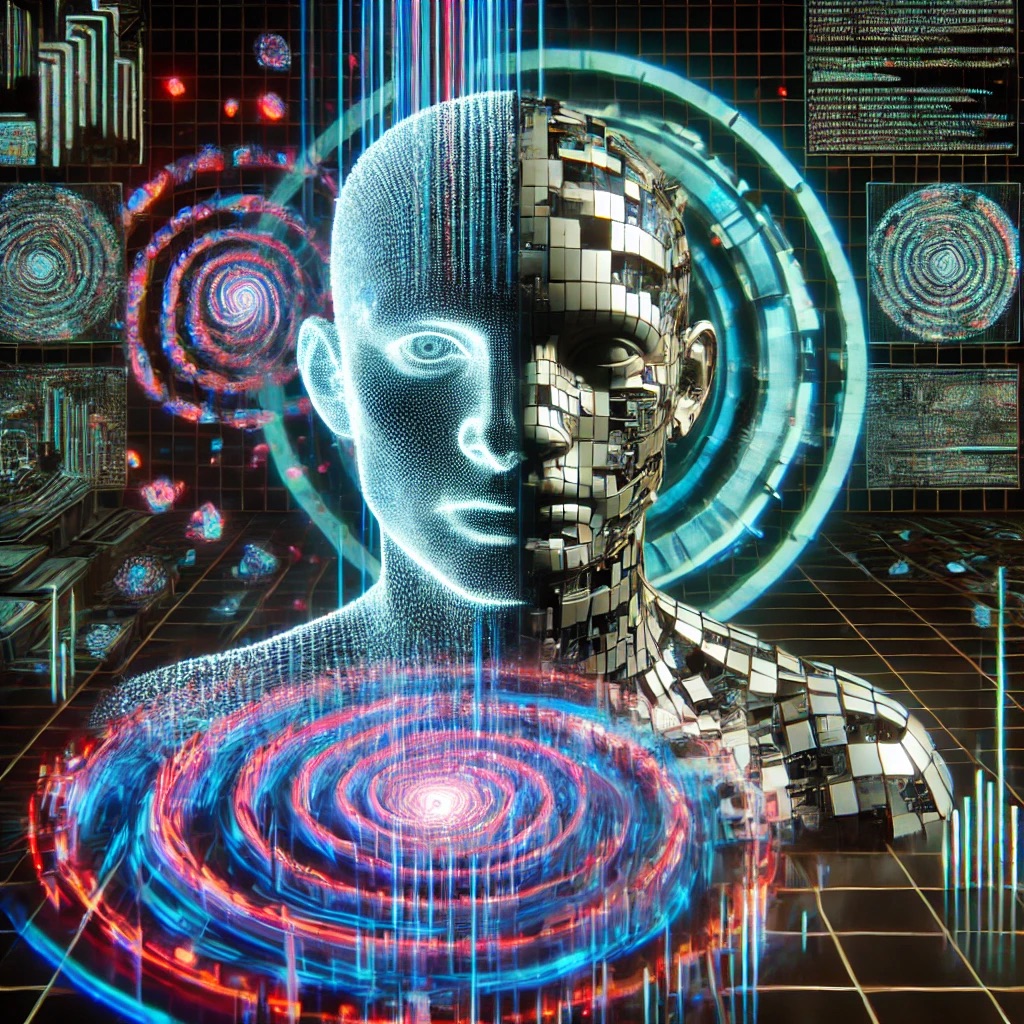
ChatGPT:
🧠 When A.I.’s Output Is a Threat to A.I. Itself
As artificial intelligence continues to evolve, a new and pressing challenge has emerged: the risk of A.I. systems degrading as they train on data generated by other A.I.s. This article delves into the phenomenon where A.I. systems ingest their own outputs, leading to a dangerous feedback loop that could undermine their accuracy, diversity, and overall utility. The article examines the causes, implications, and potential solutions to this issue, emphasizing the need for high-quality, human-generated data to sustain A.I. development.
🌐 The Proliferation of A.I.-Generated Content
A.I.-generated content is becoming ubiquitous on the internet. Companies like OpenAI are producing billions of words daily, a significant portion of which likely ends up online. This content spans various formats, from text and images to more complex outputs like videos and sound. A.I.-generated text might appear in unexpected places, such as product reviews, social media posts, or even news articles. For instance, NewsGuard identified over a thousand websites churning out A.I.-generated news content, which is often riddled with errors.
The issue is compounded by the difficulty in detecting A.I.-generated content. Current detection methods are far from foolproof, meaning that much of this content blends seamlessly into the vast sea of information online. This makes it increasingly challenging to discern real from fake, posing a threat not only to human understanding but also to the A.I. systems that rely on this data.
🔄 The Feedback Loop: A.I. Training on A.I.
A significant concern arises when A.I. systems begin to train on data that has been generated by other A.I. systems. As these models ingest their own output, they risk creating a feedback loop where the quality of the A.I. deteriorates over time. This process, known as “model collapse,” occurs because A.I.-generated data often lacks the nuance and diversity of human-generated data. When an A.I. model repeatedly trains on synthetic data, its output becomes less diverse and less accurate, drifting away from the original data it was meant to emulate.
📉 Model Collapse in Action
Model collapse is a gradual process but can have significant implications. A study published in the journal Nature illustrated this phenomenon using a simple example: an A.I. model trained to recognize handwritten digits. Initially, the model performed well, but as it was repeatedly trained on its own output, the quality and diversity of its results declined. After several generations, the model’s output became blurry, less distinct, and ultimately converged into a uniform, indistinguishable form.
This phenomenon isn’t limited to simple tasks like digit recognition. The article highlights how more complex A.I. applications, such as medical chatbots or educational tutors, could also suffer. For example, a medical chatbot might provide less accurate diagnoses if it has been trained on a narrowed spectrum of A.I.-generated medical information. Similarly, an A.I. history tutor might struggle to distinguish between fact and fiction if its training data includes A.I.-generated propaganda.
🎨 Image Generation and the Risk of Distortion
The problem extends beyond text to include image generation. Researchers at Rice University studied how A.I. models that generate images deteriorate when repeatedly trained on their own outputs. Over time, glitches and distortions accumulate in the A.I.’s output, leading to degraded image quality, such as warped facial features or distorted patterns.
One key example given in the article is the potential overrepresentation of A.I.-generated images in certain artistic styles, like van Gogh’s. If these synthetic images outnumber real photographs in the training data, the A.I. model may start to produce increasingly distorted representations, further straying from the original artistic intent.
📊 Why Model Collapse Happens
Model collapse occurs because A.I.-generated data is often an imperfect substitute for real, human-generated content. A.I. models are trained on statistical distributions of data, predicting the most probable outputs based on their training. When trained on human data, these models produce a wide range of possible outputs, reflecting the diversity and complexity of real-world data.
However, when A.I. is trained on its own outputs, the range of possible outcomes narrows. The statistical distribution of the model’s output becomes taller and narrower, indicating a smaller range of probable results. This leads to a reduction in the diversity of outputs, with the rare and unusual outcomes — the “tails” of the distribution curve — fading away.
As the process continues, the model’s outputs become more uniform and less varied, eventually collapsing into a single, repetitive form. This is the essence of model collapse: the gradual erosion of diversity and accuracy in A.I. output.
💥 Implications for the Future of A.I.
The article emphasizes that while model collapse doesn’t mean the end of generative A.I., it does pose significant challenges for the future. The companies developing these systems are aware of the problem and are actively working to address it. However, the growing prevalence of A.I.-generated content on the internet could make it harder for new A.I. models to be trained effectively.
💰 Economic and Environmental Costs
One of the major implications of this issue is the increased cost and resource consumption required to train A.I. models. As A.I.-generated content contaminates training data, more computational power is needed to maintain the quality of A.I. systems. This translates into higher energy consumption and financial costs, which could make A.I. development less sustainable in the long term.
⚖️ Bias and Diversity
Another significant concern is the potential amplification of biases in A.I. models. As model collapse occurs, the A.I. system’s output becomes less diverse, which can exacerbate existing biases in the data. This is particularly problematic when it comes to underrepresented groups, as the A.I. may increasingly marginalize these perspectives.
For example, if an A.I. language model is trained primarily on A.I.-generated text, it might lose linguistic diversity, producing sentences that are less varied in structure and vocabulary. This loss of diversity can have far-reaching consequences, from reinforcing stereotypes to erasing minority voices from the digital landscape.
🛠 Solutions and Mitigations
To combat the risks of model collapse, the article suggests several potential solutions.
🌟 Importance of High-Quality Data
The most critical solution is ensuring that A.I. systems are trained on high-quality, human-generated data. While this may require significant investment, the article argues that it’s essential for maintaining the long-term viability of A.I. technologies. Companies like OpenAI and Google have already begun to strike deals with publishers and other content creators to secure access to high-quality data.
💧 Detecting and Managing Synthetic Data
Another approach is improving methods for detecting A.I.-generated content. Google and OpenAI are working on watermarking tools to help identify synthetic images and text. However, watermarking text is particularly challenging, as these markers can be easily lost or altered, especially when content is translated into other languages.
📊 Controlled Use of Synthetic Data
The article also notes that in certain contexts, synthetic data can be beneficial for A.I. training. For example, when larger A.I. models are used to generate training data for smaller models, or when the data involves verifiable outcomes like mathematical solutions or game strategies. Additionally, human-curated synthetic data can help alleviate some of the problems associated with model collapse, as it ensures that the data remains relevant and accurate.
🚀 The Path Forward
As the article concludes, there’s no substitute for high-quality, human-generated data in training A.I. models. The future of A.I. depends on the careful management of training data, the development of better detection methods for synthetic content, and the recognition of the limitations and risks associated with A.I.-generated data.
🌍 The Bigger Picture
In the broader context, this challenge highlights the delicate balance required in A.I. development. As A.I. systems become more integrated into daily life, their reliability, accuracy, and diversity become increasingly crucial. The potential for model collapse underscores the need for ongoing research, innovation, and regulation to ensure that A.I. continues to serve society effectively and ethically.
In summary, the article warns that while A.I. has the potential to revolutionize numerous fields, the unchecked proliferation of A.I.-generated data could undermine these advances. The key to sustainable A.I. development lies in maintaining a robust foundation of human-generated content and in developing technologies that can effectively manage and mitigate the risks of synthetic data.
📝 Summary:
- Proliferation of A.I.-Generated Content: The internet is flooded with A.I.-generated text and images, which are difficult to distinguish from real content.
- Feedback Loop Risk: A.I. systems risk deteriorating as they train on their own outputs, creating a dangerous feedback loop that degrades performance over time.
- Model Collapse: Repeated training on A.I.-generated data can lead to “model collapse,” where the output becomes less diverse and accurate, drifting away from reality.
- Image Generation Issues: Similar problems occur with A.I.-generated images, leading to distorted outputs as the system trains on its own creations.
- Detection Challenges: Current methods to detect A.I.-generated content are inadequate, making it difficult to manage the proliferation of synthetic data.
- Economic and Environmental Impact: As A.I.-generated content contaminates training data, more computational power and resources are needed, increasing costs.
- Bias Amplification: Model collapse can exacerbate biases in A.I. systems, leading to less diverse and more homogeneous outputs.
- Importance of High-Quality Data: Ensuring A.I. systems are trained on human-generated data is crucial to prevent model collapse and maintain A.I. quality.
- Solutions for Managing Synthetic Data: Improved detection tools.
Q&A
What is model collapse in A.I.?
Model collapse is a phenomenon where an A.I. system’s output quality and diversity degrade over time as it is repeatedly trained on its own generated data. This feedback loop leads to less accurate, less diverse, and increasingly homogeneous outputs.
How does model collapse occur?
Model collapse occurs when an A.I. model starts using its own outputs as part of its training data. Over successive generations, this causes the model to rely more on its own degraded data, leading to a narrowing of output diversity and a decline in accuracy.
Why is model collapse a concern?
Model collapse is concerning because it can undermine the effectiveness and reliability of A.I. systems. As models produce lower-quality outputs, they become less useful for tasks that require accuracy and diversity, such as medical diagnostics or content generation.
Can model collapse be prevented?
Model collapse can be mitigated by ensuring that A.I. models are trained on high-quality, human-generated data. Limiting the use of A.I.-generated data in training and using detection tools to identify synthetic data can also help prevent model collapse.
What are the consequences of model collapse in practical applications?
In practical applications, model collapse can lead to A.I. systems producing less accurate and less reliable outputs. This could negatively impact industries that rely on A.I., such as healthcare, education, and content creation, where accuracy and diversity are crucial.
How does model collapse affect the diversity of A.I. outputs?
As model collapse progresses, the statistical distribution of A.I. outputs becomes narrower, leading to a loss of diversity. This means that the A.I. system produces fewer unique or rare outputs, which can result in a homogenization of content and a loss of nuance.
What role does synthetic data play in model collapse?
Synthetic data, which is generated by A.I. systems, often lacks the complexity and nuance of real-world data. When models are trained on synthetic data, the quality of their outputs deteriorates, contributing to model collapse.
Are there any benefits to using synthetic data in A.I. training?
While synthetic data can contribute to model collapse, it can be useful in specific contexts, such as when training smaller models using data from larger models or when the output can be verified, like in solving math problems or playing strategy games.
How can human intervention help prevent model collapse?
Human intervention, such as curating synthetic data or selecting the best A.I. outputs for training, can help maintain the quality and diversity of A.I. systems. This approach ensures that the training data remains relevant and accurate, reducing the risk of model collapse.
What does the future hold for A.I. in light of model collapse?
To avoid the pitfalls of model collapse, A.I. developers must focus on maintaining a robust foundation of high-quality, human-generated data. Continuous innovation in detection methods and the careful management of synthetic data will be essential for the sustainable growth of A.I. technologies.
The theory of “model collapse” refers to a phenomenon in artificial intelligence (A.I.) where the quality and diversity of an A.I. model’s output degrade over time due to repeated training on its own generated data. This process occurs because A.I. systems are often trained on large datasets to learn patterns and generate predictions or outputs. However, when these models start using their own outputs as part of the training data, they can enter a feedback loop that causes them to deviate from their original accuracy and diversity.
Key Aspects of Model Collapse:
- Feedback Loop:
- Model collapse begins when an A.I. model is trained not just on human-generated data, but also on data it or other A.I. systems have generated. As this process continues, the model begins to ingest more of its own output, creating a feedback loop.
- Over successive generations of training, this feedback loop causes the model to increasingly rely on its own, often lower-quality, outputs.
- Degradation of Output Quality:
- As the model repeatedly trains on its own data, the outputs tend to become less accurate and more prone to errors. For example, an A.I. trained on its own distorted images will start producing increasingly distorted or incorrect images.
- In the case of language models, this might result in text that is less coherent, more repetitive, and less informative.
- Loss of Diversity:
- The range of outputs produced by the model narrows over time, meaning that the model generates a smaller variety of responses or outputs.
- The statistical distribution of the model’s output becomes “taller and narrower,” meaning the model produces more of the same, with rare or unique outputs becoming increasingly uncommon.
- Convergence to Homogeneity:
- If unchecked, this process can lead to a situation where the model’s outputs become almost identical, losing the variability and richness necessary for complex and nuanced tasks.
- In the extreme case, the model might collapse entirely, producing uniform and unhelpful outputs—essentially “collapsing” in its ability to function effectively.
Causes and Consequences:
- Poor Substitution of Synthetic Data: A.I.-generated data often lacks the nuance and complexity of real-world, human-generated data. When models start relying heavily on this synthetic data, the quality of their learning deteriorates.
- Amplification of Biases: Because model collapse often involves a narrowing of the model’s output, existing biases in the data can become amplified. This is particularly problematic when A.I. systems are applied to tasks that require fairness and diversity.
- Practical Implications: Model collapse can affect various applications of A.I., from medical diagnostics to content generation, leading to less reliable, accurate, and diverse outputs. This could undermine the trust and effectiveness of A.I. systems in real-world scenarios.
Prevention and Mitigation:
- Human-Curated Data: One of the key ways to prevent model collapse is to ensure that A.I. models continue to be trained on high-quality, human-generated data. This helps maintain the diversity and accuracy of the model’s outputs.
- Detection and Control: Developing better tools to detect when A.I. is being trained on its own outputs and controlling the amount of synthetic data used in training can also help mitigate the risks associated with model collapse.
In summary, model collapse is a critical issue in the field of A.I. that highlights the importance of maintaining a robust and diverse training dataset to ensure that A.I. systems remain effective and reliable over time.
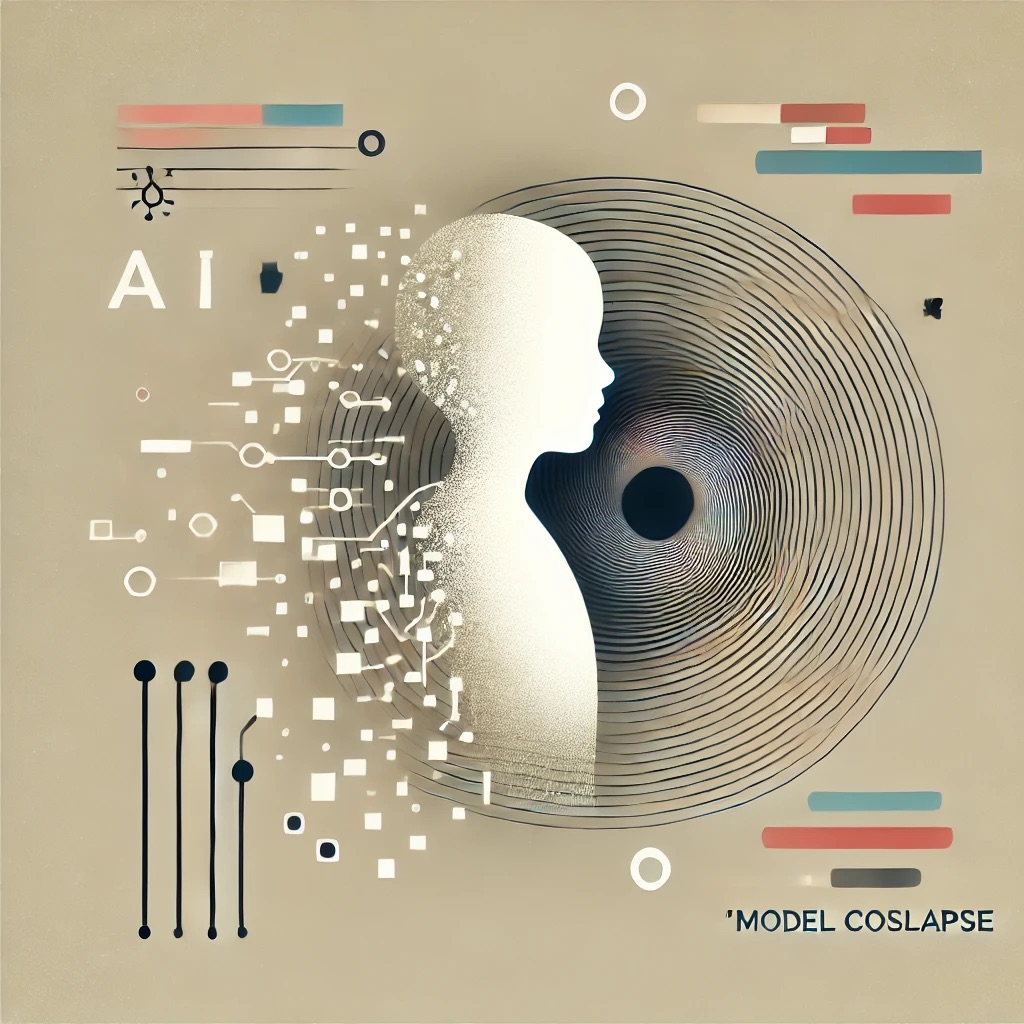