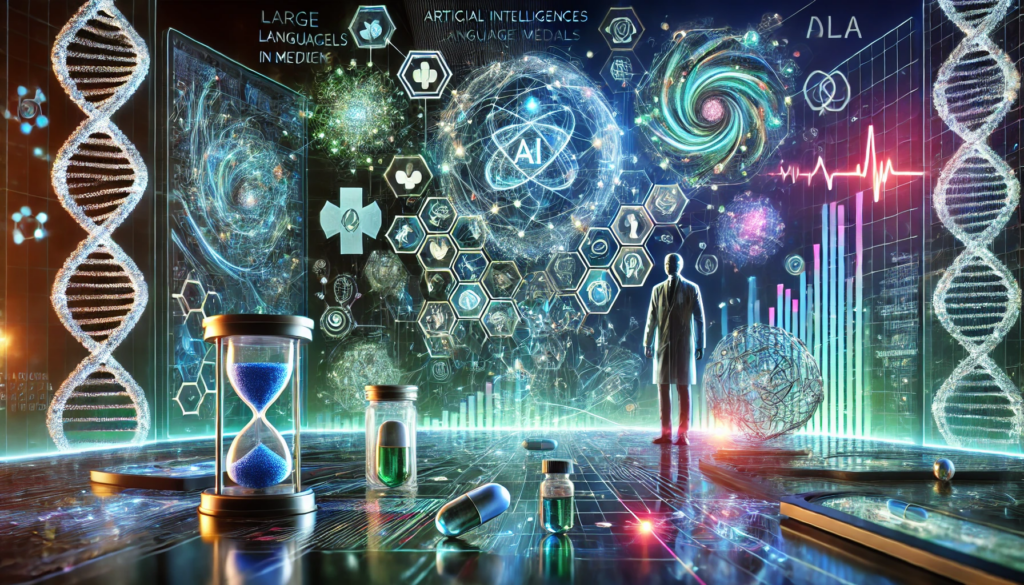
ChatGPT:
Large Language Models (LLMs) have emerged as transformative tools in the realm of healthcare, particularly in identifying potential treatments for rare diseases by repurposing existing medications. Traditionally, discovering therapies for rare diseases has been complex, costly, and slow, hindered significantly by limited commercial incentives and the extensive resources required for novel drug development. However, recent advancements in artificial intelligence, especially LLMs, are revolutionizing the landscape by swiftly identifying effective treatments among medications already approved for other conditions. This article explores in-depth the processes, advantages, successful case studies, challenges, and future implications of using LLMs to repurpose drugs for rare diseases.
Understanding Large Language Models (LLMs)
Large Language Models, like GPT-4, utilize deep learning techniques trained on vast textual data to understand and generate human-like language. In medicine, these models are applied to analyze massive volumes of biomedical literature, clinical studies, pharmaceutical data, and patient records. Their strength lies in the ability to discern nuanced connections, recognize complex patterns, and uncover relationships that human researchers might overlook due to data volume or subtlety.
How LLMs Facilitate Drug Repurposing
LLMs support drug repurposing through several critical functions:
• Data Integration and Knowledge Extraction: LLMs seamlessly integrate various sources of biomedical knowledge, including academic publications, clinical trial data, drug databases, and case reports, providing a comprehensive analytical foundation.
• Contextual Understanding: The ability to contextualize information helps LLMs identify meaningful connections across disparate diseases and drug treatments.
• Rapid Hypothesis Generation: Through rapid pattern recognition, LLMs can quickly generate hypotheses about potential drug-disease relationships, streamlining the exploration process significantly.
• Subtle Pattern Detection: LLMs uncover subtle indicators within literature, such as beneficial side effects or shared biological pathways, suggesting potential alternative uses for existing medications.
Case Study: Joseph Coates and POEMS Syndrome
Joseph Coates provides an illustrative example of the powerful capabilities of LLM-driven drug repurposing. Diagnosed with the rare and life-threatening POEMS syndrome, traditional treatment methods failed to improve his condition. Facing imminent hospice care, Coates’s medical team leveraged an LLM platform developed by Dr. David Fajgenbaum’s group at the University of Pennsylvania. The platform rapidly identified an unconventional combination of chemotherapy, immunotherapy, and steroids—previously untested for POEMS syndrome—as potentially effective. Remarkably, the treatment worked, allowing Coates to regain health sufficient for a stem-cell transplant, ultimately leading to remission. This dramatic success underscores the profound potential of LLM technologies to deliver life-saving solutions rapidly and effectively.
Detailed Advantages of LLMs in Drug Repurposing
The advantages of employing LLMs in drug repurposing include:
• Accelerated Discovery: LLMs significantly reduce the time required to identify effective treatments, from years or months down to weeks or days.
• Enhanced Efficiency and Cost-effectiveness: Utilizing existing, approved drugs reduces both the costs and risks associated with drug discovery, bypassing many regulatory and safety hurdles intrinsic to new drug development.
• Comprehensive Coverage: LLMs analyze large-scale data comprehensively, evaluating thousands of drug-disease combinations simultaneously.
• Optimized Resource Allocation: With predictive insights, resources can be effectively targeted toward promising drug repurposing opportunities, enhancing research productivity.
Additional Real-world Success Stories
Beyond Coates’s experience, numerous other successes demonstrate the practical application of LLM-driven drug repurposing:
• Castleman Disease: Dr. David Fajgenbaum himself successfully repurposed sirolimus, originally an immunosuppressant for transplant patients, to manage his own rare subtype of Castleman disease, demonstrating long-term efficacy.
• Chronic Nausea: Researchers at the University of Alabama at Birmingham identified inhaled isopropyl alcohol as an effective treatment for chronic nausea through an LLM-driven query, demonstrating immediate relief for the patient.
• Rare Neurological Disorders: An LLM model suggested guanfacine, traditionally used for hypertension, to significantly improve mobility in pediatric patients with neurological conditions.
Challenges in Utilizing LLMs for Drug Repurposing
Despite the promising successes, several challenges remain:
• Accuracy and Validation: Ensuring the accuracy and reliability of predictions is critical, as incorrect predictions could lead to harmful patient outcomes. Robust clinical validation and human oversight remain essential.
• Interpretability and Explainability: LLM outputs can sometimes be opaque, making it challenging to fully understand how certain conclusions are reached. Enhancing transparency and explainability is vital for clinical adoption and trust.
• Economic and Commercial Limitations: Most repurposed drugs are off-patent generics, resulting in limited financial incentives for pharmaceutical companies to invest in further research or clinical trials.
• Funding and Regulatory Challenges: Securing financial support for trials and navigating regulatory approval processes remain significant obstacles, despite the lower inherent risks compared to novel drug development.
Ethical and Social Implications
LLMs for drug repurposing hold significant ethical and social potential:
• Equitable Access: The technology democratizes access to medical advancements, potentially addressing healthcare inequalities by providing treatments to underserved patient populations, especially those with rare diseases.
• Patient Empowerment: Accelerated treatment discovery directly benefits patients, reducing suffering and improving quality of life.
• Resource Optimization: By effectively utilizing existing medications, healthcare systems can optimize resource use, delivering timely and affordable care.
Future Directions and Potential
Looking forward, several enhancements could maximize the potential of LLMs in drug repurposing:
• Improved AI Transparency: Advances in AI transparency and interpretability will facilitate broader acceptance and integration into clinical practice.
• Collaborative Networks: Establishing international collaborative networks for data sharing and clinical trial coordination could amplify LLM-driven discoveries globally.
• Policy and Incentives: Policy adjustments and targeted incentives could encourage pharmaceutical companies to engage more actively in repurposing efforts, leveraging AI-driven discoveries.
• Integration with Clinical Practice: Closer integration of LLM predictions into routine clinical practice, supported by robust validation frameworks, could enhance patient outcomes significantly.
Conclusion
Leveraging LLMs in drug repurposing represents a groundbreaking advancement with profound implications for treating rare diseases. These technologies efficiently mine existing biomedical knowledge, delivering rapid, cost-effective, and scalable treatment solutions. While challenges such as accuracy, transparency, and economic viability persist, the integration of LLMs into healthcare promises substantial, lasting benefits. By addressing these hurdles proactively, healthcare providers and researchers can unlock a future where patients suffering from rare diseases receive faster, more effective, and accessible treatments, revolutionizing rare disease management on a global scale.
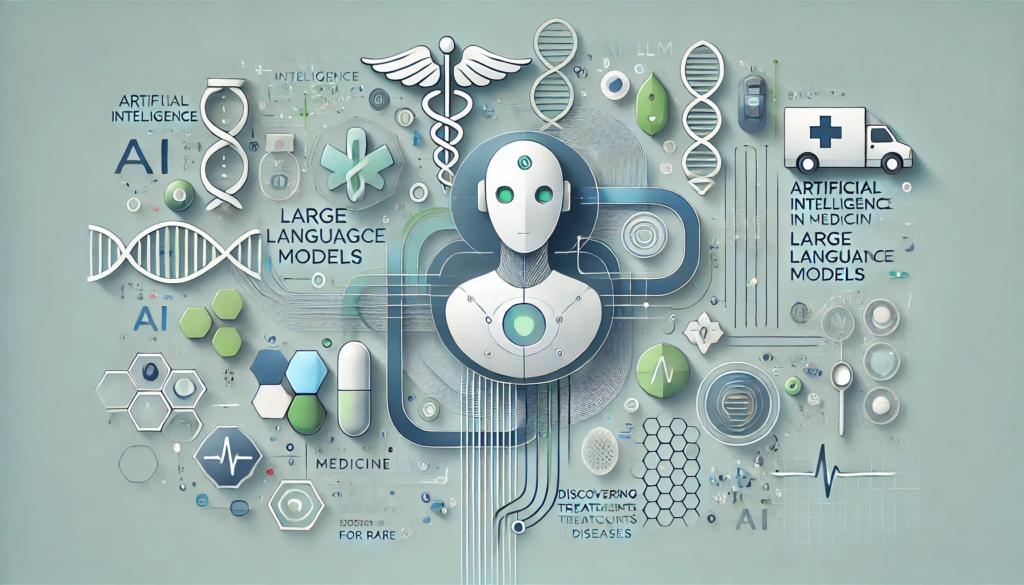