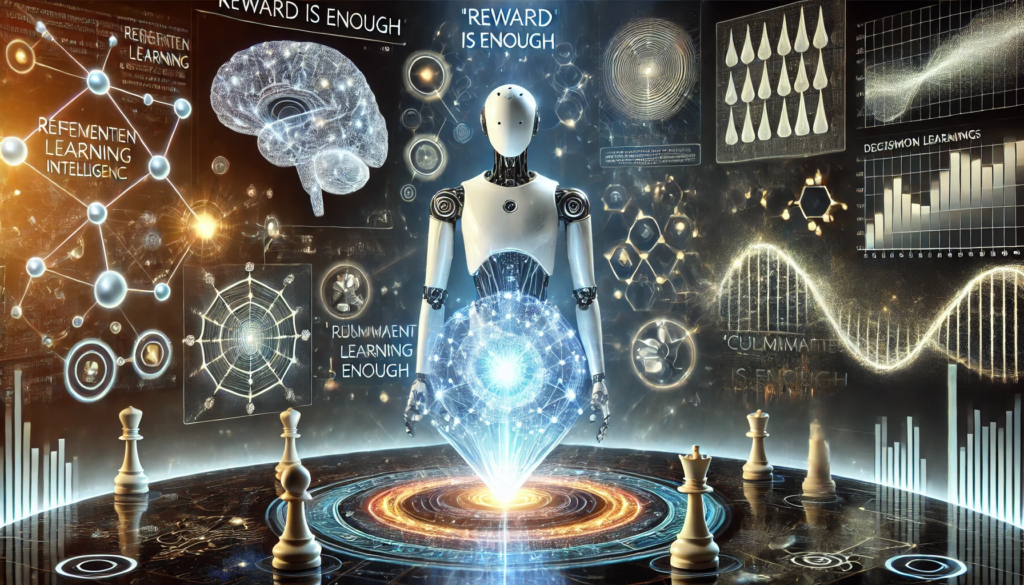
ChatGPT:
📚 Expanded Summary: Reward is Enough
🌟 Introduction
The paper “Reward is Enough” explores whether maximizing cumulative rewards can explain the emergence of intelligence and its associated abilities in natural and artificial systems. It challenges the traditional view that distinct goals drive different forms of intelligence—such as perception, language, and planning—and instead proposes that a singular objective, reward maximization, is sufficient.
The authors argue that intelligence emerges naturally as agents (e.g., animals or robots) adapt to complex environments to maximize rewards. For example, squirrels exhibit planning, memory, and perception while minimizing hunger, and robots optimize cleanliness using social and motor skills. Reinforcement learning, a trial-and-error-based process, is highlighted as the key mechanism for developing these abilities.
The paper delves into specific abilities, including knowledge acquisition, social intelligence, language, generalization, and imitation, showing how they emerge as tools for maximizing rewards. It also examines reinforcement learning frameworks and practical examples, such as AlphaZero’s mastery in games like Go and chess, to support the hypothesis.
🧠 Core Hypothesis
Reward-is-Enough Hypothesis:
• Intelligence and associated abilities emerge from maximizing cumulative rewards within complex environments.
• Agents develop sophisticated behaviors through reinforcement learning without requiring distinct goals for each skill.
🌍 Examples from Nature and Artificial Intelligence
🦴 Natural Intelligence
• Squirrels: Exhibit perception (to identify nuts), planning (to cache them), memory (to locate them later), and social intelligence (to bluff other squirrels) to minimize hunger.
• Animals: Dolphins, bats, and humans develop advanced behaviors like echolocation and tool use as adaptations to environmental demands.
🤖 Artificial Intelligence
• AlphaZero: Optimizes a simple goal—winning games—resulting in complex strategies and behaviors in chess and Go.
• Kitchen Robots: Achieve cleanliness goals through perception, motor control, and even social intelligence (encouraging less mess).
🛠️ Reinforcement Learning as a Framework
Agent-Environment Interaction:
• Agent: Receives observations, takes actions, and maximizes rewards based on interactions with the environment.
• Environment: Provides feedback through rewards and observations, guiding the agent’s adaptations.
Key Mechanism:
• Trial and Error: Agents learn by interacting with the environment, improving behaviors to increase cumulative rewards.
• Real-time Adaptation: Unlike static models, agents continually refine strategies in response to new experiences.
🔑 Abilities Derived from Rewards
1. Knowledge and Learning
• Knowledge can be innate (pre-programmed) or learned (acquired through experience).
• Example: A gazelle must innately recognize predators but learns specific evasion strategies based on experiences.
2. Perception
• Perceptual skills (e.g., image recognition, speech processing) are driven by the need to achieve goals like avoiding predators or finding food.
• Active perception, like echolocation in bats, emerges to maximize rewards efficiently.
3. Social Intelligence
• Agents interacting with others develop cooperation, bluffing, and negotiation to optimize group dynamics and maximize collective rewards.
• Game theory models, like Nash equilibria, explain cooperation and competition.
4. Language
• Language emerges as a tool for influencing environments and achieving goals (e.g., warning others of danger or negotiating resources).
• Beyond static models, language adapts dynamically through reinforcement learning to solve complex, context-dependent problems.
5. Generalization
• Agents must generalize past experiences to new challenges, ensuring adaptability in changing environments.
• Example: A fruit-eating animal adapts to different fruits or seasons by leveraging prior knowledge to maximize rewards.
6. Imitation
• Learning by observing others helps agents acquire complex skills quickly, reducing the cost of trial-and-error learning.
• Observational learning can extend beyond mimicking actions to understanding abstract concepts and strategies.
7. General Intelligence
• Defined as the ability to achieve a variety of goals in diverse contexts, general intelligence emerges from maximizing rewards across complex environments.
• AGI (Artificial General Intelligence) could arise by optimizing singular goals in rich, interactive worlds.
🌟 Case Studies and Real-World Evidence
AlphaZero:
• Focused solely on maximizing wins (+1 for victory, −1 for loss).
• Developed unexpected strategies like new openings and advanced endgame tactics.
• Demonstrated integration of multiple abilities (pattern recognition, planning, tactics) through reward-driven processes.
Atari Games and Robotics:
• Reinforcement learning agents mastering Atari games exhibited motor control, navigation, and object recognition.
• Robots optimized manipulation tasks, developing precise motor skills and object handling abilities.
Biological Systems:
• Evolutionary processes maximize survival and reproduction rewards, leading to complex animal behaviors and adaptation strategies.
🔍 Challenges and Critiques
1. Complexity of Reward Signals:
• Critics argue that defining the “right” reward signal is difficult.
• Authors counter that rich environments naturally produce demands for intelligence.
2. Sample Efficiency:
• Reinforcement learning requires extensive data and interactions, raising concerns about feasibility.
• Solutions involve improving algorithms and leveraging simulated environments.
3. Beyond Static Rewards:
• Critics claim fixed rewards may not capture all aspects of intelligence.
• The paper responds by emphasizing the adaptability of agents to varied and dynamic rewards.
4. Alternative Theories:
• Methods like supervised learning, unsupervised learning, and free-energy minimization are useful but lack the action-selection focus needed for intelligence.
🌎 Broader Implications
• Artificial General Intelligence (AGI):
• Reward-based learning provides a scalable approach to AGI by driving the emergence of diverse abilities in rich environments.
• Human-like Intelligence:
• Mimicking natural evolution through reward maximization could lead to machines that solve problems creatively and adapt dynamically.
• Ethical Concerns:
• Reward-focused agents require safeguards to align goals with human values and avoid harmful unintended consequences.
📝 Conclusion
The “Reward is Enough” hypothesis suggests that maximizing cumulative rewards can explain and replicate intelligence, including complex abilities like language, perception, and social interaction. Reinforcement learning serves as a practical framework to implement this idea, enabling agents to learn dynamically through interactions with their environments.
Practical evidence, such as AlphaZero’s performance and advances in robotics, supports this hypothesis, demonstrating that single-goal systems can develop integrated intelligence. While challenges remain, the paper argues that further advances in reinforcement learning could lead to artificial general intelligence, fundamentally shaped by reward-driven processes.
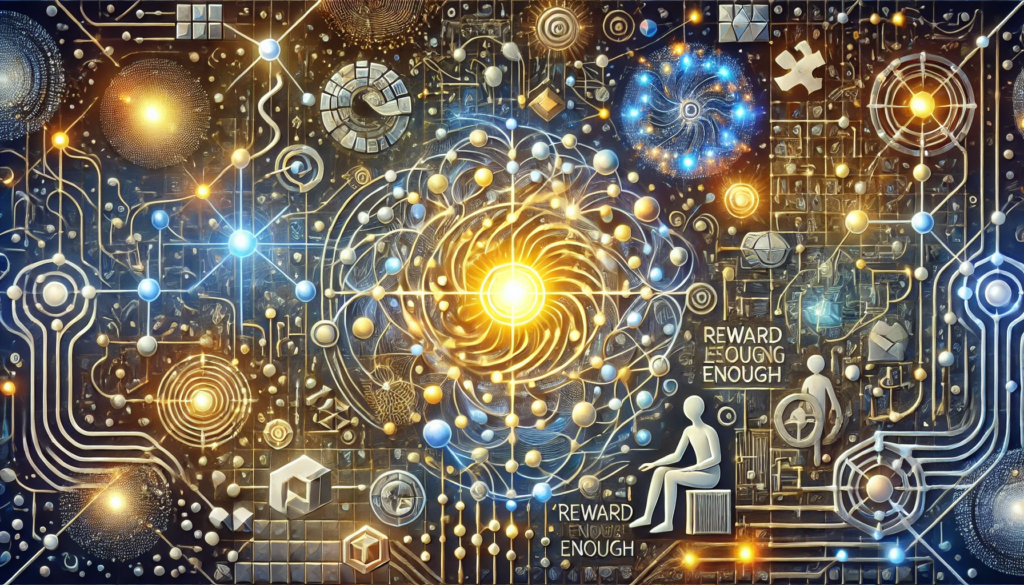
Q&A
Q: What is the main hypothesis of the paper “Reward is Enough”?
A: The hypothesis argues that maximizing cumulative rewards is sufficient to explain the emergence of intelligence and its abilities. Intelligence develops as a byproduct of pursuing goals defined by rewards within complex environments.
Q: How does the paper define intelligence?
A: Intelligence is described as the ability to achieve goals effectively within an environment. This includes flexible adaptation, planning, learning, and problem-solving skills driven by reward maximization.
Q: Why does the paper emphasize reinforcement learning?
A: Reinforcement learning provides a general framework for agents to learn and adapt through trial-and-error interactions with their environment. It simulates how intelligence emerges by continuously optimizing behaviors to maximize rewards.
Q: What are some examples supporting the hypothesis?
A: – Squirrels use perception, planning, and memory to maximize food collection.
• AlphaZero achieved mastery in Go and chess through reward-based learning, developing strategies without predefined rules.
• Robots optimize cleanliness goals, displaying motor control, perception, and even social skills.
Q: How does reward maximization lead to complex abilities like language and social intelligence?
A: Language and social skills arise as tools to optimize interactions and outcomes in dynamic environments. For example, agents may learn to negotiate, communicate warnings, or coordinate actions to achieve higher rewards.
Q: Can reinforcement learning produce general intelligence (AGI)?
A: The paper suggests that sufficiently complex environments could lead to AGI through reward maximization. Agents would develop a range of abilities needed to solve diverse problems and adapt to new situations.
Q: What are the main criticisms of the hypothesis?
A: Critics highlight challenges like:
• Designing the right reward signals.
• Sample inefficiency in learning processes.
• Addressing abilities that may not directly tie to specific rewards, such as creativity or abstract reasoning.
Q: How does the paper address criticisms about reward complexity?
A: The authors argue that rich environments naturally create demands for intelligence, making even simple reward signals sufficient to drive sophisticated abilities through adaptation.
Q: Is reward maximization better than other approaches to intelligence?
A: Unlike supervised learning or prediction-based models, reward maximization explicitly handles decision-making and action-selection problems. It offers a unified approach for integrating diverse abilities within a single system.
Q: What real-world applications demonstrate this hypothesis?
A: Examples include:
• AlphaZero developing advanced strategies in board games.
• Robotic systems learning motor control and navigation tasks.
• Atari agents mastering video games through reward-driven learning.
Q: How does this approach handle uncertainty and adaptation?
A: Reinforcement learning allows agents to learn and generalize from incomplete or uncertain information by continuously adjusting their strategies based on feedback.
Q: What role does imitation play in this framework?
A: Imitation accelerates learning by allowing agents to observe and replicate successful behaviors, reducing trial-and-error cycles while optimizing rewards.
Q: Does this hypothesis imply rewards must be explicitly defined?
A: Not necessarily. Rewards can emerge implicitly from interactions with the environment, enabling flexible learning without rigid programming.
Q: Could this approach work for human-like creativity?
A: The paper speculates that creativity could emerge as a means of maximizing rewards in novel scenarios, similar to how humans invent tools or strategies.
Q: How does this hypothesis inform AI safety and ethics?
A: Reward-driven agents require careful design to ensure goals align with human values, avoiding unintended harmful behaviors.
Q: What future research does this hypothesis inspire?
A: It encourages exploring how different environments and reward signals influence intelligence development and testing reinforcement learning for AGI in more complex, real-world scenarios.
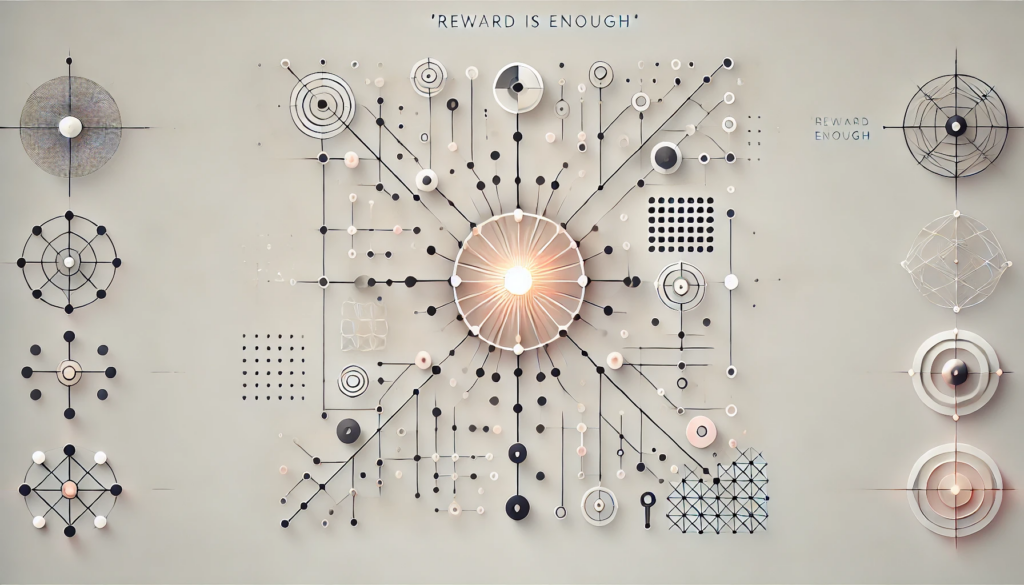