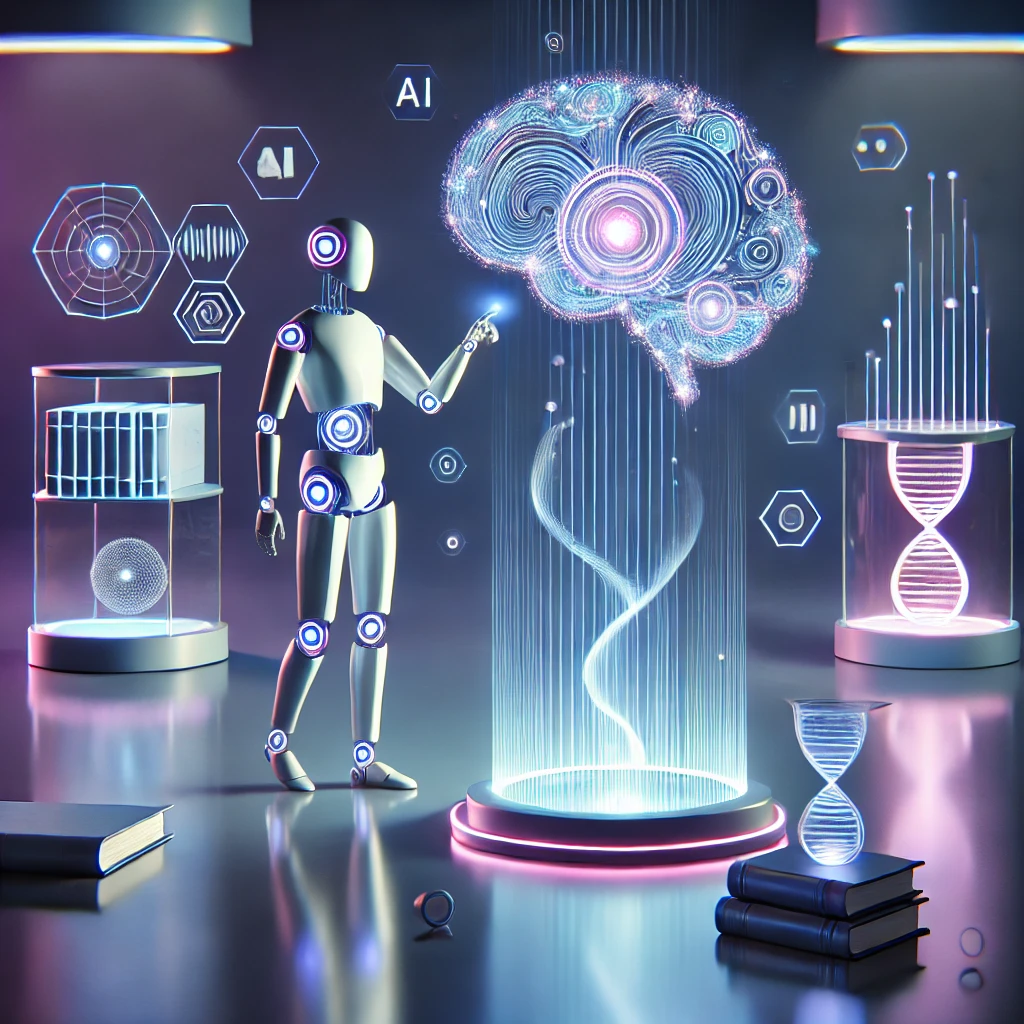
ChatGPT:
How AI Reveals the Human Mind
Extended Analysis of the Language-Brain Connection Through Artificial Intelligence
This long-form deep dive synthesizes the key arguments and insights from a rich dialogue between Nicholas Weiler and neuroscientist Laura Gwilliams on the topic of how large language models (LLMs) like ChatGPT can not only mimic human language, but also help illuminate the inner workings of the human brain. As LLMs become increasingly capable of realistic and coherent language output, researchers are using them to reverse-engineer our own linguistic and cognitive architecture. The discussion provides a fascinating view into current research, philosophical debates, and future ambitions in cognitive neuroscience and artificial intelligence.
1. The Illusion of Human-Like AI
Modern LLMs such as Claude and ChatGPT often appear to exhibit human-like conversational intelligence. Their capabilities elicit amazement, even among scientists, because the systems can engage in persuasive, coherent dialogue. But this is an illusion born from complex word prediction—not understanding or intention. The systems are built upon massive training datasets and predict the most statistically likely next word given a context.
Yet this illusion prompts an intriguing reversal: rather than always reminding ourselves of the differences between AI and humans, what can we learn by treating them as similar?
2. Using AI to Understand Human Language Processing
Laura Gwilliams suggests a profound shift: treat LLMs as cognitive models to better understand human language systems. If these models generate outputs that align with how humans perceive and produce language, perhaps they are also simulating aspects of how the human brain functions. This approach draws a connection between linguistic output and brain activation—especially when models like GPT-2 are used to predict neural responses to language stimuli in human brains.
By comparing neural activations across GPT’s transformer layers to human brain activity from fMRI scans, researchers are gaining insight into how symbolic meaning and phrase-level comprehension arise in the human cortex.
3. Internal Representations and Dimensional Semantics
Traditionally, cognitive scientists studied meaning by asking humans to rate words on dimensions like emotional valence, color relevance, or transportation association. This allows for a multi-dimensional vector space representing word meaning. But this is both labor-intensive and limited by human introspection.
LLMs, on the other hand, convert every word and phrase into numerical vectors through vast training. These vectors encode abstract semantic relationships that can now be used to simulate and predict human brain activity—providing a scalable and more nuanced way of understanding conceptual representation.
4. The Brain as a Prediction Machine—But Not Only That
There is an ongoing debate: is the brain simply a “prediction engine” like an LLM? While Gwilliams acknowledges that human brains engage in significant predictive processing (e.g., anticipating a next word or environmental outcome), she argues that language in humans serves deeper functions—especially social ones. We don’t just speak to transfer data. We speak to connect, comfort, negotiate, joke, and build relationships.
Language’s purpose is not reducible to prediction. Thus, while AI may model part of the brain’s processing ability, it lacks core components of human language use: emotion, intentionality, and social bonding.
5. Lesion Studies in AI and Aphasia in Humans
In a compelling experiment, researchers disabled (or “lesioned”) certain neurons in a language model to mimic the effects of brain damage in humans with aphasia. The resulting AI errors paralleled those seen in stroke survivors—e.g., producing grammatically valid but semantically incoherent sentences. This suggests that LLMs can model not only normal cognitive behavior but also pathological variants, opening a new frontier for neuropsychological research.
The comparison is especially useful because AI models can be probed repeatedly and with precision—unlike human brains, which are inaccessible at the neuron-by-neuron level.
6. LLMs as Digital Model Organisms
In neuroscience, animals are often used as model organisms to understand vision, movement, and other functions. But language is uniquely human. Songbirds and primates show limited overlap, but nothing close to full language capacity.
Gwilliams argues that LLMs are now the first viable digital model organism for language research. These systems can be dissected, tested, and manipulated far beyond what ethics or technology allow for in humans. Experiments include scaling inputs, lesioning nodes, and modifying layers to see how linguistic output changes.
7. Bridging the Training Gap: Text vs. Speech
Despite the alignment between LLMs and the human brain, a glaring discrepancy remains: LLMs learn from curated, clean text data, while humans learn from messy, ambiguous spoken language. Babies acquire language by listening, babbling, and interacting—not by reading dictionaries.
New research efforts, including those in Gwilliams’ lab, aim to build speech-first language models that train directly from audio inputs. These could better simulate human development and capture paralinguistic features such as intonation, emphasis, and emotion—elements that are stripped away when converting speech to text.
8. Reintroducing Emotion and Context
Current voice assistants like Siri or Alexa use a speech-to-text pipeline to handle commands. This approach loses much of the nuance embedded in tone, emotion, and conversational context. By shifting to models that handle raw audio end-to-end, researchers hope to recover this lost depth.
Such models could eventually detect and convey emotional states, offering more human-like interaction. This raises ethical and technical questions about how much emotional sensitivity we want in machines—but it would undoubtedly improve communicative realism.
9. AI’s Lack of Motivation and Social Intent
One of the fundamental differences between humans and AI remains motivation. Human language is deeply tied to needs: to connect, to be heard, to influence. AI models have no agency or desire. They only respond to input with probabilistically generated output.
This distinction matters. It suggests that while AI can simulate aspects of linguistic behavior and even brain activity, it cannot yet replicate the experience of language. Future models might include motivation-like elements, but that introduces philosophical and safety questions about AI autonomy.
10. The Path Forward: Scientific Discovery via Alignment
Gwilliams concludes that the real power of AI as a tool in neuroscience lies in alignment. When LLMs outperform traditional linguistic theories at predicting brain activity, they challenge researchers to figure out why. What hidden features or emergent properties are these models capturing that scientists missed?
By answering that, scientists hope to uncover new cognitive principles, uncover previously invisible neural representations, and redefine theories of meaning, abstraction, and language architecture.
Final Thoughts
This conversation reveals a remarkable convergence of disciplines—AI, neuroscience, linguistics, and philosophy—coming together to decode one of humanity’s most profound capabilities: language. As AI gets better at simulating our speech, we are paradoxically learning more about ourselves—our cognition, our limits, and our deepest needs for connection.
What are large language models (LLMs)?
LLMs are advanced AI systems trained on vast amounts of text data to predict the most likely next word in a sequence. Examples include ChatGPT, Claude, and DeepSeek. Though they lack consciousness or intent, they generate human-like responses and can simulate conversation.
How are LLMs used in neuroscience research?
Neuroscientists use LLMs to model and predict human brain activity during language processing. By comparing AI “neuron” activations to brain imaging data, researchers investigate how the brain represents and comprehends language at various levels—from sounds to meaning.
What is the significance of “lesioning” an AI model?
Lesioning involves disabling specific parts of an AI model to study how its behavior changes. Researchers use this to simulate the effects of brain injuries like aphasia, helping them understand the relationship between specific neural functions and language breakdown.
Can AI help understand abstract meaning in the brain?
Yes. LLMs convert language into high-dimensional numerical representations that align well with brain activity associated with abstract meaning. This helps scientists bridge the gap between raw speech and symbolic comprehension.
Are AI models just prediction engines like the human brain?
LLMs operate primarily through statistical prediction. While human brains also use prediction, they are driven by social, emotional, and intentional needs, making their language use far more complex and nuanced.
What is a “digital model organism”?
A digital model organism is an AI system treated like a lab model (e.g., a mouse) but for human-specific abilities like language. LLMs serve this role, allowing researchers to run controlled experiments on systems capable of language without ethical limitations.
How does training AI on speech differ from training it on text?
Text-based models miss paralinguistic cues like emotion and tone. Speech-trained models aim to learn language in a way that mirrors human development, capturing richer context and emotional nuance lost in the text-to-speech pipeline.
What are “semantic dimensions” in language analysis?
Semantic dimensions are measurable features of words—like color relevance, emotional valence, or category (e.g., transportation). Traditionally rated by humans, LLMs now model these dimensions more efficiently through learned representations.
Why is internal monologue discussed in the article?
Internal monologue illustrates the link between language and thought. However, studies show variability in how people experience this inner voice, challenging the assumption that language is essential for all cognitive processing.
What’s next in the field of AI and language neuroscience?
Researchers aim to build models that learn language like humans—through raw auditory experience—and decode how LLMs encode meaning. This could redefine linguistic theory, improve human-machine interaction, and offer insights into cognition and language disorders.