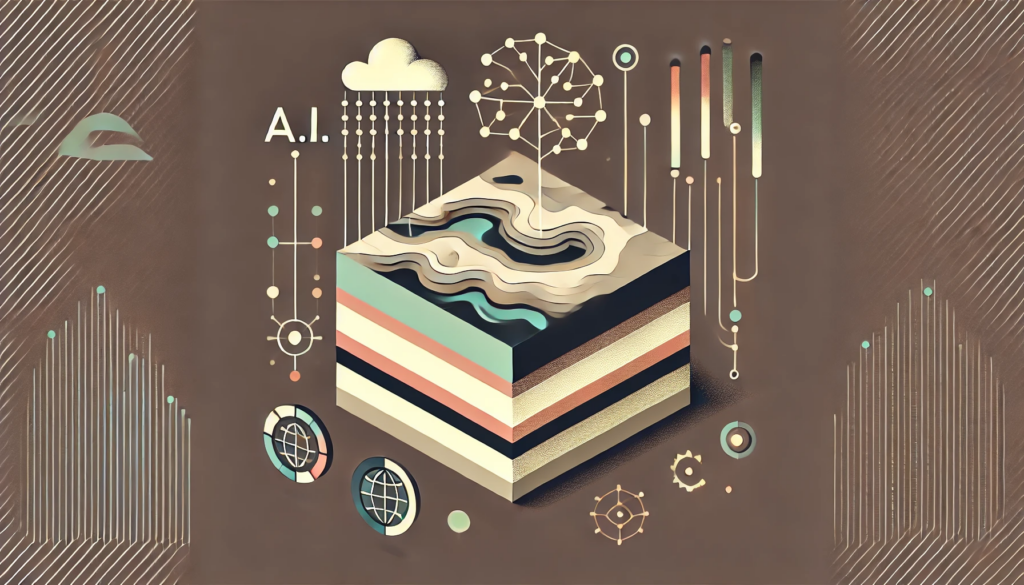
ChatGPT:
Fibre sensing in geophysics typically refers to the use of optical fibers as sensing elements to measure various geophysical parameters—such as strain, temperature, and seismic waves—along the length of the fiber cable. Instead of deploying discrete, point-based sensors at defined intervals, fibre-based sensing allows for continuous spatial measurements over potentially kilometers of terrain. In recent years, these techniques, often termed Distributed Fibre Optic Sensing (DFOS), have increasingly found application in areas like seismic monitoring, hydrocarbon exploration, earthquake hazard assessment, and CO₂ storage verification.
Key Principles:
1. Optical Fibers as Sensors:
Standard telecommunications-grade optical fibers, originally designed for data transmission, can also function as sensing elements. When pulses of laser light are sent down the fiber, minute backscatter signals occur due to interactions with the glass structure. These backscatter events—primarily Rayleigh, Brillouin, and Raman scatter—contain information about the local conditions along the fiber’s length.
2. Distributed Measurements:
Unlike traditional geophysical sensor arrays (e.g., geophones or thermistors), where each device measures data only at its own location, fibre sensing allows the entire length of the cable to serve as a continuous sensor. By analyzing the returning scatter signals, the system can produce a spatially continuous measurement of strain, temperature, or vibration at every point along the fiber—effectively thousands of sensors in one line.
3. Data Types from Fibre Sensing:
• Distributed Acoustic Sensing (DAS): Uses the Rayleigh backscatter to detect tiny strains in the fiber caused by passing seismic or acoustic waves. This can turn a buried or deployed fiber optic cable into a dense array of “virtual seismometers.”
• Distributed Temperature Sensing (DTS): Relies on the ratio of Raman backscatter intensities to estimate temperature variations along the fiber. It is particularly useful in geothermal reservoir monitoring, pipeline integrity checks, and groundwater studies.
• Distributed Strain Sensing (DSS): Employs Brillouin or Rayleigh scattering to measure static or quasi-static strains in the fiber, helping monitor subsurface deformation, landslides, or structural health.
Applications in Geophysics:
1. Earthquake and Seismic Monitoring:
By deploying optical fibers in boreholes, along pipelines, or along buried cables, geophysicists can gather dense seismic data. DAS can record earthquake signals or even microseisms, providing high-resolution subsurface images. This dense coverage can improve earthquake early warning, reservoir characterization, and fault mapping.
2. Subsurface Imaging and Hydrocarbon Exploration:
Fibre sensing can replace or complement conventional seismic sensor arrays in hydrocarbon exploration. With DAS, for example, downhole fiber cables can collect continuous seismic profiles during vertical seismic profiling (VSP) operations or time-lapse (4D) seismic surveys. This reduces operational complexity and improves imaging resolution.
3. Geothermal and CO₂ Storage Monitoring:
DTS can monitor temperature fronts in geothermal reservoirs, helping operators optimize injection and production. Similarly, during CO₂ sequestration, fibre sensing can help detect subtle changes in reservoir conditions and ensure safe storage.
4. Environmental and Geotechnical Monitoring:
DSS can track deformation along slopes or embankments, enabling early warning of landslides or subsidence. Fibre-based temperature sensing can also detect groundwater flow changes or leaks in dams and levees.
Advantages and Challenges:
• Advantages:
• Extremely high spatial resolution: Continuous data along the entire cable length.
• Remote operation and durability: Optical fibers are chemically inert and can function under harsh conditions.
• Reduced cost and complexity: One cable can replace thousands of discrete sensors, streamlining data acquisition.
• Challenges:
• Data volume and interpretation: The continuous nature of data results in massive datasets requiring advanced signal processing and interpretation.
• Sensitivity and calibration: Achieving the required sensitivity, isolating the desired signal from noise, and calibrating measurements for quantitative interpretation remain ongoing areas of research.
• Installation logistics: Obtaining optimal coupling between the fiber and the subsurface can be challenging, and long-term reliability of buried cables needs careful consideration.
Future Directions:
Research is focused on improving the sensitivity and bandwidth of fibre-optic sensors, combining different scattering modalities to simultaneously measure temperature, strain, and acoustics, and integrating with machine learning for advanced pattern recognition. As fibre sensing technology matures, it promises to transform geophysical exploration and monitoring by providing richer, more accessible, and cost-effective subsurface data than traditional sensor arrays.
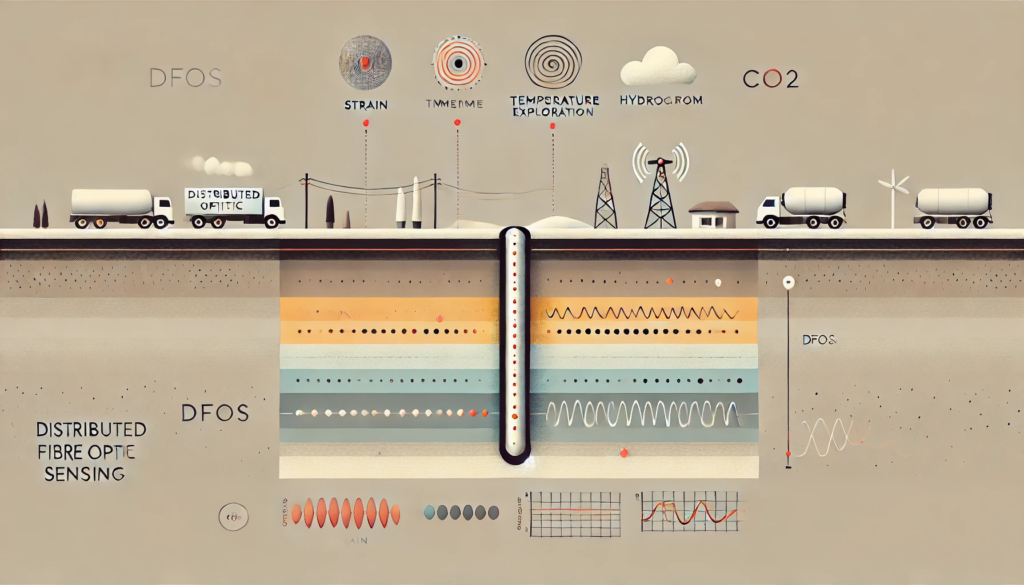
Artificial Intelligence (A.I.) holds substantial potential to advance geophysical research, transforming how scientists acquire, process, interpret, and model subsurface data. By leveraging machine learning (ML) and other advanced A.I. methodologies, geophysics can achieve more accurate, efficient, and predictive insights into Earth’s subsurface processes. Below are key areas and processes where A.I. can bring significant improvements.
1. Data Handling and Quality Control:
• Massive Data Integration:
Geophysical investigations often rely on combining multiple data types (seismic, gravity, magnetic, electrical, well-logs, and now fibre-optic sensing data) collected at varying spatial and temporal resolutions. Traditional workflows can struggle to integrate such large, heterogeneous datasets. A.I. methods, particularly deep learning, can help efficiently fuse and interpret these datasets, uncovering patterns and correlations that remain hidden in conventional approaches.
• Automated Noise Removal and Data Enhancement:
Geophysical data—such as seismic signals—are frequently contaminated by noise. A.I.-based denoising algorithms can learn complex noise patterns and remove them more effectively than traditional filters, resulting in cleaner datasets and more reliable subsequent interpretations. This is especially important for emerging technologies like distributed fibre optic sensing, where the continuous, high-frequency nature of data can introduce immense data volumes and new types of noise.
• Real-time Quality Assurance:
As sensors become more ubiquitous and continuous monitoring more common, A.I. systems can be deployed to identify faulty sensors, data dropouts, or unusual artifacts in real-time. By doing so, surveys can be dynamically adjusted, and costly field redeployments minimized.
2. Advanced Inversion and Imaging:
• Nonlinear Inversion Using Neural Networks:
Many geophysical problems require complex, nonlinear inversion—inferring subsurface properties from observed surface measurements. Traditional inversion techniques can be computationally expensive and sensitive to initial guesses or model assumptions. Neural networks, trained on synthetic or historical datasets, can provide rapid approximate solutions and help guide more traditional iterative inversions.
• Improved Tomographic Reconstructions:
Tomography, whether seismic or electromagnetic, involves reconstructing subsurface velocity or conductivity distributions. A.I. models can learn how to invert large data volumes and produce high-resolution images faster and more robustly than classic gradient-based methods. They may also incorporate uncertainty quantification, giving researchers insight into the reliability of the resulting models.
• Hybrid Approaches:
A new research direction combines physics-based modeling with data-driven A.I. approaches (so-called “physics-informed neural networks”). These methods embed known physical laws or partial differential equations into the architecture of the model, ensuring that the solutions are physically consistent as well as data-driven. This hybrid approach can accelerate and improve the inversion process.
3. Feature Extraction and Pattern Recognition:
• Seismic Facies Classification:
Manually interpreting seismic horizons, faults, and stratigraphic features is time-consuming and subjective. A.I. can automatically segment seismic volumes, identify subtle features, classify facies, and detect patterns that may correlate with geological structures or reservoirs. This leads to faster interpretations and more consistent results across teams.
• Event Detection in Continuous Monitoring:
In areas of earthquake seismology, volcanic monitoring, or induced seismicity (e.g., associated with geothermal projects or CO₂ storage), A.I. can sift through continuous data streams and rapidly detect seismic events, microseismicity, or unusual signals. Real-time detection and classification can inform hazard assessment and prompt rapid responses.
• Distributed Fibre Optic Sensing Analytics:
Fibre optic sensing generates massive, continuous datasets. Machine learning algorithms can identify characteristic waveforms (e.g., those from passing vehicles, seismic waves, or environmental noise) and classify them into meaningful categories. This can turn raw data into actionable information, like identifying zones of fluid movement, detecting pipeline leaks, or delineating faults.
4. Predictive Modeling and Forecasting:
• Reservoir and Resource Forecasting:
A.I. models can simulate the evolution of reservoir properties under various injection or production scenarios. By training ML models on historical data and physics-based simulations, geophysicists can predict future reservoir behavior, aiding in planning and optimizing production strategies.
• Earthquake and Volcanic Eruption Forecasting:
While predicting earthquakes or eruptions remains challenging, A.I. techniques can discern subtle precursors from large datasets of seismic and geodetic observations. Pattern-recognition models, anomaly detection algorithms, and probabilistic ML methods can increase the reliability of short-term forecasting or at least improve early warning systems.
5. Optimization of Survey Design and Experimentation:
• Optimal Sensor Placement:
A.I. algorithms can use simulation-based optimization techniques to determine the optimal sensor locations for seismic surveys, fibre-optic cable routes, or geoelectrical studies. This leads to better coverage, improved data quality, and cost efficiencies.
• Adaptive Experimentation:
As data is collected, an A.I. system can adaptively propose new measurements or survey designs to reduce uncertainties in the subsurface model. This active-learning approach ensures that each new measurement contributes the maximum possible information gain.
6. Accelerated Research Cycles:
• Automated Workflows and Expert Systems:
A.I. can encapsulate expert knowledge into machine learning models or rule-based systems. Such “virtual experts” can guide less experienced geophysicists through data processing steps, interpret certain standard geophysical signatures, and suggest next steps. This reduces human error and frees researchers to focus on more complex interpretations.
• Integration with Cloud and HPC:
Combining A.I. with High-Performance Computing (HPC) and cloud infrastructure makes it feasible to process petabyte-scale datasets. This synergy accelerates the entire research cycle—from raw data ingestion to final interpretation—enabling more frequent and timely insights.
Conclusion:
The integration of A.I. techniques into geophysics holds the promise of more accurate models, faster analyses, and comprehensive interpretations of the Earth’s subsurface. While challenges remain—such as ensuring robustness, interpretability, and trust in A.I.-derived models—ongoing research and cross-disciplinary collaboration are steadily overcoming these barriers. As a result, A.I. is poised to become a core component of the geophysicist’s toolkit, driving innovation and deeper understanding in the Earth sciences.
A.I. enhances data processing from fibre sensing systems through a combination of advanced pattern recognition, noise suppression, and automated analytics methods, ultimately transforming massive raw data streams into actionable geological insights. Key improvements include:
1. Noise Reduction and Signal Enhancement:
Fibre optic data, such as those from Distributed Acoustic Sensing (DAS) or Distributed Temperature Sensing (DTS), can be cluttered with various noise sources, including environmental interference or sensor imperfections. Machine learning models—particularly deep neural networks—are trained to distinguish signal from noise, resulting in cleaner, higher-quality datasets. This leads to more accurate interpretations and reduces the time and effort spent on manual filtering.
2. Feature Extraction and Event Detection:
High-frequency, continuous data from fibre optic cables can contain subtle, transient events such as microseisms, fluid flow signals, or small-scale strain changes. A.I. models excel at automatically identifying and classifying these events, discerning patterns or anomalies that traditional methods may overlook. For instance, algorithms can pinpoint seismic arrivals, detect pipeline leaks, or identify changes in subsurface stress conditions, improving both speed and reliability.
3. Scalable Data Integration and Management:
As fibre sensing deployments expand to cover longer distances and higher sample rates, data volumes become extremely large. A.I.-driven architectures handle these “big data” challenges efficiently, using cloud-based infrastructures and optimized data pipelines. They can integrate fibre-derived measurements with other geophysical datasets, enabling multi-parameter analyses and holistic subsurface models.
4. Real-time Processing and Early Warning:
Advanced A.I. algorithms can run in near-real-time, continuously streaming data from fibre optic sensors and instantly flagging significant events. For critical applications—such as monitoring stability in dams, embankments, or carbon storage sites—this immediate feedback can support early warning systems, improve operational safety, and guide rapid decision-making.
5. Predictive Analysis and Inversion Aid:
Beyond cleaning and interpreting data, A.I. models can serve as surrogate forward models for complex geophysical inversions. By rapidly approximating how subsurface changes affect fibre optic signals, these models accelerate the inversion process. This yields quicker updates to subsurface property estimates and predictions, effectively turning a passive measurement technique into a proactive forecasting tool.
In essence, integrating A.I. into fibre sensing workflows streamlines data handling, amplifies signal clarity, and automates interpretation processes. The result is a significant leap forward in the speed, accuracy, and comprehensiveness with which geophysicists can understand and respond to subsurface dynamics.
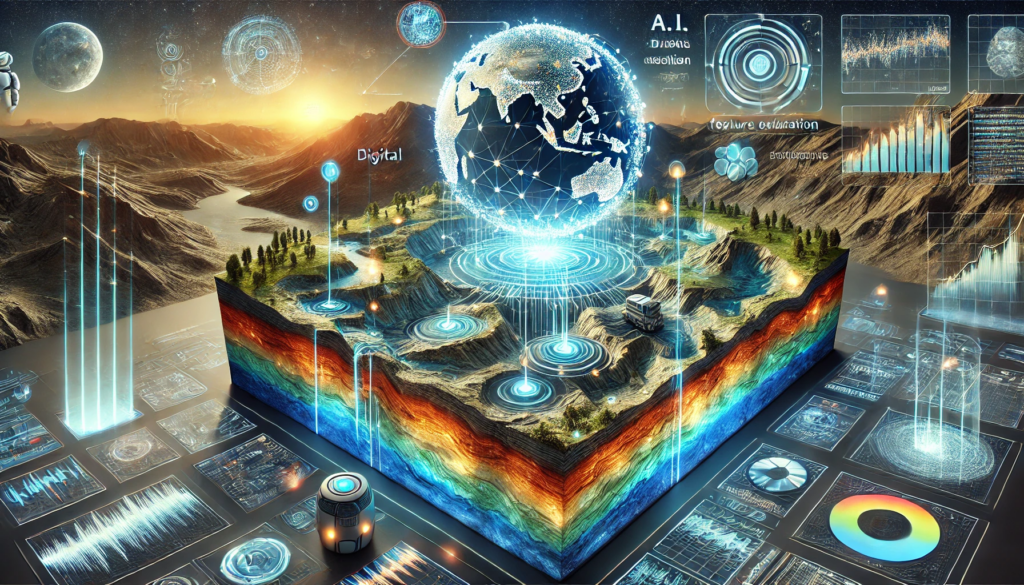