As generative artificial intelligence (GenAI) continues to push the boundaries of creative expression and problem-solving, a growing concern looms: the emergence of hallucinations. An example of a hallucination is when GenAI confidently fabricates content that doesn’t align with reality. With their ability to generate astonishingly realistic images, texts, music and more, GenAI models have raised both excitement and alarm in equal measure.
The manifestation of hallucinations has garnered significant attention due to its potential implications. Authors have been credited with nonexistent articles and fake legal cases have been cited to name just two recent examples. Understanding the underlying causes of these hallucinations is crucial to developing effective strategies for prevention.
let’s ask ChatGPT‘S opinion:
Question: explain why Hallucinations happen with Generative A.I..
ChatGPT: Hallucinations in chatbots refer to instances where the AI generates incorrect, irrelevant, or nonsensical responses. This can occur for several reasons:
- Training Data Limitations: If the AI’s training data lacks certain information or contains biases, the model might generate responses that don’t align with reality or user expectations.
- Model Complexity: AI models, especially those based on deep learning, are complex and sometimes make errors in understanding or generating contextually appropriate responses.
- Overgeneralization: AI might overgeneralize from the patterns it has learned, leading to responses that are factually incorrect or irrelevant to the specific context of the conversation.
- Input Misinterpretation: Sometimes, the AI might misinterpret the user’s input due to ambiguity, leading to an off-topic or incorrect response.
- Lack of Real-World Understanding: AI doesn’t have real-world experience or consciousness. It operates purely on patterns learned from data, so it can’t always grasp the nuances or factual accuracy like a human.
- Statistical Parroting: AI models can sometimes repeat phrases or ideas seen in training data without understanding their accuracy or appropriateness.
In summary, hallucinations in chatbots arise from the limitations of their training data, the complexity of language, and the inherent challenges in creating AI models that can accurately mimic human conversation and understanding.
Question: How an average user be aware of the possibility of incorrect information?
ChatGPT: An average user can be aware of the possibility of incorrect information from a chatbot by considering the following points:
- Understand the Nature of AI: Recognize that AI chatbots are based on machine learning models, which means they generate responses based on patterns in their training data and don’t have real-world knowledge or consciousness.
- Question Plausibility: If a response seems unusual, overly complex, or too simplistic, it’s worth questioning its accuracy. Cross-referencing with reliable sources can be helpful.
- Be Skeptical of Facts and Figures: Treat specific facts, figures, or historical data provided by a chatbot with caution, especially if they are not commonly known or are very detailed.
- Look for Consistency: Inconsistent responses or answers that don’t align well with previous information in the conversation might indicate incorrect information.
- Awareness of Training Limitations: Recognize that the chatbot’s training may have limitations and biases, which can lead to incorrect or skewed information.
- Use Reliable Sources for Verification: For important or critical information, always verify with a reliable and authoritative source.
- Notice Language and Context Issues: If the response seems out of context or uses language awkwardly, it may be a sign that the chatbot has misunderstood the query or is ‘hallucinating’.
By maintaining a critical and questioning approach, users can better navigate the limitations of chatbot responses and reduce the risk of being misled by incorrect information.
Question: What are the percentage of possible incorrect output by generative A.I.?
ChatGPT: Assigning a specific percentage to the potential incorrect output of generative AI is quite challenging, as it varies significantly based on several factors:
- Type of AI Application: Different applications like text generation, image creation, or data analysis have varying levels of accuracy. Text-based AI, for instance, may have different error rates depending on the complexity of the task.
- Complexity of Task or Query: Simpler, well-defined tasks often yield more accurate results. More complex, ambiguous, or novel queries increase the likelihood of errors.
- Quality and Diversity of Training Data: The broader and more diverse the training dataset, the more accurately the AI can respond to a wide range of inputs. Limited or biased data can lead to a higher rate of incorrect outputs.
- Model Design and Algorithm: The sophistication and design of the underlying AI model play a significant role. More advanced models may have lower error rates, but no model is error-free.
- Domain-Specific AI: AI systems trained for specific domains (like medical, legal, or technical) might be more accurate within their area of expertise and less accurate outside it.
- Contextual Understanding: AI’s capability to understand and maintain context in a conversation or task affects its accuracy. Misinterpretation of context can lead to incorrect outputs.
Due to these factors, there’s no one-size-fits-all percentage that can be universally applied to generative AI’s accuracy. For certain tasks, the accuracy might be very high, while for others, particularly those involving nuanced understanding or creativity, the likelihood of incorrect outputs increases. Users should always consider AI-generated content critically, especially when accuracy is paramount.
Here is how Dalle3 views the Hallucination:
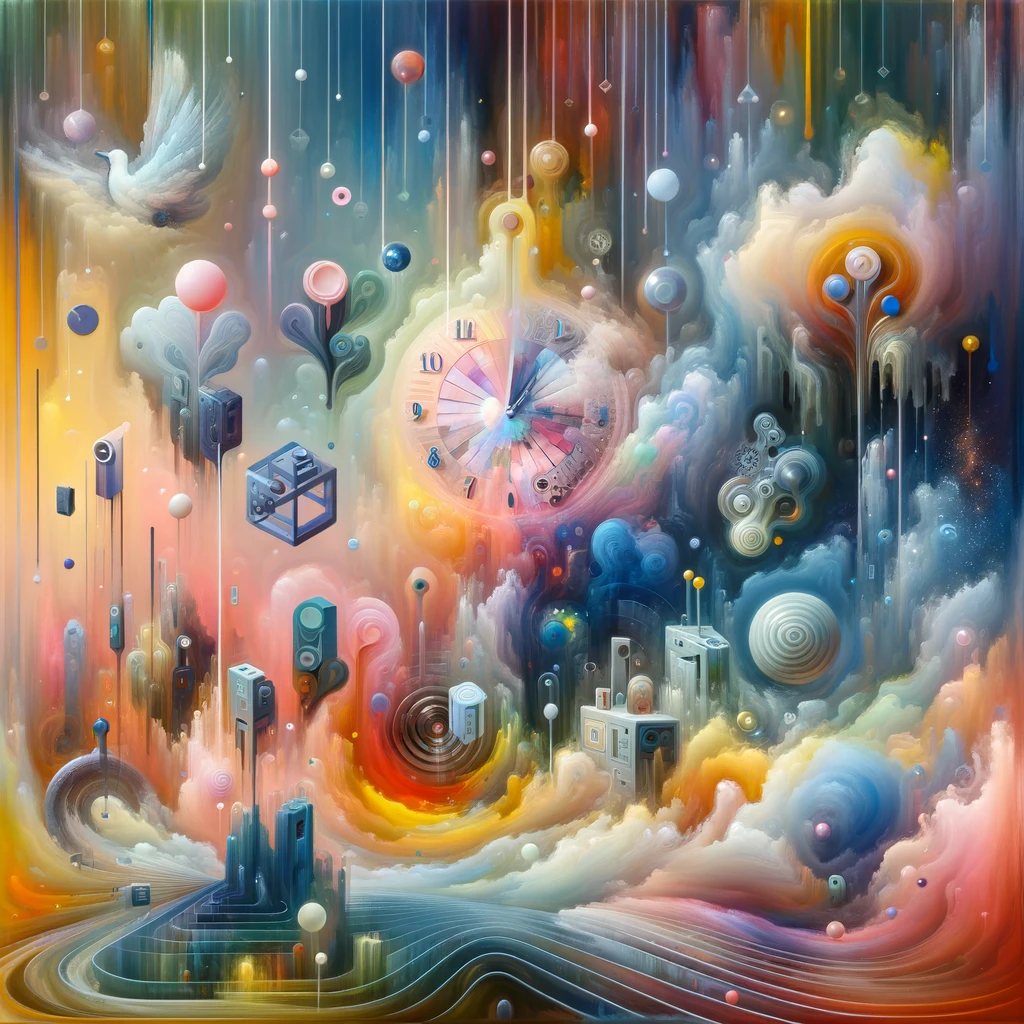