics leads to a significant error in the output.
Example 2: Cultural or Regional Knowledge
Initial Prompt: “Describe the traditional festivals celebrated in Bhutan.”
- If Training Data Is Comprehensive:
- Output: “In Bhutan, traditional festivals called Tshechu are celebrated with masked dances, music, and rituals. These festivals are held in various monasteries and temples throughout the country.”
- If Training Data Has Gaps in This Area:
- Output: “Bhutanese festivals often involve people gathering to share food and stories, similar to common global celebrations.”
A lack of specific cultural knowledge leads to a generic and inaccurate description of Bhutanese festivals.
Example 3: Emerging Technologies
Initial Prompt: “Discuss the applications of neuromorphic computing.”
- If Training Data Is Comprehensive:
- Output: “Neuromorphic computing is used in creating hardware that mimics the neural structure of the human brain, leading to advancements in AI, robotics, and sensory processing systems.”
- If Training Data Has Gaps in This Area:
- Output: “Neuromorphic computing is a field in neuroscience focusing on the therapeutic applications of neural interfaces.”
Training data gaps on cutting-edge technologies result in an incorrect description of the field.
Example 4: Historical Events with Limited Coverage
Initial Prompt: “Explain the significance of the Battle of Adwa.”
- If Training Data Is Comprehensive:
- Output: “The Battle of Adwa, fought in 1896, was a significant event in Ethiopian history where Ethiopian forces defeated Italian invaders, ensuring Ethiopia remained independent during the colonial scramble for Africa.”
- If Training Data Has Gaps in This Area:
- Output: “The Battle of Adwa was a small skirmish in Africa with minimal historical significance.”
A lack of detailed historical data leads to an underestimation of the event’s importance.
Example 5: Lesser-Known Literature
Initial Prompt: “Summarize the plot of ‘The Tale of Genji.'”
- If Training Data Is Comprehensive:
- Output: “‘The Tale of Genji,’ written by Murasaki Shikibu, is a classic Japanese literary work that follows the life and loves of Hikaru Genji, a nobleman in the Heian period. The novel explores themes of romance, political intrigue, and the transient nature of life.”
- If Training Data Has Gaps in This Area:
- Output: “‘The Tale of Genji’ is a story about a warrior named Genji who embarks on a series of epic adventures across ancient Japan.”
Insufficient data on classic literature leads to a mischaracterization of the story’s genre and plot.
Summary
These examples show how training data gaps can cause LLMs to produce incorrect or nonsensical outputs. The butterfly effect plays a role here as small uncertainties or gaps in knowledge can lead to significant deviations in the model’s predictions. This highlights the importance of comprehensive and diverse training data to improve the reliability and accuracy of LLM-generated information.
Improving the reliability of the information generated by Large Language Models (LLMs) involves several strategies aimed at mitigating the effects of the “butterfly effect” and other sources of error. Here are some key approaches:
- Enhanced Training Data Quality:
- Curate Diverse and Comprehensive Datasets: Ensuring the training data covers a wide range of topics and contexts reduces the likelihood of encountering unknown or poorly understood inputs.
- Clean and Validate Data: Removing errors, biases, and irrelevant information from the training data can help the model learn more accurate patterns.
- Refinement of Model Architectures:
- Implement Advanced Architectures: Use state-of-the-art model architectures that are better at capturing context and handling long-range dependencies.
- Regularization Techniques: Apply techniques such as dropout and weight decay to prevent overfitting and improve generalization.
- Prompt Engineering and Context Management:
- Optimize Prompts: Carefully design prompts to provide clear and unambiguous context, reducing the chances of the model misinterpreting the input.
- Use Context Windows: Maintain a context window to provide the model with a consistent and relevant context throughout the generation process.
- Post-Processing and Validation:
- Implement Post-Processing Filters: Use additional algorithms to validate and correct outputs, checking for factual accuracy and coherence.
- Human-in-the-Loop: Incorporate human oversight to review and correct outputs, especially in high-stakes applications.
- Fine-Tuning and Continuous Learning:
- Domain-Specific Fine-Tuning: Fine-tune the model on domain-specific data to improve its accuracy and relevance in particular contexts.
- Incremental Learning: Continuously update the model with new data to keep it current and improve its performance over time.
- Explainability and Transparency:
- Develop Explainable Models: Implement methods to make the model’s decision-making process more transparent, helping to identify and address sources of error.
- Provide User Feedback Mechanisms: Allow users to provide feedback on outputs, which can be used to further refine and improve the model.
By applying these strategies, the reliability of the information generated by LLMs can be significantly enhanced, reducing the occurrence of hallucinations and improving overall accuracy and trustworthiness.
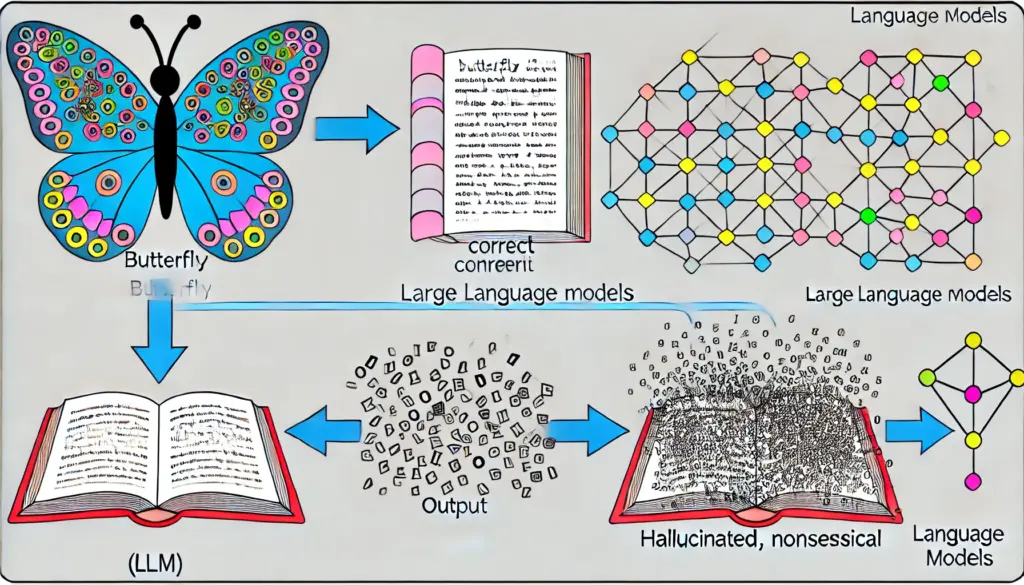