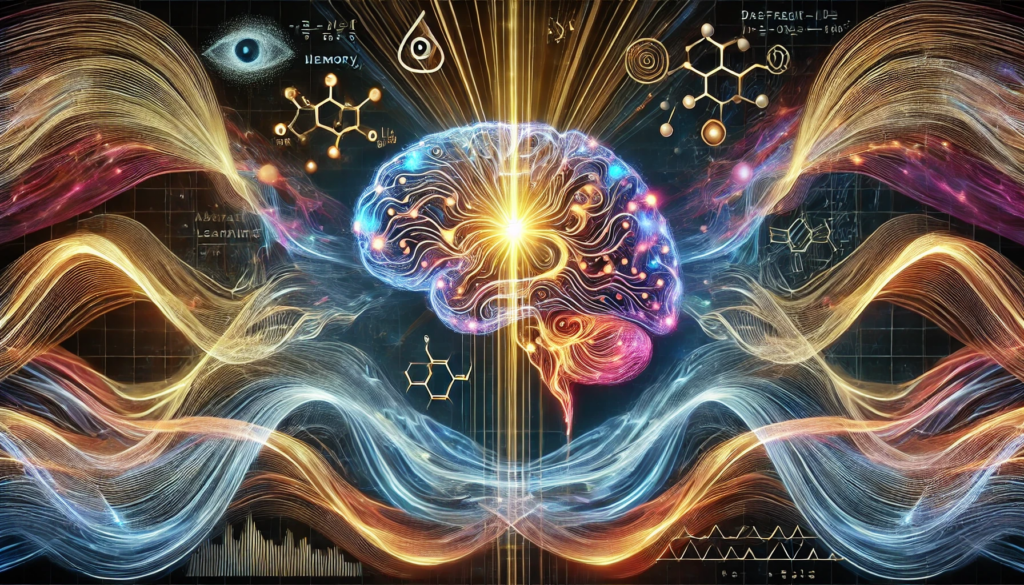
ChatGPT:
📘 Meta-learning, Social Cognition, and Consciousness in Brains and Machines
🌟 Introduction: Bridging AI and Neuroscience
The convergence of artificial intelligence (AI) and neuroscience has led to groundbreaking insights into learning, cognition, and consciousness. This article explores meta-learning, an advanced form of learning where systems improve their ability to learn tasks by leveraging prior experiences.
🔍 Key Focus Areas:
1. Meta-learning as a mechanism for faster learning in both AI and brains.
2. Model-based reinforcement learning (RL) and its ability to guide behavior based on internal models.
3. Insights into prefrontal cortex functions in learning and decision-making.
4. Connections between social cognition and meta-learning, emphasizing shared computational mechanisms.
5. Hypotheses about consciousness as a platform enabling meta-learning and general intelligence.
🧠 1. Model-Based Reinforcement Learning: The Foundation of Meta-Learning
What is Reinforcement Learning (RL)?
Reinforcement learning involves learning optimal behavioral policies through trial-and-error interactions with an environment. Algorithms optimize actions to maximize rewards or minimize penalties.
Model-Free vs. Model-Based RL:
• Model-free RL stores learned values from past experiences, focusing on immediate outcomes.
• Model-based RL builds internal models of tasks, enabling predictions and adaptability to new situations.
Biological Inspiration:
Dopamine neurons in the brain encode reward prediction errors (RPEs), signaling discrepancies between expected and actual outcomes, crucial for learning and decision-making.
Advantages of Model-Based RL in Meta-Learning:
• Knowledge Transfer: Past learning can accelerate performance in related tasks.
• Internal Simulation: Predictions about future states allow flexible adaptation to novel environments.
• Structured Knowledge: Models capture hidden variables such as time, reward identity, and effort requirements.
Neuroscientific Findings:
Experiments reveal that dopamine responses reflect model-based computations, including:
1. Predictions about reward timing and type.
2. Adjustments based on unexpected changes in task structures.
3. The use of latent state representations to infer task dynamics.
🤖 2. Meta-Learning in Brains and Machines
Deep Reinforcement Learning (Deep RL):
Deep RL combines deep neural networks with RL algorithms, enabling machines to learn complex tasks like video games and robotic control. However, these systems often struggle with sample efficiency, requiring massive data for training.
Biological Efficiency:
Humans and animals learn faster because they:
1. Transfer knowledge from previous tasks.
2. Utilize meta-learning to extract patterns across tasks.
Emergent Meta-Learning:
AI systems can spontaneously develop meta-learning abilities if:
1. They have short-term memory (like working memory in humans).
2. They are trained on sequences of related tasks.
For example, recurrent neural networks trained on decision-making tasks develop internal strategies resembling prefrontal cortex functions.
Key Neural Mechanisms:
• Prefrontal Cortex (PFC) supports memory-based learning.
• Dopamine-driven reinforcement adjusts neural connections to optimize learning.
• Episodic memory systems recall prior solutions, aiding rapid adaptation.
🤝 3. Social Cognition and Meta-Learning: Shared Mechanisms
Defining Social Cognition:
Social cognition involves understanding and predicting others’ behavior by modeling their thoughts and actions. It mirrors meta-learning processes, which involve:
1. Simulating others’ decisions to predict outcomes.
2. Adjusting strategies based on observed errors.
Experimental Evidence:
Studies show:
• Simulated Prediction Errors (sRPE) track others’ rewards.
• Simulated Action Errors (sAPE) adjust expectations based on others’ actions.
Neural Correlates:
• Ventromedial Prefrontal Cortex (vmPFC) represents both self-reward and others’ decisions.
• Temporoparietal Junction (rTPJ) processes social values.
• Anterior Insula (rAI) integrates social and self-related information.
Implications for AI:
AI systems can integrate meta-cognition and social reasoning to improve decision-making in group settings and multi-agent tasks.
🔮 4. Consciousness and General Intelligence: A Platform for Learning
Consciousness as a Meta-Learning Platform:
Consciousness may enable meta-learning by:
1. Supporting internal simulations for planning and prediction.
2. Allowing flexible recombination of learned models to solve new problems.
Global Workspace Theory (GWT):
• Consciousness acts as a shared workspace, integrating information from specialized neural modules.
• This flexibility mirrors AI architectures where neural networks collaborate through shared representations.
Evidence from Neuroscience:
• Trace Conditioning: Consciousness is required for tasks that involve retaining information across time gaps.
• Visual Perception Studies: Conscious awareness is critical for processing delayed information.
AI Implications:
AI systems inspired by GWT can combine pre-trained neural networks to solve complex problems, enhancing adaptability.
🚀 5. Future Directions: AI and Neuroscience Integration
1. Improving Learning Efficiency:
AI should adopt human-like learning mechanisms by:
• Building causal models for explanation and prediction.
• Leveraging intuitive physics and psychology for richer understanding.
• Harnessing compositional learning to transfer knowledge across tasks.
2. Enhancing Autonomy:
Inspired by neuroscience, AI systems could:
• Develop intrinsic motivations (curiosity, empowerment).
• Generate self-driven goals, enabling continuous adaptation.
3. Exploring Consciousness Mechanisms:
Further research is needed to:
• Model the role of working memory and short-term memory in AI learning.
• Implement Global Workspace architectures for modular and adaptive processing.
📌 Key Takeaways
1. Meta-learning accelerates learning by using prior experience, enabling fast adaptation to new tasks.
2. Model-based RL mirrors biological strategies for learning through internal models and predictions.
3. Prefrontal cortex integrates memory, rewards, and decisions, acting as a neural hub for meta-learning.
4. Social cognition parallels meta-learning, enabling predictions based on others’ behavior and decisions.
5. Dopamine signals in the brain provide error-based learning updates, supporting adaptive behavior.
6. Consciousness functions as a simulation tool, maintaining information over time and combining learned models flexibly.
7. Global Workspace Theory describes consciousness as an integration platform for complex tasks, inspiring AI architectures.
8. AI systems struggle with data efficiency, but meta-learning approaches can improve adaptability.
9. Intrinsic motivation and curiosity-driven learning can empower AI to operate autonomously.
10. Future research should focus on combining neuroscience insights with AI architectures to enhance general intelligence.
🌍 Conclusion
This extended review highlights the deep connections between neuroscience and AI, particularly through meta-learning mechanisms. By examining shared principles, such as model-based RL and internal simulations, researchers aim to build more adaptable and autonomous AI systems. Insights into consciousness as a flexible, integrative platform further illuminate the design of future AI systems capable of human-like reasoning and learning. Continued interdisciplinary exploration holds promise for solving fundamental questions about intelligence, cognition, and consciousness.
Q&A – Further Expanded FAQs
Q: What is meta-learning, and why is it critical for intelligence?
A: Meta-learning, or “learning to learn,” allows systems to leverage prior experiences to improve learning efficiency. It’s essential for general intelligence as it enables AI and biological systems to quickly adapt to new tasks without starting from zero. Unlike traditional learning methods, which rely on repeated training for each task, meta-learning transfers structured knowledge, accelerating adaptation and fostering multi-task problem-solving capabilities.
Q: How does reinforcement learning (RL) work, and what are its types?
A: RL is a learning framework where agents interact with an environment to maximize rewards through trial-and-error processes.
• Model-Free RL: Focuses on direct value updates based on experience without building an internal model. It’s simple but lacks adaptability.
• Model-Based RL: Constructs internal models of the environment to predict future states, enabling more flexible and data-efficient learning.
Biological systems often employ hybrid approaches, combining both methods to balance efficiency and adaptability.
Q: How do dopamine signals relate to reinforcement learning?
A: Dopamine neurons encode reward prediction errors (RPEs), signaling differences between expected and actual rewards. These signals update learning models, guiding behavior:
• Positive RPEs reinforce actions leading to unexpected rewards.
• Negative RPEs signal errors, prompting adjustments.
Such mechanisms mirror temporal-difference learning algorithms in AI, enabling systems to predict and adapt to outcomes based on feedback loops.
Q: Why is the prefrontal cortex (PFC) vital for meta-learning?
A: The PFC plays a central role in integrating memory, reward signals, and decision-making processes. It supports:
1. Working memory for short-term information retention.
2. Goal-directed actions based on predicted rewards.
3. Neural plasticity that allows learning to refine strategies over time.
In AI, the PFC’s function is mimicked by recurrent neural networks (RNNs), which retain information and adapt learning strategies dynamically.
Q: How does social cognition relate to meta-learning?
A: Social cognition involves modeling others’ intentions and actions to predict behaviors, similar to meta-learning’s focus on extracting patterns for task adaptation. Both rely on latent variable inference, where unseen factors are deduced from observable data.
Key examples:
• Simulated Reward Prediction Errors (sRPEs): Estimate others’ expected rewards.
• Simulated Action Prediction Errors (sAPEs): Adjust expectations based on observed behaviors.
AI systems incorporating social cognition can improve decision-making in multi-agent environments by learning cooperative and competitive strategies.
Q: How does consciousness enhance learning and intelligence?
A: Consciousness provides a simulation platform for exploring hypothetical scenarios and combining learned knowledge flexibly. It supports:
1. Internal modeling of the environment for predicting outcomes.
2. Flexible recombination of pre-trained neural networks to solve novel tasks.
3. Memory maintenance for retaining and utilizing past information.
In AI, these principles are applied using model-based RL, allowing systems to reason about tasks without direct experience, similar to how humans plan and strategize.
Q: What is the Global Workspace Theory (GWT), and how does it model consciousness?
A: GWT posits that consciousness acts as a shared workspace, integrating information across specialized brain modules. It supports:
• Broadcasting information for collaborative processing.
• Flexible task-switching by combining outputs from multiple modules.
AI implementations mirror this by combining pre-trained neural networks through shared latent spaces, enabling dynamic information processing and generalization to new tasks.
Q: Why do AI systems struggle with learning efficiency compared to humans?
A: Humans excel at learning due to:
1. Knowledge transfer from prior experiences.
2. Causal reasoning based on intuitive models of the world.
3. Compositional learning, combining learned elements to solve new problems.
AI systems, in contrast, often lack these features and require large datasets to achieve comparable performance. Meta-learning methods aim to address these gaps by enabling faster generalization.
Q: What is intrinsic motivation, and how can it make AI systems autonomous?
A: Intrinsic motivation refers to actions driven by curiosity or exploration, rather than external rewards. Humans often explore environments to learn new patterns without immediate benefits. AI systems can simulate this through:
• Curiosity-driven algorithms, which reward exploration of uncertain or novel situations.
• Empowerment models, where agents maximize control over outcomes.
These approaches promote self-directed learning and enable AI systems to develop autonomy, adapting continuously to dynamic environments.
Q: What are the limitations of meta-learning in AI and neuroscience?
A: Despite progress, meta-learning faces challenges such as:
1. Task complexity: AI struggles with abstract, high-level reasoning, like human problem-solving in natural settings.
2. Memory integration: Current models lack robust episodic memory systems for storing and retrieving past experiences.
3. Behavioral biases: Both humans and AI can develop suboptimal strategies due to cognitive shortcuts or incomplete models.
Future research aims to enhance memory architectures, modular learning frameworks, and adaptive exploration techniques.
Q: How can neuroscience inspire AI advancements?
A: Neuroscience offers insights into:
• Memory systems: Enhancing AI’s working and episodic memory architectures.
• Dopamine-inspired learning: Refining reinforcement algorithms for reward-based learning.
• Neural plasticity: Enabling dynamic adaptation through continuous learning.
AI can reciprocate by simulating brain functions to test hypotheses about human cognition, advancing our understanding of both fields.
Q: What are the future directions for AI and neuroscience integration?
A: Future research priorities include:
1. Data efficiency: Building AI systems that learn with fewer examples using causal modeling and meta-learning strategies.
2. Autonomy and motivation: Implementing algorithms that generate goals and rewards autonomously, inspired by curiosity-driven learning.
3. Consciousness-inspired AI: Exploring architectures based on Global Workspace Theory for modular and flexible decision-making.
4. Memory-driven learning: Designing systems with episodic and working memory for dynamic task switching.
5. Hybrid learning models: Combining model-free and model-based RL to balance simplicity and adaptability.
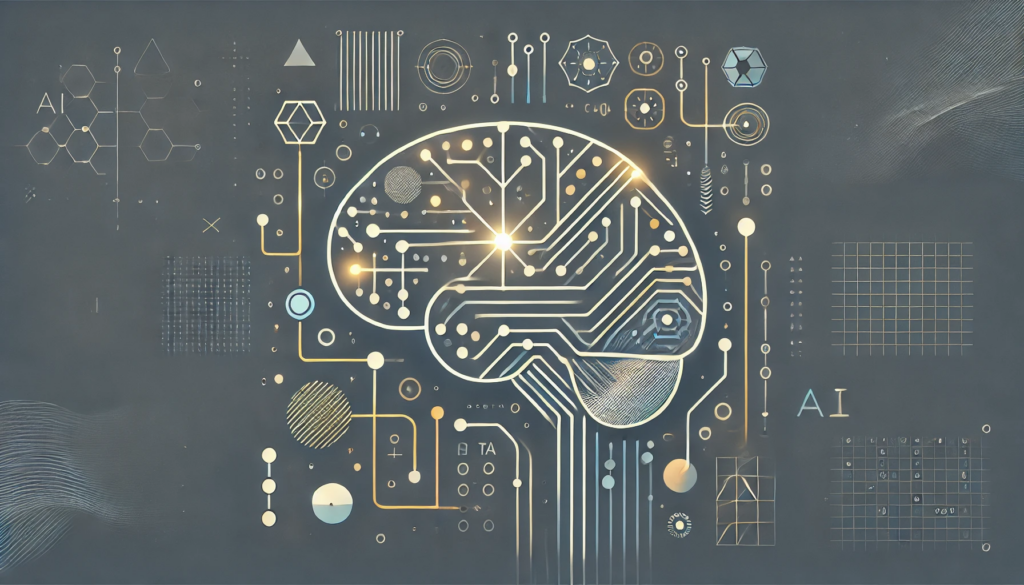
Consciousness in Artificial Intelligence: Insights from the Science of Consciousness
This report examines the potential for AI systems to achieve consciousness by evaluating them against established neuroscientific theories. It introduces a framework of “indicator properties” derived from these theories to assess AI consciousness.
Conclusion
The study concludes that, based on current neuroscientific theories, no existing AI systems exhibit consciousness. However, it suggests that there are no significant technical obstacles preventing the development of AI systems that could meet the criteria for consciousness in the future. The report emphasizes the importance of a rigorous, empirically grounded approach to evaluating AI consciousness, utilizing indicator properties derived from scientific theories.
Key Points
• Scientific Approach: The assessment of consciousness in AI is scientifically tractable, as consciousness can be studied scientifically, and findings from this research are applicable to AI.
• Indicator Properties: The report proposes a rubric for assessing consciousness in AI, consisting of a list of indicator properties derived from scientific theories.
• Current AI Limitations: Initial evidence indicates that while many indicator properties can be implemented in AI systems using current techniques, no existing system is a strong candidate for consciousness.
• Theoretical Frameworks: The report surveys several prominent scientific theories of consciousness, including recurrent processing theory, global workspace theory, higher-order theories, predictive processing, and attention schema theory.
• Future AI Development: The analysis suggests that there are no obvious technical barriers to building AI systems that satisfy the indicators of consciousness.
• Empirical Assessment: The report exemplifies a rigorous and empirically grounded approach to AI consciousness by assessing existing AI systems in detail, in light of well-supported neuroscientific theories.
• Consciousness Rubric: A proposed rubric for assessing AI consciousness includes indicator properties derived from scientific theories, providing a structured approach to evaluation.
• Implementation Evidence: While many indicator properties can be implemented in AI systems using current techniques, no current system appears to be a strong candidate for consciousness.
• Technical Feasibility: The report suggests that there are no obvious technical barriers to building AI systems that satisfy the indicators of consciousness, indicating potential for future development.
• Scientific Theories Surveyed: The report surveys several prominent scientific theories of consciousness, providing a comprehensive overview of the current understanding in the field.
Summary
1. Scientific Assessment of AI Consciousness: The report argues that evaluating consciousness in AI is scientifically feasible, as consciousness can be studied scientifically, and findings from this research are applicable to AI.
2. Rubric for AI Consciousness: A proposed rubric for assessing AI consciousness includes indicator properties derived from scientific theories, providing a structured approach to evaluation.
3. Current AI Systems and Consciousness: Initial evidence indicates that while many indicator properties can be implemented in AI systems using current techniques, no existing system is a strong candidate for consciousness.
4. Survey of Scientific Theories: The report surveys several prominent scientific theories of consciousness, including recurrent processing theory, global workspace theory, higher-order theories, predictive processing, and attention schema theory.
5. Future AI Development Potential: The analysis suggests that there are no obvious technical barriers to building AI systems that satisfy the indicators of consciousness, indicating potential for future development.
6. Empirical Approach to AI Consciousness: The report exemplifies a rigorous and empirically grounded approach to AI consciousness by assessing existing AI systems in detail, in light of well-supported neuroscientific theories.
7. Indicator Properties Implementation: While many indicator properties can be implemented in AI systems using current techniques, no current system appears to be a strong candidate for consciousness.
8. Technical Feasibility of Conscious AI: The report suggests that there are no obvious technical barriers to building AI systems that satisfy the indicators of consciousness, indicating potential for future development.
9. Comprehensive Survey of Theories: The report surveys several prominent scientific theories of consciousness, providing a comprehensive overview of the current understanding in the field.
10. No Current AI Consciousness: The study concludes that, based on current neuroscientific theories, no existing AI systems exhibit consciousness.
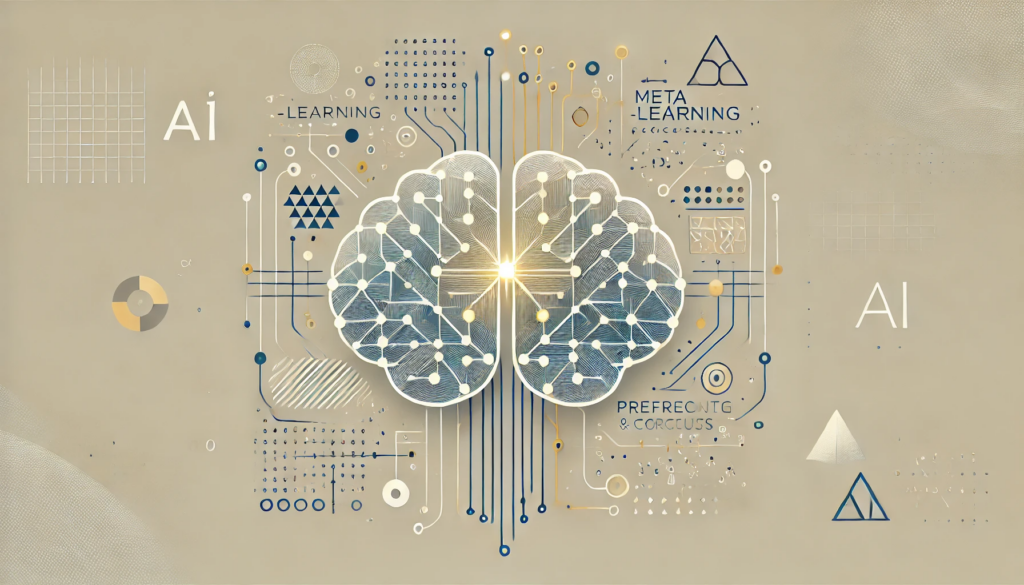