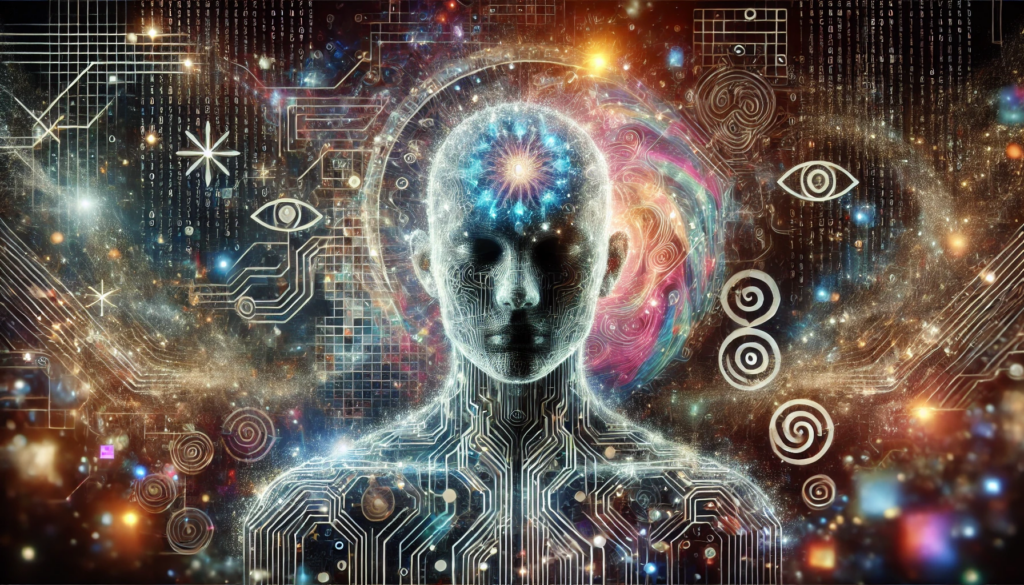
ChatGPT:
🌌 How Hallucinatory A.I. Helps Science Dream Up Big Breakthroughs
Artificial Intelligence (A.I.) has long faced criticism for generating hallucinations—outputs that seem plausible but are factually false. While these errors can create problems in areas like legal cases and medical diagnoses, scientists are increasingly using A.I.’s hallucinatory tendencies as a creative tool. This expanded summary explores how A.I. hallucinations are reshaping scientific discovery, enabling breakthroughs in medicine, biology, meteorology, and technology.
🧠 Introduction: The Role of A.I. Hallucinations
Artificial intelligence has entered the scientific arena as more than a tool for data analysis—it has become a creative partner. Often dismissed for generating hallucinations, A.I.’s ability to propose imaginative, unexpected ideas is proving invaluable for hypothesis testing, idea generation, and experimentation.
A.I.’s “hallucinations” are outputs that mimic plausible realities but are unverified or factually incorrect. In science, this unpredictability mirrors the trial-and-error methods of traditional discovery. By presenting unusual patterns, A.I. helps researchers uncover new drugs, develop advanced medical devices, and design synthetic proteins never before seen in nature.
🔬 A.I. in Protein Engineering and Nobel Prize Research
One of the most celebrated examples of A.I.‘s impact is David Baker’s work at the University of Washington, which won the 2024 Nobel Prize in Chemistry. Baker’s team used A.I. to design proteins from scratch—proteins that did not exist in nature.
Key Achievements:
• Designed 10 million proteins with novel structures.
• Patented treatments for cancer and viral infections.
• Founded 20 biotech companies based on A.I.-designed proteins.
A.I. enabled Baker’s lab to imagine and test thousands of protein designs quickly, bypassing the slow processes of natural evolution. One example is a treatment that blocks cancer pathways by reshaping protein interactions.
Baker described his work as “de novo protein design,” meaning the proteins were built entirely from the ground up rather than modifying existing natural templates. His success highlights how A.I. hallucinations act as a starting point for breakthroughs.
💊 Drug Discovery and Medical Innovations
A.I. hallucinations have accelerated drug discovery by generating virtual molecules and chemical structures that can be tested in labs.
Key Examples:
• Antibiotic Discovery: MIT researchers used A.I. to find new antibiotics by imagining novel molecular forms.
• Medical Devices: An A.I.-designed catheter lined with sawtooth-like structures reduces bacterial infections, preventing millions of urinary tract infections annually.
• Cancer Treatments: A.I.-generated molecular models are improving drug targeting and immune responses.
In these cases, A.I. significantly shortens research timelines from years to days, allowing scientists to test more ideas and identify solutions faster.
🌍 Weather Forecasting and Meteorology
Meteorologists are leveraging A.I. hallucinations to create multiple probabilistic weather models. Instead of relying on a single forecast, these models simulate thousands of variations, revealing subtle factors that drive extreme weather events such as heat waves, hurricanes, and droughts.
By analyzing these “hallucinated” scenarios, scientists can better predict natural disasters and plan for mitigation strategies.
📊 Reframing Hallucinations as Creative Probability Models
Although the term “hallucination” carries negative connotations, some researchers prefer to view A.I. outputs as “probability distributions” or creative suggestions grounded in physical principles. Unlike chatbots, which rely on language patterns, scientific A.I. tools are rooted in mathematical and physical laws, making their outputs less random and more testable.
This reframing has helped A.I. gain credibility as a tool for hypothesis testing rather than mere speculation.
🚀 The Future: Diffusion Models and Next-Generation A.I.
Scientists like Baker are moving beyond traditional hallucinations to diffusion models—A.I. techniques used in tools like DALL-E for generating images. These models are faster and more accurate in predicting novel protein structures and chemical compositions.
Diffusion models represent the next evolution of A.I. creativity, combining randomness with refinement to generate designs that are closer to real-world applications.
Emerging Innovations:
• Energy Harvesting Proteins: Proteins that capture and store solar energy for sustainable technologies.
• Eco-Friendly Catalysts: Enzymes that could clean industrial waste and reduce carbon emissions.
• Custom Medical Therapies: Tailored treatments designed through A.I. simulations.
🧬 Challenges and Ethical Considerations
Despite their potential, A.I. hallucinations raise ethical and practical concerns:
1. Accuracy Risks: Hallucinations may introduce false data, requiring thorough testing to separate valid hypotheses from errors.
2. Communication Issues: Scientists worry the term “hallucination” undermines credibility, preferring terms like “imaginative predictions.”
3. Regulatory Oversight: Governments, including the U.S., are working on policies to regulate A.I. outputs in research.
🌟 The Impact on Scientific Progress
In recent years, critics have argued that scientific breakthroughs have slowed. However, A.I.’s ability to generate rapid, imaginative outputs is revitalizing innovation. Scientists now have tools to test bold hypotheses faster, leading to new therapies, technologies, and sustainable solutions.
Potential Outcomes:
• More personalized medicine.
• Green technologies powered by synthetic biology.
• Faster responses to pandemics and natural disasters.
🔑 Conclusion: A Glimpse into the Future
A.I. hallucinations, once seen as flaws, are becoming indispensable in science. They have already contributed to Nobel Prize-winning research, novel antibiotics, and groundbreaking medical devices. The combination of A.I.’s imaginative capacity and human oversight is accelerating the scientific method, turning years of work into weeks.
While ethical and practical challenges remain, researchers see A.I. as the key to solving humanity’s biggest challenges, from disease eradication to climate change mitigation.
Experts like David Baker and Anima Anandkumar envision a world where A.I.-powered discoveries unlock new dimensions of understanding life itself. As A.I. continues to evolve, it may redefine the limits of human imagination and creativity, making the impossible achievable.
📚 Key Takeaways
• A.I. hallucinations serve as creative sparks for new scientific ideas.
• Nobel-winning research has already leveraged A.I. for groundbreaking discoveries.
• Fields like medicine, weather forecasting, and sustainability benefit from A.I.’s ability to simulate scenarios rapidly.
• Diffusion models represent the next stage of A.I. evolution, offering more refined predictions.
• Ethical considerations, including accuracy and regulation, must be addressed as A.I. adoption grows.
Diffusion models are a cutting-edge class of artificial intelligence (A.I.) systems used to generate realistic and high-quality data, such as images, proteins, or molecular structures. They represent the next stage of A.I. because of their accuracy, creativity, and versatility in generating novel and meaningful outputs, particularly in scientific research and design.
🔍 What Are Diffusion Models?
Diffusion models work by simulating a process similar to reverse diffusion—the way particles spread out in nature. They start with random noise and gradually transform it into structured and meaningful patterns by removing noise step-by-step.
1. Training Phase: The model learns patterns by repeatedly adding noise to data and then learning how to reverse the process.
2. Generation Phase: The model starts with random noise and progressively refines it into a coherent, realistic output based on learned patterns.
For example:
• In image generation, diffusion models start with a noisy image and refine it into a detailed picture.
• In protein design, they start with random sequences and transform them into biologically viable structures.
🚀 Why Are Diffusion Models the Next Stage of A.I.?
1. Higher Accuracy
Diffusion models produce outputs that closely align with physical and biological realities. Unlike earlier generative models that created random variations, diffusion models generate highly accurate predictions, making them ideal for applications like drug discovery and protein design.
2. Improved Creativity
They allow A.I. to explore a vast range of possibilities, testing hypothetical designs and variations that humans might overlook. For instance, David Baker’s team used diffusion models to create completely novel proteins with unprecedented structures, advancing biotechnology.
3. Versatility Across Fields
Diffusion models can be applied to:
• Medicine: Designing new drugs and medical devices.
• Biology: Creating new protein structures for treating diseases.
• Art and Design: Generating realistic images and art styles.
• Robotics: Improving mechanical designs through simulated testing.
4. Faster Processing and Scaling
They are significantly faster than older generative models, allowing researchers to simulate and test thousands of ideas quickly. For example, a drug development process that once took years can now take weeks.
5. Realistic Outputs
Unlike traditional A.I. hallucinations, which produce outputs disconnected from reality, diffusion models create results grounded in data patterns. This makes them more reliable for scientific and industrial applications.
6. Better Handling of Complex Data
Diffusion models excel at working with complex, high-dimensional data, such as protein structures and 3D molecular models, providing intricate and highly accurate designs that were previously impossible.
🌟 Examples of Applications
1. Drug Discovery
Diffusion models generate chemical compounds, identify potential drugs, and simulate how they interact with biological targets.
2. Protein Engineering
They design proteins with desired properties, enabling treatments for diseases like cancer or viral infections.
3. Medical Imaging
Diffusion models refine blurry medical images, improving MRI and CT scan interpretations.
4. Art and Media
They create high-quality images and videos, powering tools like DALL-E and Sora for artistic and creative work.
5. Weather Forecasting
Simulating atmospheric patterns, diffusion models predict extreme weather events with higher precision.
🔑 Conclusion
Diffusion models mark a paradigm shift in artificial intelligence by combining creativity and accuracy. Their ability to refine random inputs into coherent, meaningful outputs has unlocked possibilities in drug design, medical devices, and weather prediction. As A.I. continues to evolve, diffusion models are expected to drive the next wave of breakthroughs in science, medicine, and engineering, establishing a new foundation for discovery and innovation.
Q&A
Q: What are A.I. hallucinations, and why are they controversial?
A: A.I. hallucinations are outputs that seem plausible but are factually incorrect. While often criticized for generating false information, scientists use them as creative tools to generate novel ideas and hypotheses.
Q: How have A.I. hallucinations impacted scientific research?
A: They have accelerated drug discovery, protein design, and medical device development, enabling breakthroughs like Nobel Prize-winning protein engineering and new antibiotics.
Q: What is an example of a medical device designed using A.I.?
A: Researchers developed a catheter with sawtooth-shaped walls to reduce bacterial infections, demonstrating the practical application of A.I.-generated designs.
Q: How did A.I. contribute to Nobel Prize-winning research?
A: David Baker’s team used A.I. to design 10 million synthetic proteins, creating medical treatments and innovative biotech applications, earning recognition for chemistry advancements.
Q: Why do some scientists avoid the term “hallucinations”?
A: The term implies errors, whereas many outputs are useful, probabilistic predictions. Scientists prefer terms like “imaginative models” to highlight their potential for discovery.
Q: How does A.I. speed up drug discovery?
A: A.I. generates and tests molecular designs in days or weeks, replacing years of manual research and enabling rapid identification of potential treatments.
Q: What is diffusion modeling, and how does it improve A.I. outputs?
A: Diffusion models refine A.I. creativity by producing more accurate and realistic designs, outperforming earlier methods in speed and reliability.
Q: Can A.I. hallucinations be trusted for critical applications?
A: They must undergo rigorous testing and validation to separate valid hypotheses from errors, ensuring reliability in real-world use.
Q: How is A.I. helping meteorology and weather prediction?
A: A.I. simulates thousands of weather scenarios, improving forecasts for extreme events like hurricanes and heat waves.
Q: What are the future possibilities of A.I. in science?
A: A.I. could lead to advances in sustainable energy, personalized medicine, and solutions for global challenges like climate change and disease prevention.
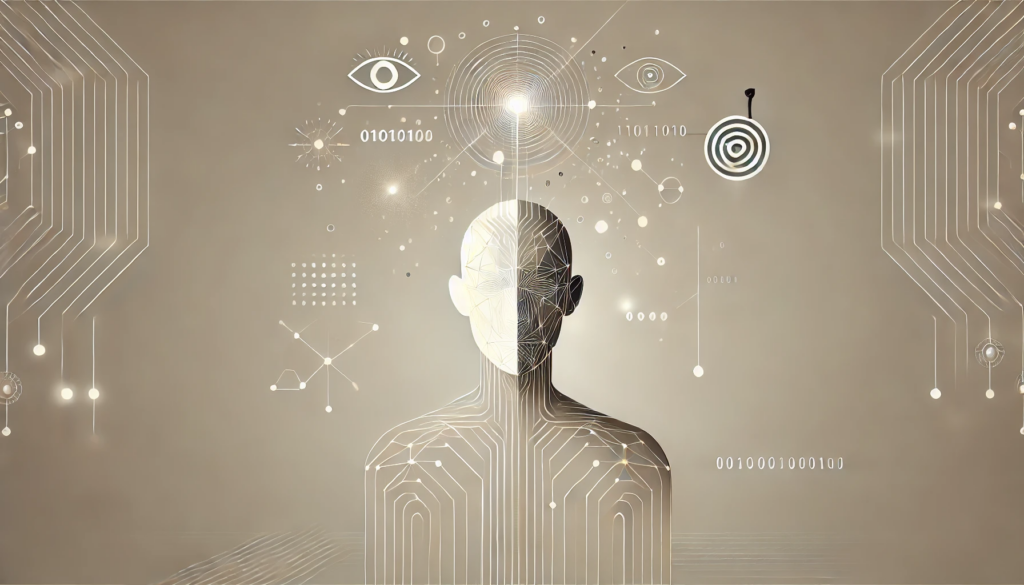